A Content Adaptive Learnable Time-Frequency Representation For Audio Signal Processing
arxiv(2023)
摘要
We propose a learnable content adaptive front end for audio signal processing. Before the modern advent of deep learning, we used fixed representation non-learnable front-ends like spectrogram or mel-spectrogram with/without neural architectures. With convolutional architectures supporting various applications such as ASR and acoustic scene understanding, a shift to a learnable front ends occurred in which both the type of basis functions and the weight were learned from scratch and optimized for the particular task of interest. With the shift to transformer-based architectures with no convolutional blocks present, a linear layer projects small waveform patches onto a small latent dimension before feeding them to a transformer architecture. In this work, we propose a way of computing a content-adaptive learnable time-frequency representation. We pass each audio signal through a bank of convolutional filters, each giving a fixed-dimensional vector. It is akin to learning a bank of finite impulse-response filterbanks and passing the input signal through the optimum filter bank depending on the content of the input signal. A content-adaptive learnable time-frequency representation may be more broadly applicable, beyond the experiments in this paper.
更多查看译文
关键词
filter-banks,content adaptive front ends
AI 理解论文
溯源树
样例
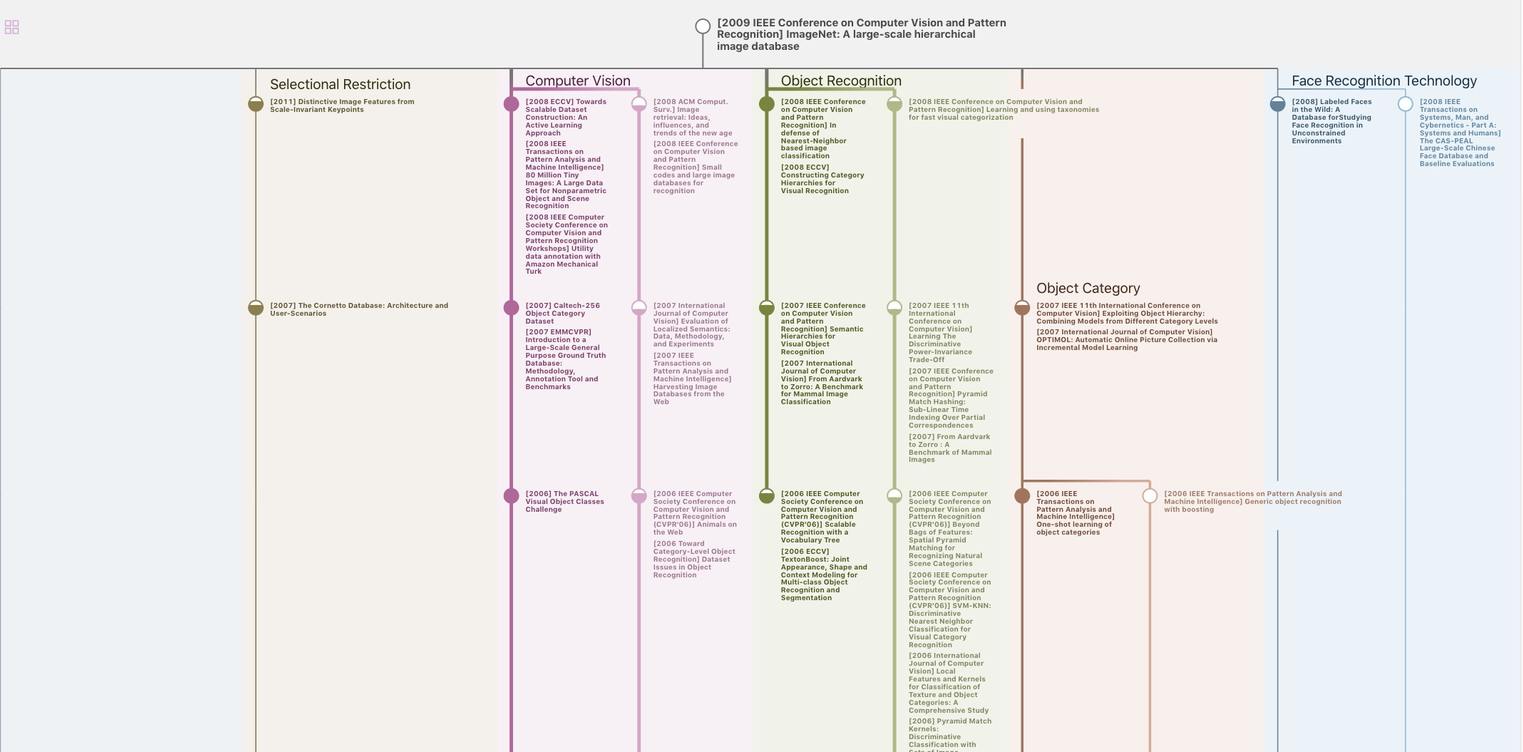
生成溯源树,研究论文发展脉络
Chat Paper
正在生成论文摘要