Online Linear Quadratic Tracking With Regret Guarantees
IEEE CONTROL SYSTEMS LETTERS(2023)
摘要
Online learning algorithms for dynamical systems provide finite time guarantees for control in the presence of sequentially revealed cost functions. We pose the classical linear quadratic tracking problem in the framework of online optimization where the time-varying reference state is unknown a priori and is revealed after the applied control input. We show the equivalence of this problem to the control of linear systems subject to adversarial disturbances and propose a novel online gradient descent-based algorithm to achieve efficient tracking in finite time. We provide a dynamic regret upper bound scaling linearly with the path length of the reference trajectory and a numerical example to corroborate the theoretical guarantees.
更多查看译文
关键词
Optimal tracking,online control
AI 理解论文
溯源树
样例
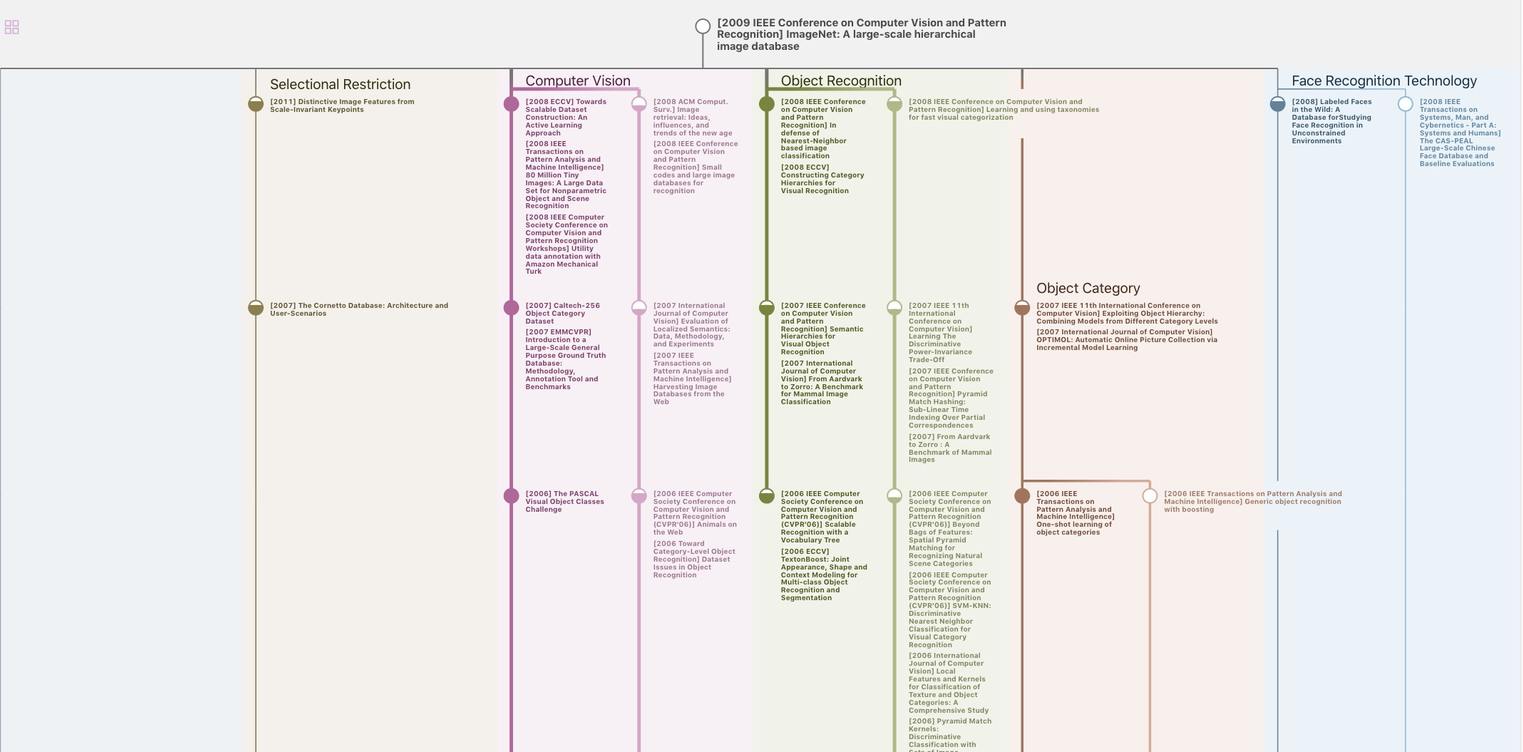
生成溯源树,研究论文发展脉络
Chat Paper
正在生成论文摘要