Fixed Design Analysis of Regularization-Based Continual Learning
arxiv(2023)
摘要
We consider a continual learning (CL) problem with two linear regression tasks in the fixed design setting, where the feature vectors are assumed fixed and the labels are assumed to be random variables. We consider an $\ell_2$-regularized CL algorithm, which computes an Ordinary Least Squares parameter to fit the first dataset, then computes another parameter that fits the second dataset under an $\ell_2$-regularization penalizing its deviation from the first parameter, and outputs the second parameter. For this algorithm, we provide tight bounds on the average risk over the two tasks. Our risk bounds reveal a provable trade-off between forgetting and intransigence of the $\ell_2$-regularized CL algorithm: with a large regularization parameter, the algorithm output forgets less information about the first task but is intransigent to extract new information from the second task; and vice versa. Our results suggest that catastrophic forgetting could happen for CL with dissimilar tasks (under a precise similarity measurement) and that a well-tuned $\ell_2$-regularization can partially mitigate this issue by introducing intransigence.
更多查看译文
关键词
continual learning,fixed design analysis,regularization-based
AI 理解论文
溯源树
样例
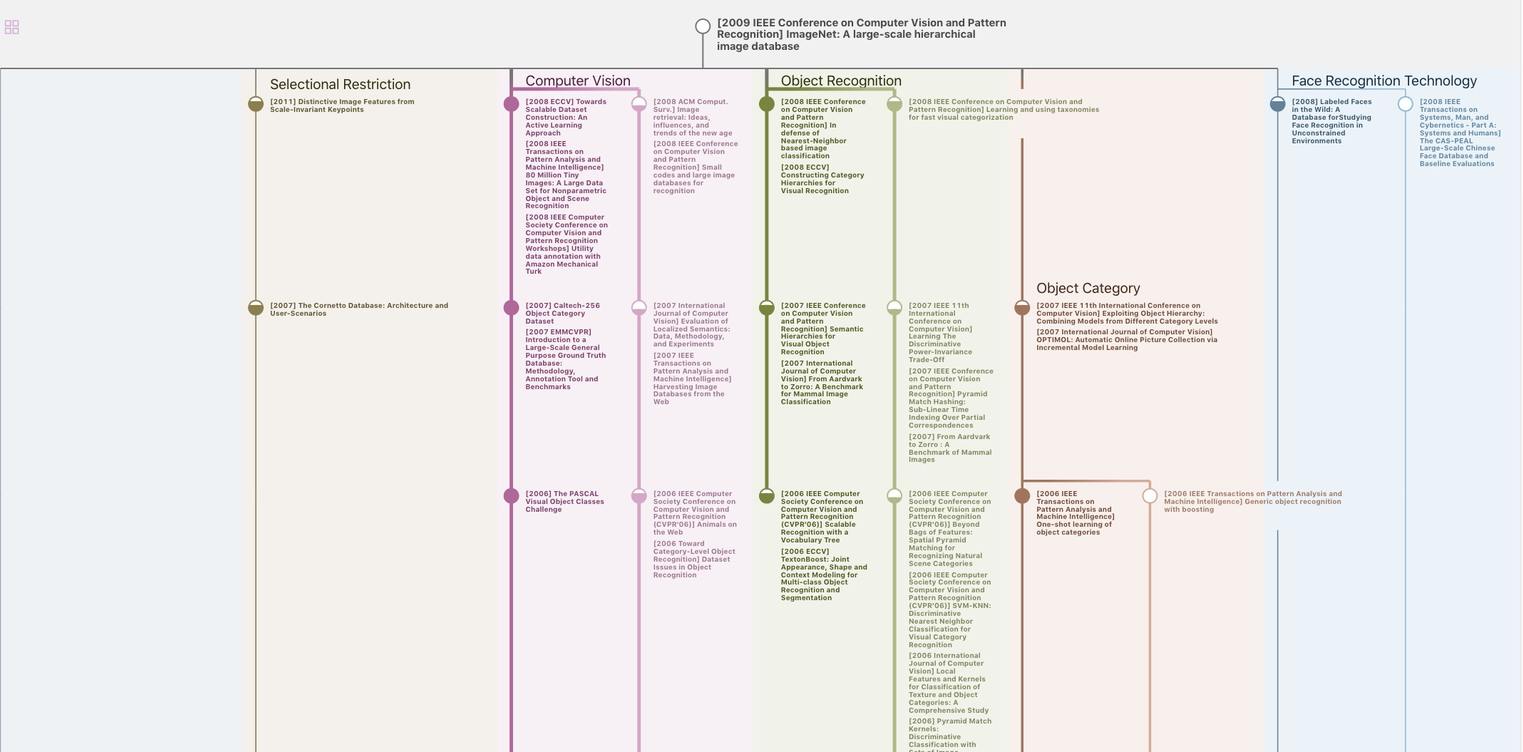
生成溯源树,研究论文发展脉络
Chat Paper
正在生成论文摘要