Efficient Neural Generation of 4K Masks for Homogeneous Diffusion Inpainting
arxiv(2023)
摘要
With well-selected data, homogeneous diffusion inpainting can reconstruct images from sparse data with high quality. While 4K colour images of size 3840 x 2160 can already be inpainted in real time, optimising the known data for applications like image compression remains challenging: Widely used stochastic strategies can take days for a single 4K image. Recently, a first neural approach for this so-called mask optimisation problem offered high speed and good quality for small images. It trains a mask generation network with the help of a neural inpainting surrogate. However, these mask networks can only output masks for the resolution and mask density they were trained for. We solve these problems and enable mask optimisation for high-resolution images through a neuroexplicit coarse-to-fine strategy. Additionally, we improve the training and interpretability of mask networks by including a numerical inpainting solver directly into the network. This allows to generate masks for 4K images in around 0.6 seconds while exceeding the quality of stochastic methods on practically relevant densities. Compared to popular existing approaches, this is an acceleration of up to four orders of magnitude.
更多查看译文
关键词
homogeneous diffusion,efficient neural generation
AI 理解论文
溯源树
样例
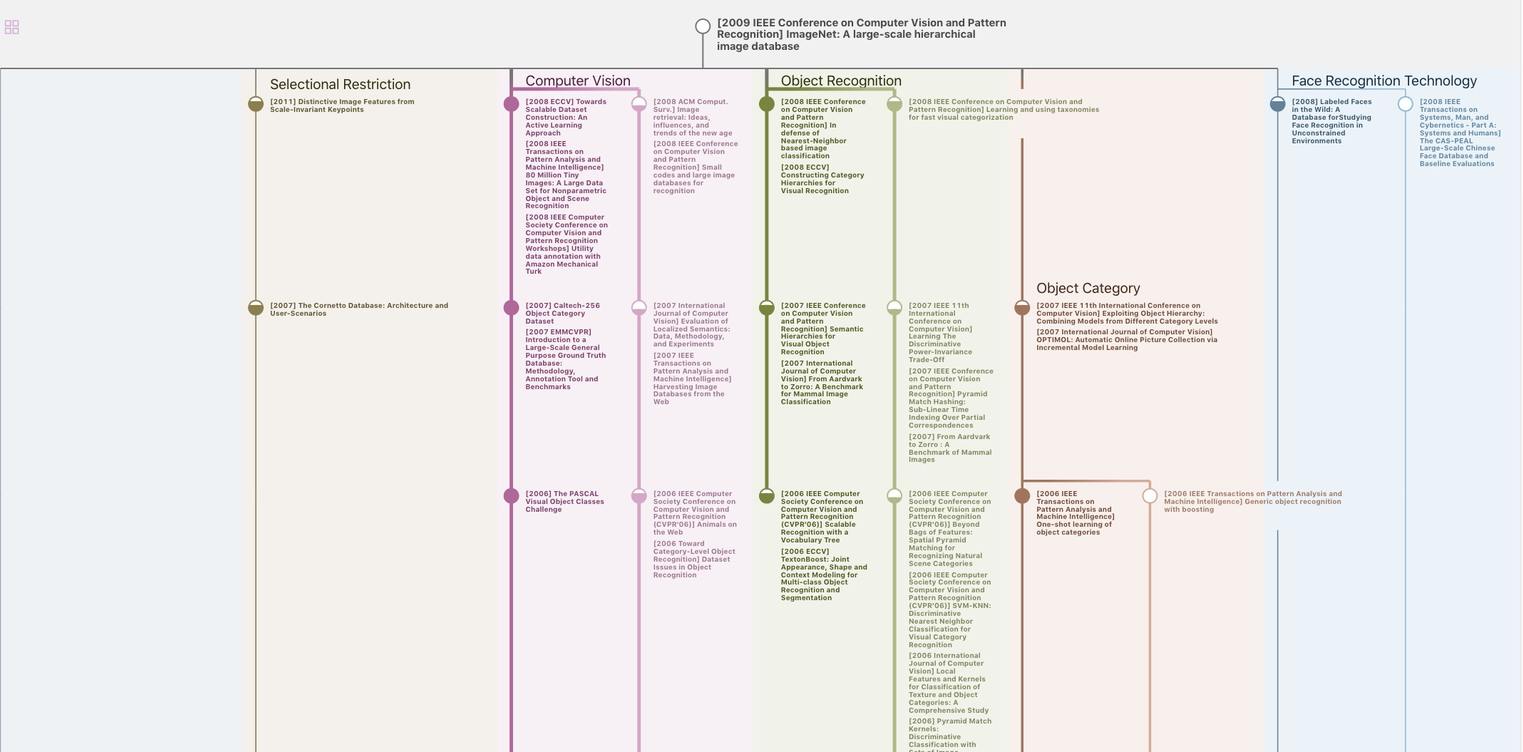
生成溯源树,研究论文发展脉络
Chat Paper
正在生成论文摘要