Single-view Neural Radiance Fields with Depth Teacher
arxiv(2023)
摘要
Neural Radiance Fields (NeRF) have been proposed for photorealistic novel view rendering. However, it requires many different views of one scene for training. Moreover, it has poor generalizations to new scenes and requires retraining or fine-tuning on each scene. In this paper, we develop a new NeRF model for novel view synthesis using only a single image as input. We propose to combine the (coarse) planar rendering and the (fine) volume rendering to achieve higher rendering quality and better generalizations. We also design a depth teacher net that predicts dense pseudo depth maps to supervise the joint rendering mechanism and boost the learning of consistent 3D geometry. We evaluate our method on three challenging datasets. It outperforms state-of-the-art single-view NeRFs by achieving 5$\sim$20\% improvements in PSNR and reducing 20$\sim$50\% of the errors in the depth rendering. It also shows excellent generalization abilities to unseen data without the need to fine-tune on each new scene.
更多查看译文
关键词
neural radiance fields,depth,teacher,single-view
AI 理解论文
溯源树
样例
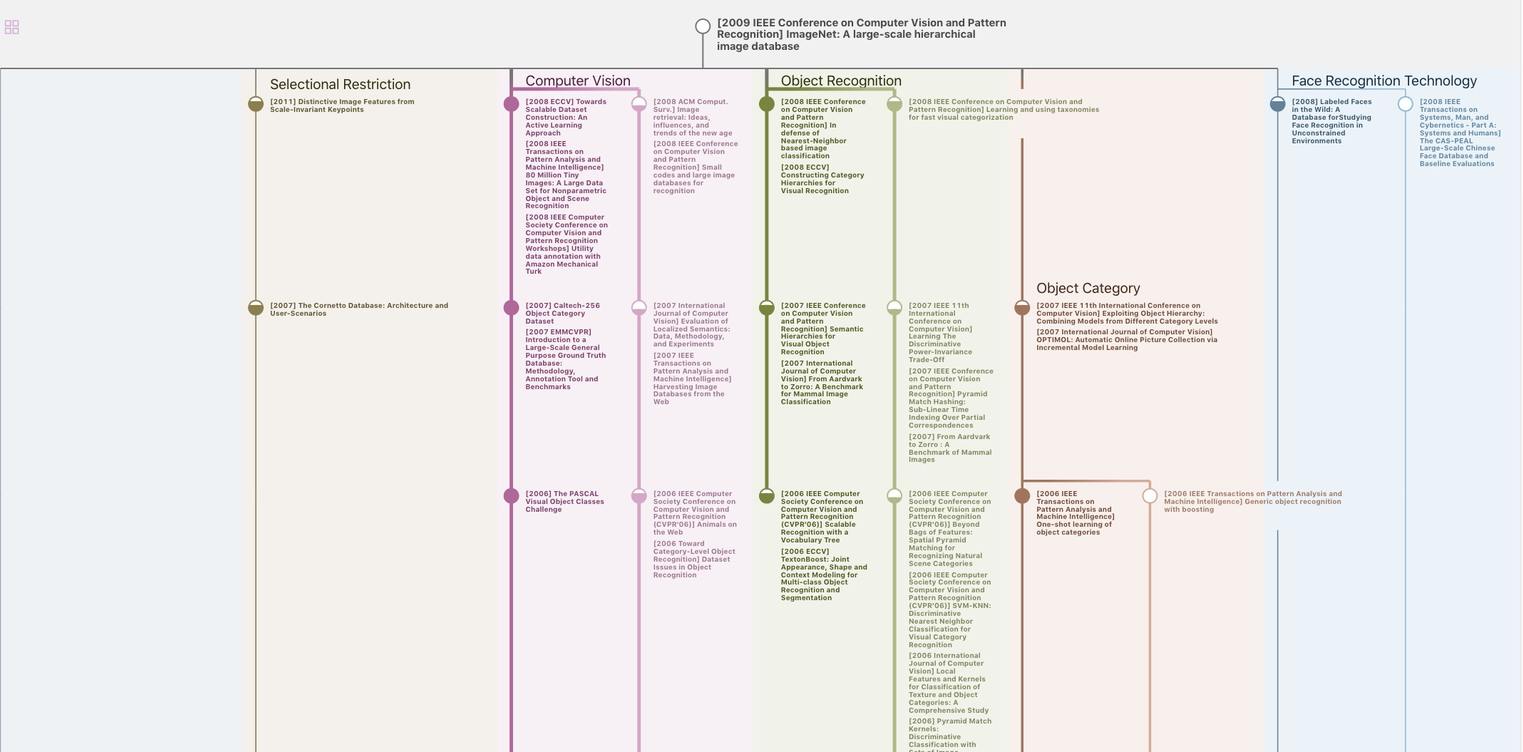
生成溯源树,研究论文发展脉络
Chat Paper
正在生成论文摘要