Efficient Learning of High Level Plans from Play
arxiv(2023)
摘要
Real-world robotic manipulation tasks remain an elusive challenge, since they involve both fine-grained environment interaction, as well as the ability to plan for long-horizon goals. Although deep reinforcement learning (RL) methods have shown encouraging results when planning end-to-end in high-dimensional environments, they remain fundamentally limited by poor sample efficiency due to inefficient exploration, and by the complexity of credit assignment over long horizons. In this work, we present Efficient Learning of High-Level Plans from Play (ELF-P), a framework for robotic learning that bridges motion planning and deep RL to achieve long-horizon complex manipulation tasks. We leverage task-agnostic play data to learn a discrete behavioral prior over object-centric primitives, modeling their feasibility given the current context. We then design a high-level goal-conditioned policy which (1) uses primitives as building blocks to scaffold complex long-horizon tasks and (2) leverages the behavioral prior to accelerate learning. We demonstrate that ELF-P has significantly better sample efficiency than relevant baselines over multiple realistic manipulation tasks and learns policies that can be easily transferred to physical hardware.
更多查看译文
关键词
bridges motion planning,deep reinforcement learning methods,deep RL,elusive challenge,fine-grained environment interaction,High Level Plans,high-dimensional environments,high-level goal-conditioned policy,High-Level Plans,leverage task-agnostic play data,long horizons,long-horizon complex manipulation tasks,long-horizon goals,long-horizon tasks,multiple realistic manipulation tasks,poor sample efficiency,real-world robotic manipulation tasks,robotic learning
AI 理解论文
溯源树
样例
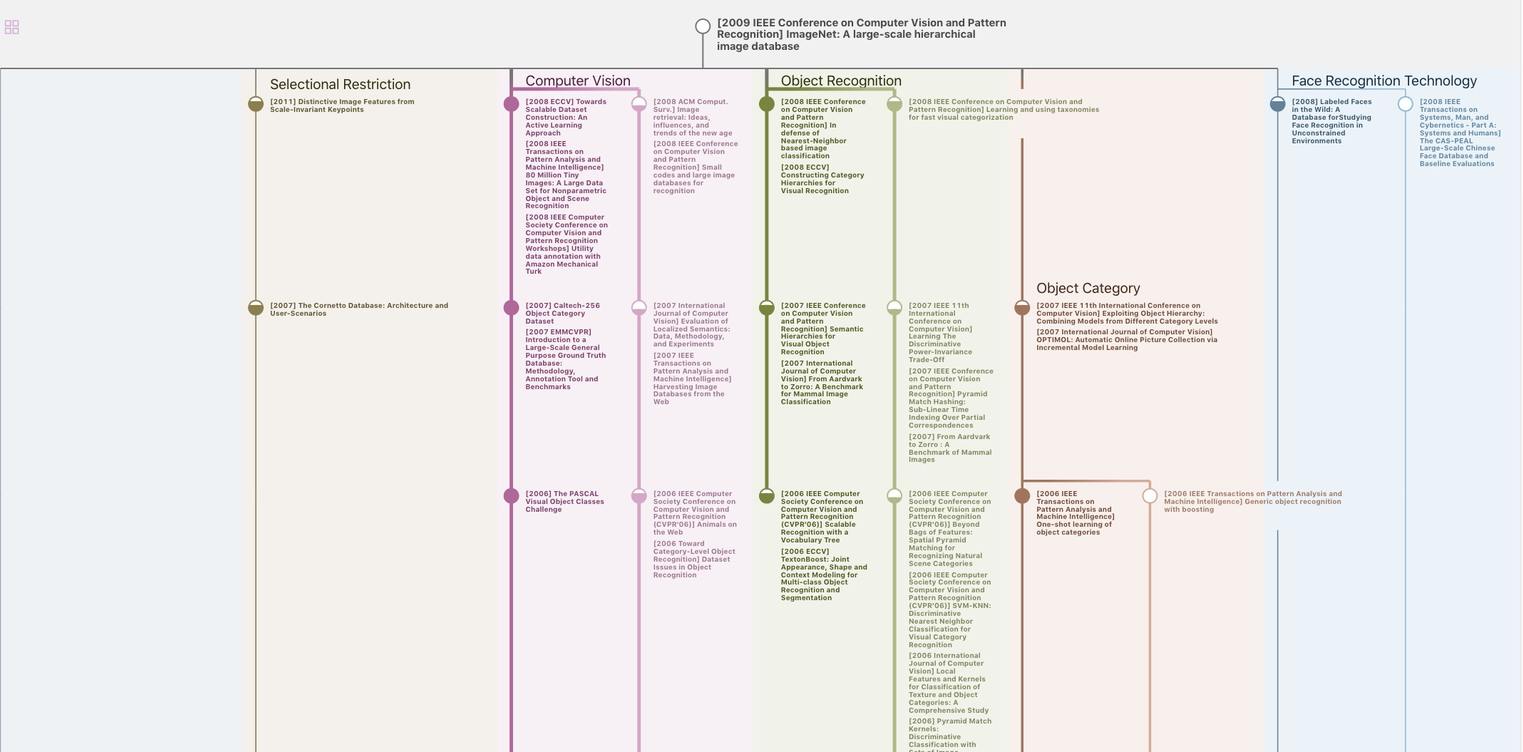
生成溯源树,研究论文发展脉络
Chat Paper
正在生成论文摘要