Fast suction-grasp-difficulty estimation for high throughput plastic-waste sorting
Journal of Mechanical Science and Technology(2023)
摘要
The selection of the grasping location is the most important task for robots that handle randomly shaped objects. In previous studies, the grasp quality was accurately evaluated, but the speed was much too low for high-throughput applications, and the focus was mainly on industrial products. In this study, a large-scale dataset for randomly deformed plastics is constructed. We propose a contact-area estimation model and difficulty function for a quantitative analysis of surface conditions. Synthetic labels were calculated using the tuned difficulty function for donut-shaped contact areas. We trained the network containing a pre-trained encoder and decoder with skip connections for grasp-difficulty map estimation. Grasp-difficulty estimations for multiple objects required at most 30.9 ms with an average error rate of 1.65 %. The algorithm had a 94.4 % grasping success rate and its computational efficiency was compared with that in previous studies. The algorithm enables the rapid sorting of continuously conveyed objects with higher throughput.
更多查看译文
关键词
Convolutional neural network, Factory automation, Rapid manipulation, Robot vision, Suction grasping
AI 理解论文
溯源树
样例
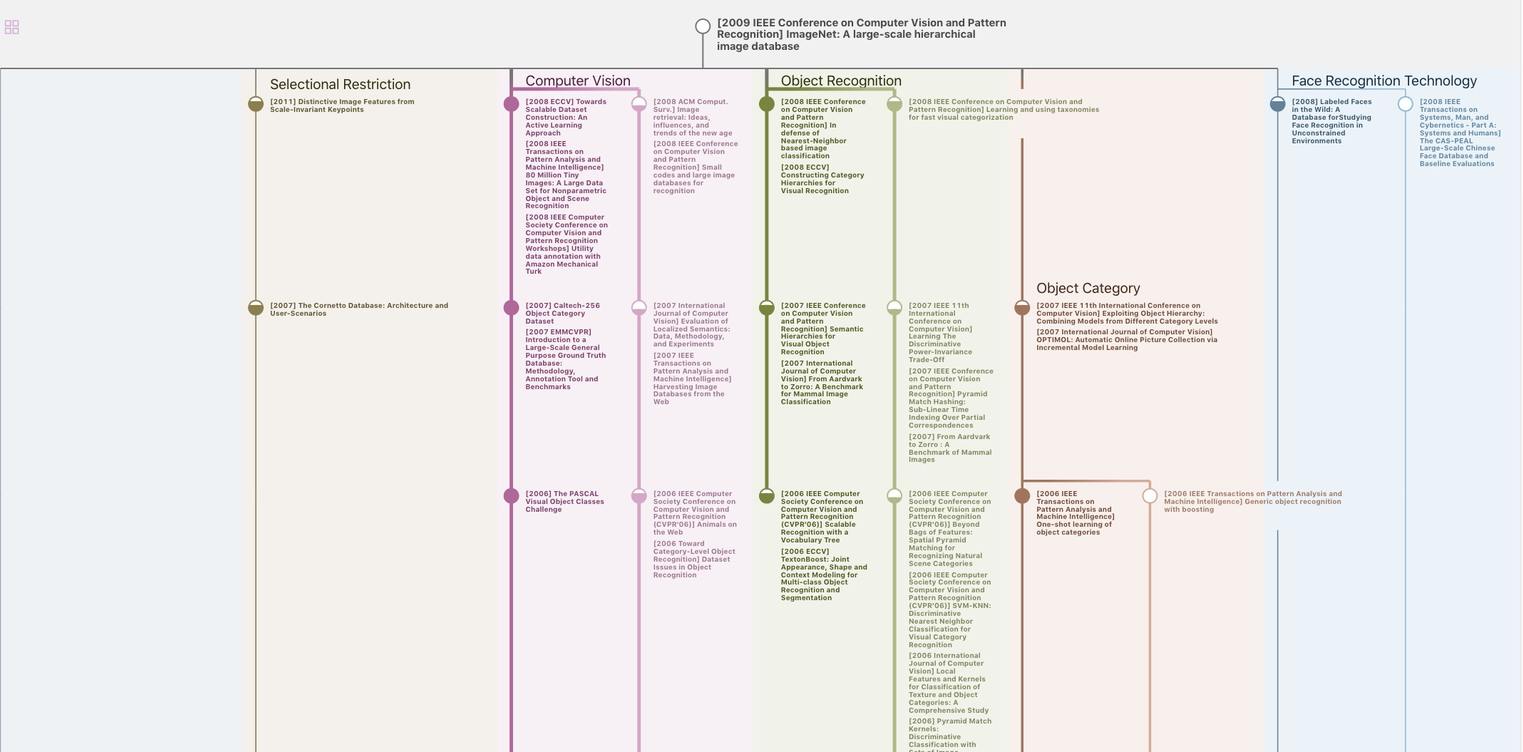
生成溯源树,研究论文发展脉络
Chat Paper
正在生成论文摘要