Granularity-driven trisecting-and-learning models for interval-valued rule induction
Applied Intelligence(2023)
摘要
Three-way decision (3WD) theory and granular computing have recently received much attention as methods for thinking and problem solving. Based on the trisecting-and-acting model in the 3WD, this paper proposes two trisecting-and-learning models for rule induction, which begin with a constructed concept space and a search for the most suitable level of granularity in high-to-low and low-to-high manners, respectively. The learning results are sets of granules that can induce raw rules. By combining the advantages of granular computing and the trisecting-and-acting method, the models only need to process one or two parts of the trisecting result, simplifying the calculation. An interval-valued rule-inducing algorithm is proposed to obtain more general rules from raw rules. It coarsens the granularity further by merging the granules through the maximal adjacent relation of the learned granules. The interval-valued rules are obtained at the same time. The models in the paper are compatible with incomplete mixed data containing numeric, symbolic, do not care, and lost types. Symbolic data are converted into numeric types by considering their semantic order. This type of conversion enables intervals to be generated from symbolic data, producing more general rules. The rationality for allowing rules with missing data is also considered in this paper. Comparative analysis with existing works and experiments reveals the improvements of our models. Although there are a small fraction of incomplete rules and fewer intervalized rules on numeric data, the rules we induce have a higher coverage ratio and a much larger intervalized ratio for symbolic data. Moreover, our model induces simpler rules for all types of datasets.
更多查看译文
关键词
Trisecting-and-acting,Rule induction,Granular computing,Three-way decision,Interval processing,Incomplete data
AI 理解论文
溯源树
样例
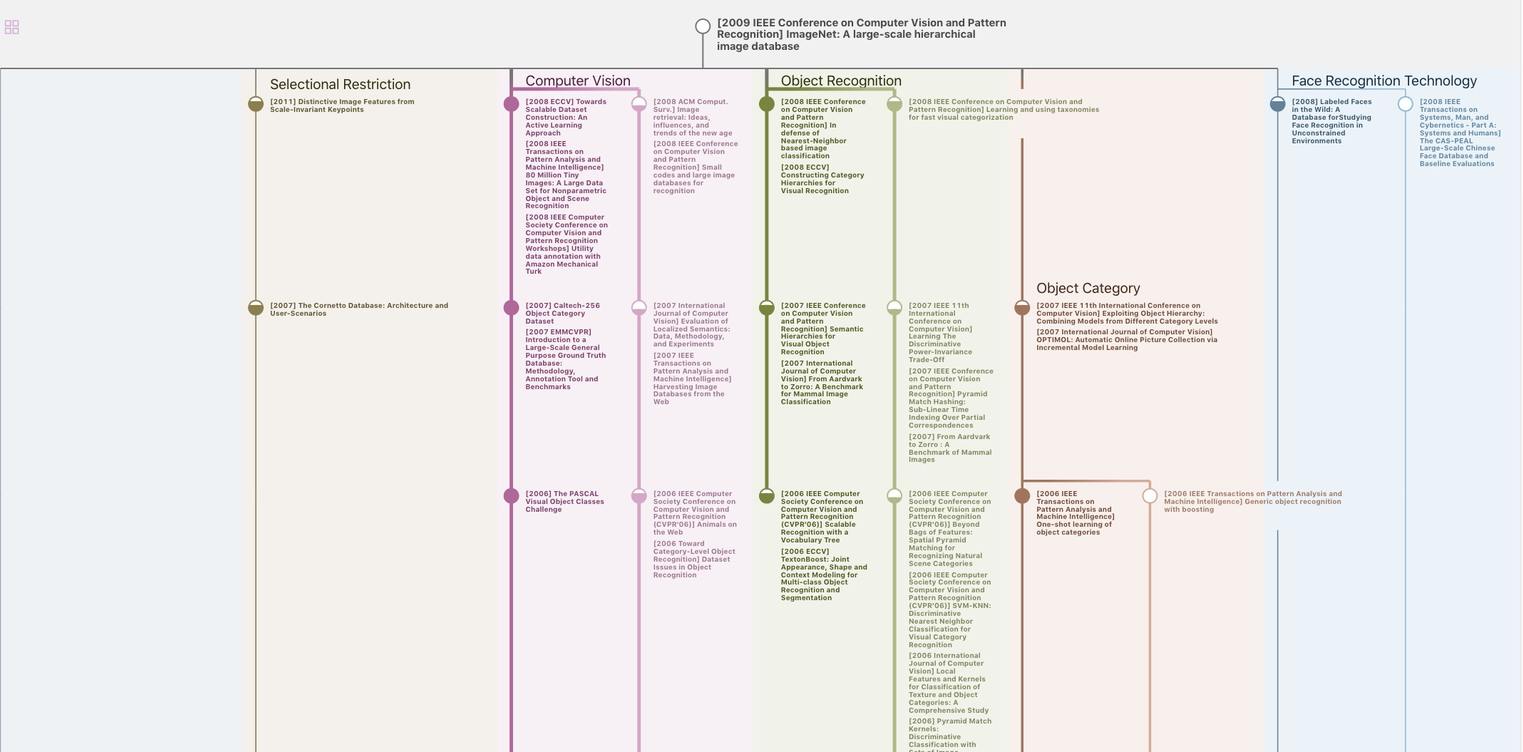
生成溯源树,研究论文发展脉络
Chat Paper
正在生成论文摘要