Olfactory perception prediction model inspired by olfactory lateral inhibition and deep feature combination
Applied Intelligence(2023)
摘要
Finding the relationship between the chemical structure and physicochemical properties of odor molecules and olfactory perception prediction, i.e. quantitative structure-odor relationship (QSOR), remains a challenging, decades-old task. With the development of deep learning, data-driven methods such as convolutional neural networks or deep neural networks have gradually been used to predict QSOR. However, the differences between the molecular structure of different molecules are subtle and complex, the molecular feature descriptors are numerous and their interactions are complex. In this paper, we propose the Lateral Inhibition-inspired feature pyramid dynamic Convolutional Network, using the feature pyramid network as the backbone network to extract the odor molecular structure features, which can deal with multi-scale changes well. Imitating the lateral inhibition mechanism of animal olfactory, we add the lateral inhibition-inspired attention maps to the dynamic convolution, to improve the prediction accuracy of olfactory perception prediction. Besides, due to a large number of molecular feature descriptors and their complex interactions, we propose to add Attentional Factorization Mechanism to a deep neural network to obtain molecular descriptive features through weighted deep feature combination based on the attention mechanism. Our proposed olfactory perception prediction model noted as LIFMCN has achieved a state-of-the-art result and will help the product design and quality assessment in food, beverage, and fragrance industries.
更多查看译文
关键词
Olfactory perception prediction, Quantitative structure-odor relationship, Convolutional neural network, Lateral inhibition, Factorization mechanism
AI 理解论文
溯源树
样例
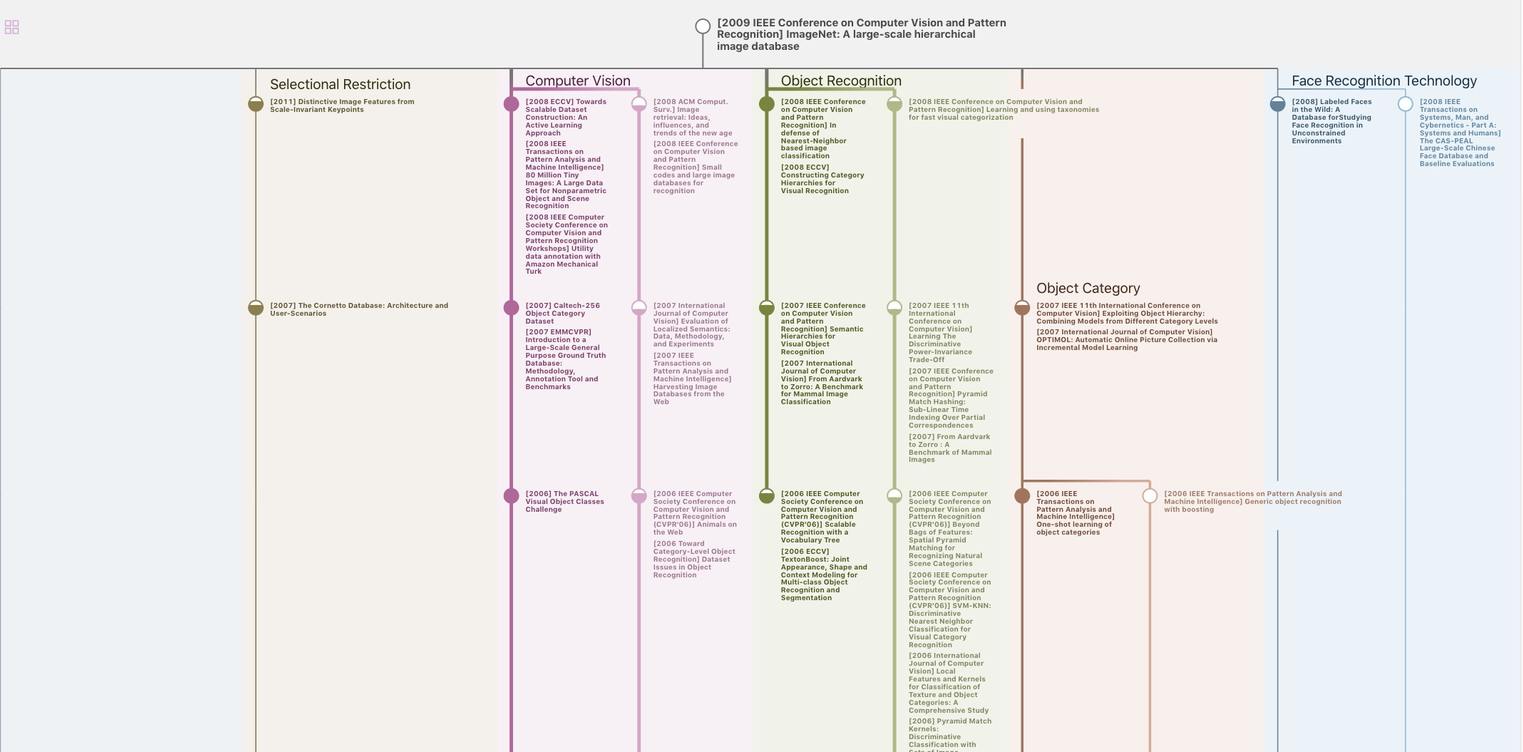
生成溯源树,研究论文发展脉络
Chat Paper
正在生成论文摘要