Prognostic value of deep learning–based fibrosis quantification on chest CT in idiopathic pulmonary fibrosis
European radiology(2023)
摘要
Objective To investigate the prognostic value of deep learning (DL)–driven CT fibrosis quantification in idiopathic pulmonary fibrosis (IPF). Methods Patients diagnosed with IPF who underwent nonenhanced chest CT and spirometry between 2005 and 2009 were retrospectively collected. Proportions of normal (CT-Norm%) and fibrotic lung volume (CT-Fib%) were calculated on CT using the DL software. The correlations of CT-Norm% and CT-Fib% with forced vital capacity (FVC) and diffusion capacity of carbon monoxide (D LCO ) were evaluated. The multivariable-adjusted hazard ratios (HRs) of CT-Norm% and CT-Fib% for overall survival were calculated with clinical and physiologic variables as covariates using Cox regression. The feasibility of substituting CT-Norm% for D LCO in the GAP index was investigated using time-dependent areas under the receiver operating characteristic curve (TD-AUCs) at 3 years. Results In total, 161 patients (median age [IQR], 68 [62–73] years; 104 men) were evaluated. CT-Norm% and CT-Fib% showed significant correlations with FVC (Pearson’s r , 0.40 for CT-Norm% and − 0.37 for CT-Fib%; both p < 0.001) and D LCO (0.52 for CT-Norm% and − 0.46 for CT-Fib%; both p < 0.001). On multivariable Cox regression, both CT-Norm% and CT-Fib% were independent prognostic factors when adjusted to age, sex, smoking status, comorbid chronic diseases, FVC, and D LCO (HRs, 0.98 [95% CI 0.97–0.99; p < 0.001] for CT-Norm% at 3 years and 1.03 [1.01–1.05; p = 0.01] for CT-Fib%). Substituting CT-Norm% for D LCO showed comparable discrimination to the original GAP index (TD-AUC, 0.82 [0.78–0.85] vs. 0.82 [0.79–0.86]; p = 0.75). Conclusion CT-Norm% and CT-Fib% calculated using chest CT–based deep learning software were independent prognostic factors for overall survival in IPF. Key Points • Normal and fibrotic lung volume proportions were automatically calculated using commercial deep learning software from chest CT taken from 161 patients diagnosed with idiopathic pulmonary fibrosis. • CT-quantified volumetric parameters from commercial deep learning software were correlated with forced vital capacity (Pearson’s r, 0.40 for normal and − 0.37 for fibrotic lung volume proportions) and diffusion capacity of carbon monoxide (Pearson’s r, 0.52 and − 0.46, respectively). • Normal and fibrotic lung volume proportions (hazard ratios, 0.98 and 1.04; both p < 0.001) independently predicted overall survival when adjusted for clinical and physiologic variables.
更多查看译文
关键词
Deep learning,Idiopathic pulmonary fibrosis,Multidetector computed tomography,Prognosis
AI 理解论文
溯源树
样例
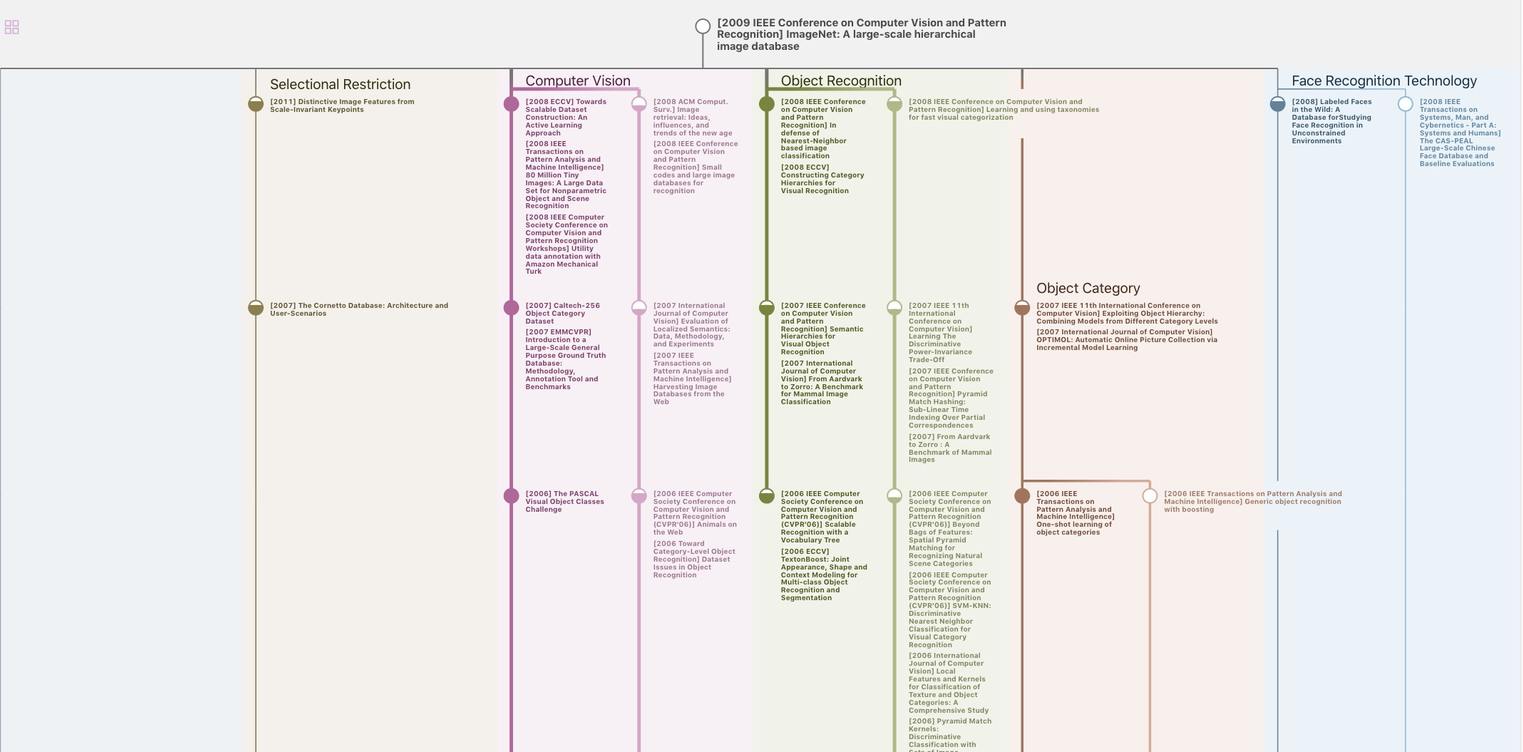
生成溯源树,研究论文发展脉络
Chat Paper
正在生成论文摘要