On the importance of interpretable machine learning predictions to inform clinical decision making in oncology.
Frontiers in oncology(2023)
摘要
Machine learning-based tools are capable of guiding individualized clinical management and decision-making by providing predictions of a patient's future health state. Through their ability to model complex nonlinear relationships, ML algorithms can often outperform traditional statistical prediction approaches, but the use of nonlinear functions can mean that ML techniques may also be less interpretable than traditional statistical methodologies. While there are benefits of intrinsic interpretability, many model-agnostic approaches now exist and can provide insight into the way in which ML systems make decisions. In this paper, we describe how different algorithms can be interpreted and introduce some techniques for interpreting complex nonlinear algorithms.
更多查看译文
关键词
decision-making support,high-stakes prediction,interpretability and explainability,opaque machine learning models,precision medicine
AI 理解论文
溯源树
样例
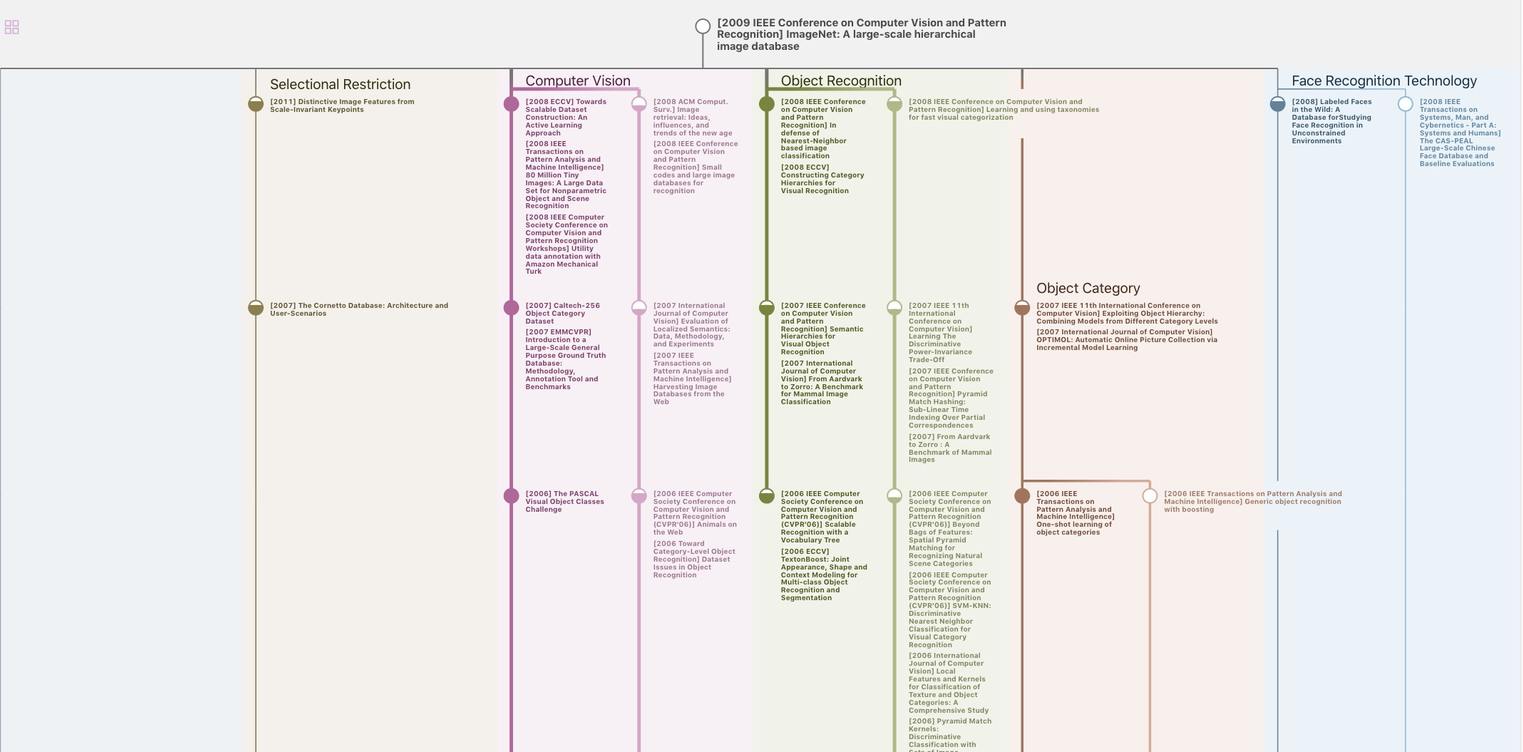
生成溯源树,研究论文发展脉络
Chat Paper
正在生成论文摘要