Learning Spatio-Temporal Aggregations for Large-Scale Capacity Expansion Problems
PROCEEDINGS OF THE 2023 ACM/IEEE 14TH INTERNATIONAL CONFERENCE ON CYBER-PHYSICAL SYSTEMS, WITH CPS-IOTWEEK 2023(2023)
摘要
Effective investment planning decisions are crucial to ensure that critical cyber-physical infrastructures satisfy performance requirements over an extended time horizon. Computing these decisions often requires solving Capacity Expansion Problems (CEPs). In the context of regional-scale energy systems, these problems are prohibitively expensive to solve owing to large network sizes, heterogeneous node characteristics (e.g., electric power and natural gas energy vectors), and a large number of operational periods. To maintain tractability, traditional approaches resort to aggregating network nodes and/or selecting a set of representative time periods. Often, these reductions do not capture the supply-demand variations that crucially impact the CEP costs and constraints, leading to suboptimal decisions. Here, we propose a novel graph convolutional autoencoder approach for spatiotemporal aggregation of a generic CEP with heterogeneous nodes (CEPHN). Our autoencoder architecture leverages graph pooling to identify nodes with similar characteristics and minimizes a multi-objective loss function. This loss function is specifically tailored to induce desirable spatial and temporal aggregations in terms of tractability and optimality of CEPHN. In particular, the output of the graph pooling provides a spatial aggregation while clustering the low-dimensional encoded representations yields a temporal aggregation. We apply our approach to generation expansion planning of coupled power and natural gas system in New England. The resulting spatiotemporal aggregation leads to a simpler CEPHN with 6 nodes (as opposed to 88 nodes in the original system) and a small set of representative days selected from a full year. We evaluate aggregation outcomes over a range of hyperparameters governing the loss function, and compare resulting upper bounds on the original problem with those obtained using previously known methods. The results from our case study show that this approach provides solutions that are 33% (resp. 10%) better those than obtained from standard spatial (resp. temporal) aggregation approaches.
更多查看译文
关键词
Dimensionality Reduction,Clustering,Graph Neural Networks,Mixed Discrete/Continuous Optimization,Power System Planning
AI 理解论文
溯源树
样例
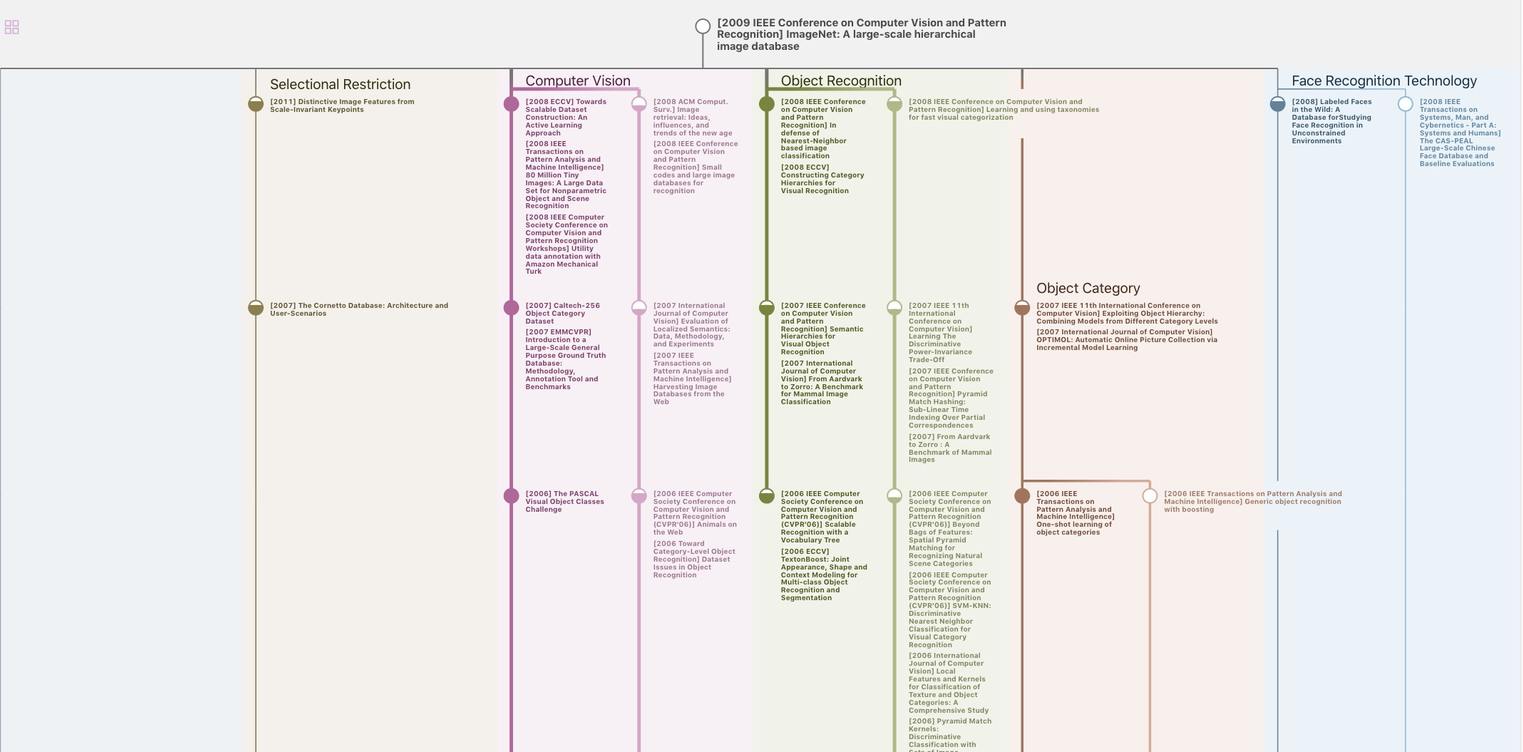
生成溯源树,研究论文发展脉络
Chat Paper
正在生成论文摘要