An improved lightweight and real-time YOLOv5 network for detection of surface defects on indocalamus leaves
Journal of Real-Time Image Processing(2023)
摘要
Indocalamus leaves are widely used in the Chinese food industry. Surface defect detection plays a crucial role in the post-harvest reprocessing of indocalamus leaves. In this study, we constructed a lightweight convolutional neural network model to detect surface defects on indocalamus leaves. We investigated four categories of surface defects, including damage, black spots, insect spots, and holes, to construct a dataset of surface defects on indocalamus leaves, which contained 4124 images for model training and evaluation. We replaced the original path aggregation network (PANet) in YOLOv5 with a cross-layer feature pyramid network (CFPN), which improved the detection performance by fusing feature maps at different levels. We proposed an improved feature fusion module, named the receptive dilated and deformable convolution field block (RDDCFB), which was integrated into the CFPN for learning within larger spatial and semantic contexts. Furthermore, a new CA mechanism was proposed to improve the feature representation capability of the network by appropriately adjusting the structure of the coordinate attention (CA) mechanism. Extensive experiments using the Pascal VOC and CIFAR-100 datasets demonstrated that this new CA block had superior accuracy and integration capabilities. On MSCOCO2017 validation datasets, experiments show that our module is consistently better than various detectors, including Faster R-CNN, YOLOv3, and YOLOv4. Finally, our quantitative results from the dataset of surface defects on indocalamus leaves indicated the effectiveness of the proposed method. The accuracy and recognition efficiency of the improved YOLOv5 model could reach 97.7% and 97 frames per second, respectively.
更多查看译文
关键词
Attention mechanism, Defect detection, Feature pyramid network, Multi-scale object, YOLOv5
AI 理解论文
溯源树
样例
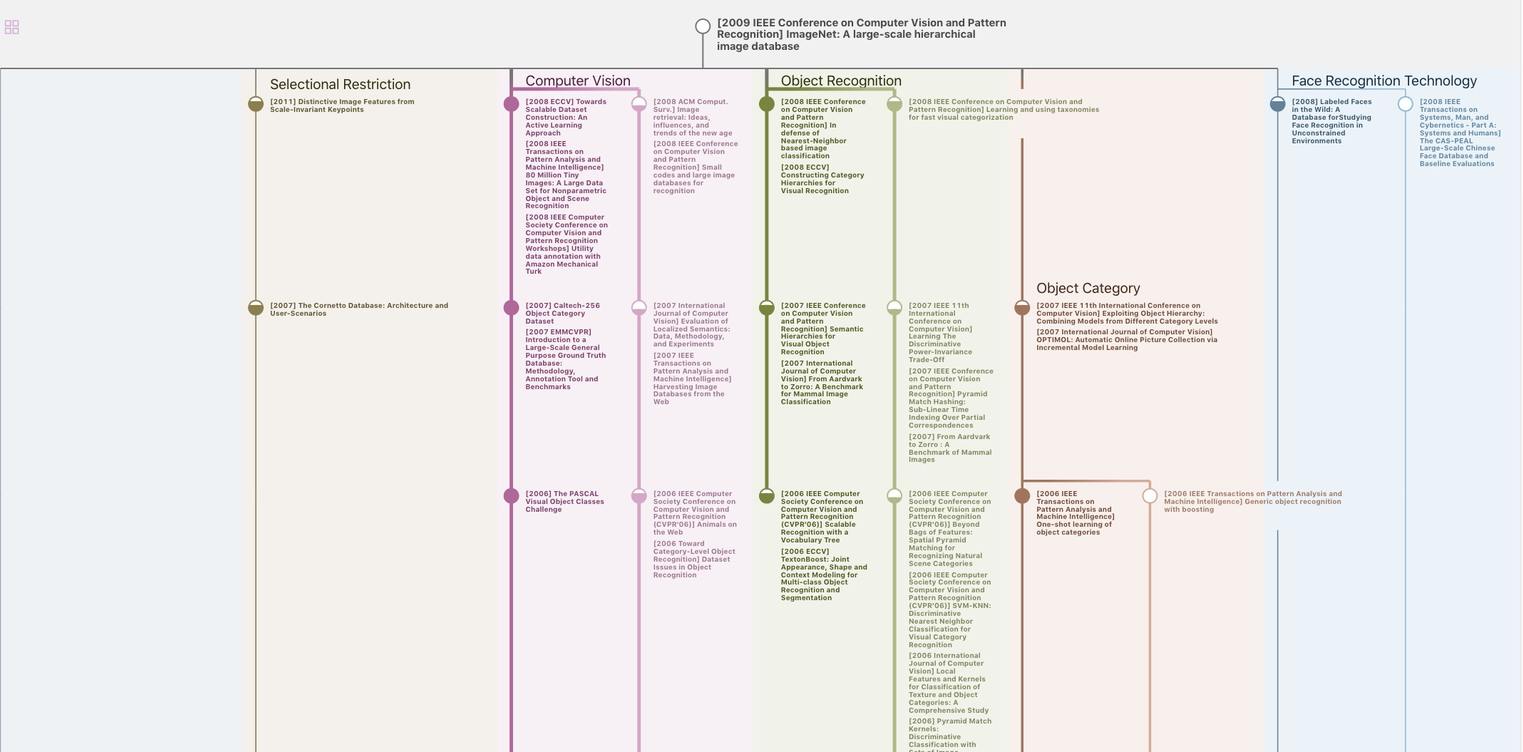
生成溯源树,研究论文发展脉络
Chat Paper
正在生成论文摘要