Policy Gradient Converges to the Globally Optimal Policy for Nearly Linear-Quadratic Regulators
arxiv(2023)
摘要
Nonlinear control systems with partial information to the decision maker are
prevalent in a variety of applications. As a step toward studying such
nonlinear systems, this work explores reinforcement learning methods for
finding the optimal policy in the nearly linear-quadratic regulator systems. In
particular, we consider a dynamic system that combines linear and nonlinear
components, and is governed by a policy with the same structure. Assuming that
the nonlinear component comprises kernels with small Lipschitz coefficients, we
characterize the optimization landscape of the cost function. Although the cost
function is nonconvex in general, we establish the local strong convexity and
smoothness in the vicinity of the global optimizer. Additionally, we propose an
initialization mechanism to leverage these properties. Building on the
developments, we design a policy gradient algorithm that is guaranteed to
converge to the globally optimal policy with a linear rate.
更多查看译文
关键词
globally optimal policy,linear-quadratic
AI 理解论文
溯源树
样例
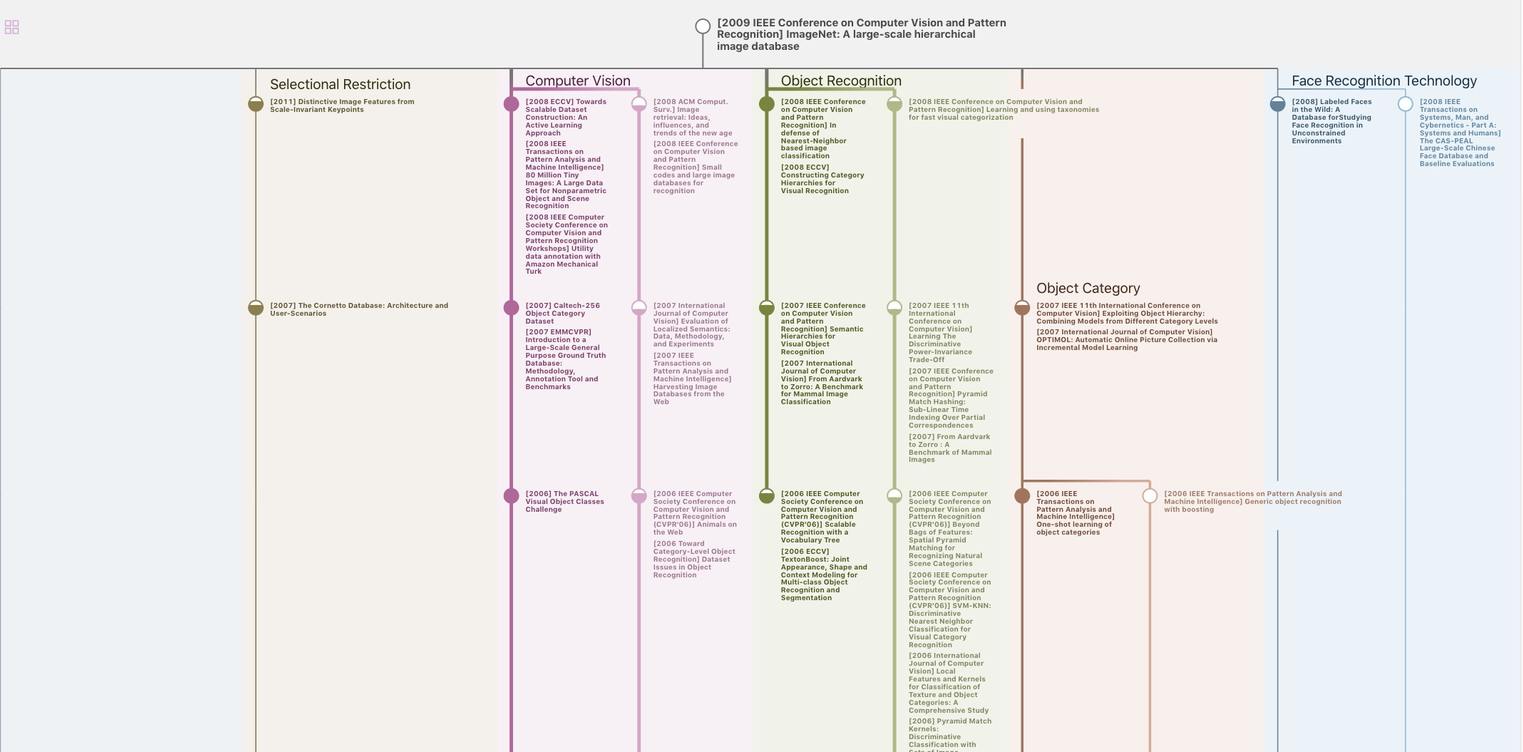
生成溯源树,研究论文发展脉络
Chat Paper
正在生成论文摘要