A Comparative Analysis of Machine and Deep Learning Classifiers for Intrusion Detection
2023 5th International Conference on Smart Systems and Inventive Technology (ICSSIT)(2023)
摘要
Presently available security tools are eluded by polymorphic malware, zero-day vulnerabilities, and unauthorized attempts. These open up loophole points in the systems for intruders to access the private network as legitimate users. An adversary can use similar commands, scripts, tools, and credentials as a system administrator to make it impossible to find the difference between a legitimate user and the intruder. A good classifier aims to classify such events automatically based on learning strategies. This study aims to analyze the appropriate classifier for the use of the Industrial Internet of Thing (IIoT). Some of the well-known classifiers from the domain of machine and deep learning techniques are experimented. The results are compared to evaluate the model applicable to industrial devices and provide appropriate security. This study utilizes two popular datasets: the UNSWNB-15 and KDD+ datasets to experiment with the models. Finally, a comparative analysis is evaluated based on accuracy, false rate, and time is taken for detection. Deep learning models claim better efficiency and thus, are suitable for IIoTs significantly.
更多查看译文
关键词
Internet of Thing,Industrial,Machine Learning,Deep Learning,Classifier.
AI 理解论文
溯源树
样例
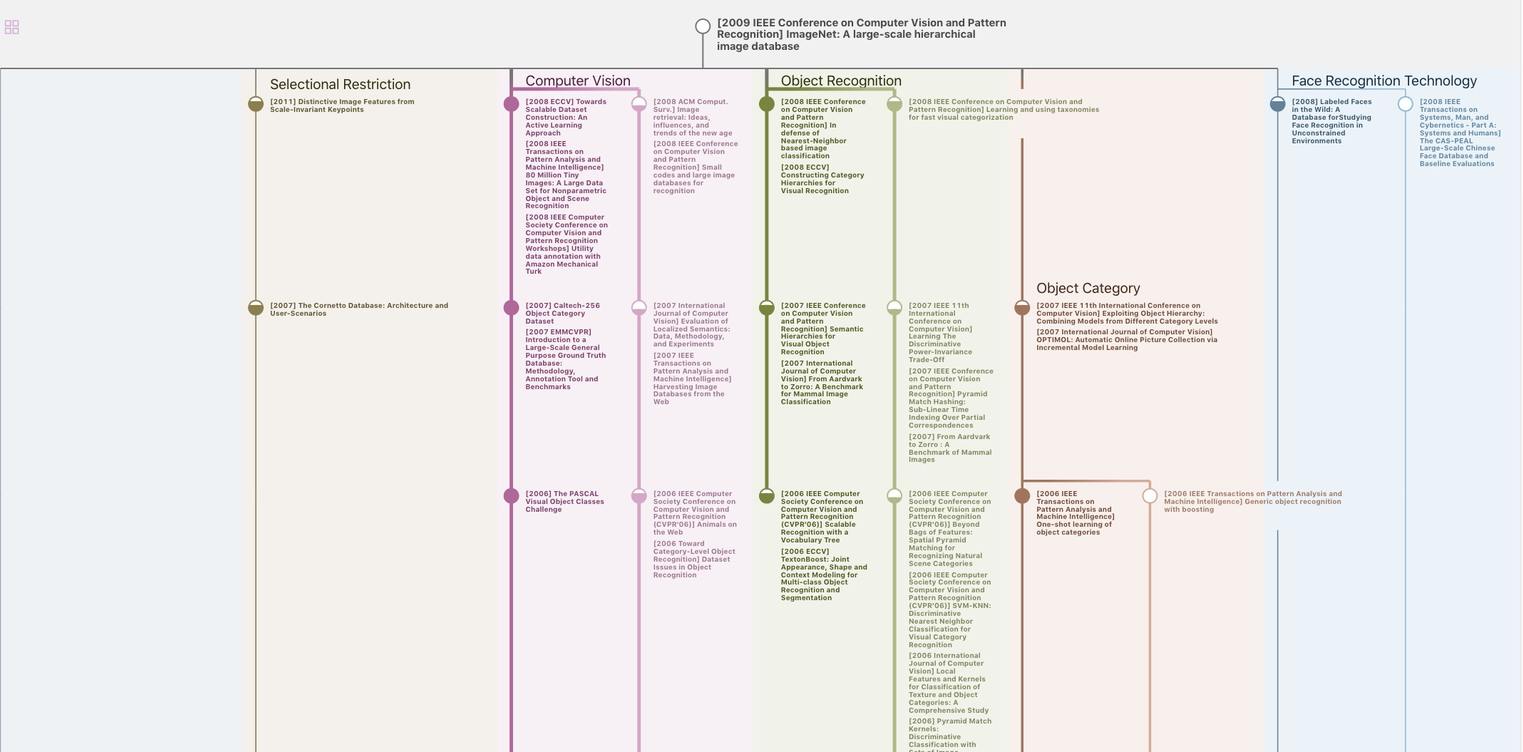
生成溯源树,研究论文发展脉络
Chat Paper
正在生成论文摘要