Exploiting the Self-Attention Mechanism in Gas Sensor Array (GSA) Data With Neural Networks
IEEE Sensors Journal(2023)
摘要
Gas sensor array (GSA) data is a sequential series of values that represents the temporal conditions of the existence/absence/mixture of gases and exhibits similarities to the textual stream of natural languages that represents semantic information. We speculate and subsequently prove that there also exist self-attention mechanisms in GSA data that can be exploited for gas classification and recognition. We first convert GSA data into a 1-D token series (called WORDs in this work) through sampling and quantization of the sensor values and then use an enhanced long short-term memory (LSTM) revision network, called LSTM-attention, to extract the self-attention mechanism in the GSA data. We demonstrate that LSTM-attention achieves a much better performance (99.6%) than CNN-based networks as well as other GSA data process techniques on UCI dynamic gases dataset. We also find out that the self-attention mechanism varies with different sampling and quantization levels during data acquisition.
更多查看译文
关键词
Gas classification,gas sensor array (GSA),long short-term memory (LSTM),self-attention mechanism
AI 理解论文
溯源树
样例
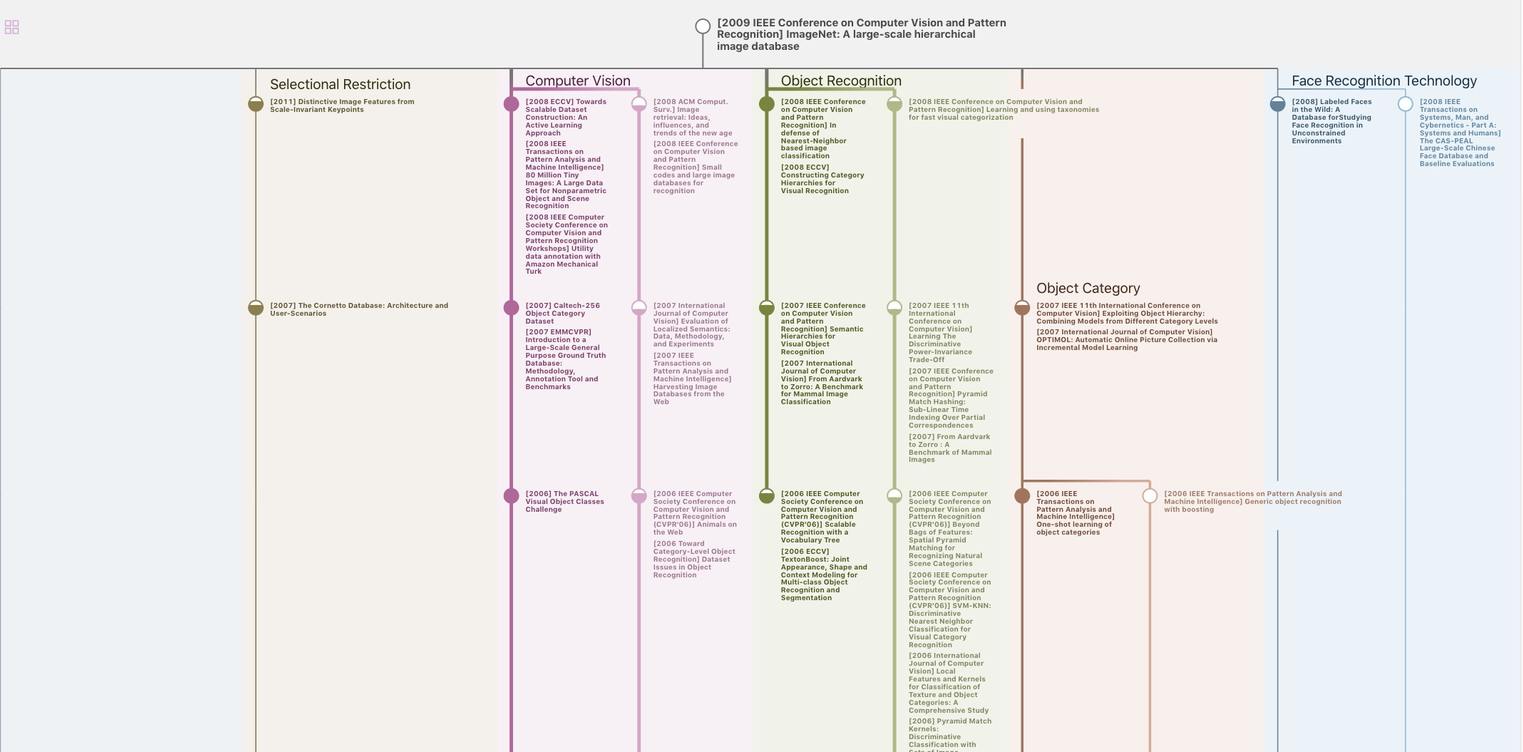
生成溯源树,研究论文发展脉络
Chat Paper
正在生成论文摘要