A Predictor-Corrector Method for Multi-objective Optimization in Fair Machine Learning
2022 IEEE/ACM International Conference on Big Data Computing, Applications and Technologies (BDCAT)(2022)
摘要
Issues of fairness often arise in graphical neural networks used for misinformation detection. However, improving fairness can often come at the cost of reducing accuracy and vice versa. Therefore, we formulate the task of balancing accuracy and fairness as a multi-objective optimization (MOO) problem where we seek to find a set of Pareto optimal solutions. Traditional first-order approaches to solving MOO problems such as multi-gradient descent can be costly, especially with large neural networks. Instead, we describe a more efficient approach using the predictor-corrector method. Given an initial Pareto optimal point, this approach predicts the direction of a neighboring solution and refines this prediction using a few steps of multi-gradient descent. We show experimentally that this approach allows for the generation of high-quality Pareto fronts faster than baseline optimization methods.
更多查看译文
关键词
multi-objective optimization,fair machine learning,iterative methods,neural networks
AI 理解论文
溯源树
样例
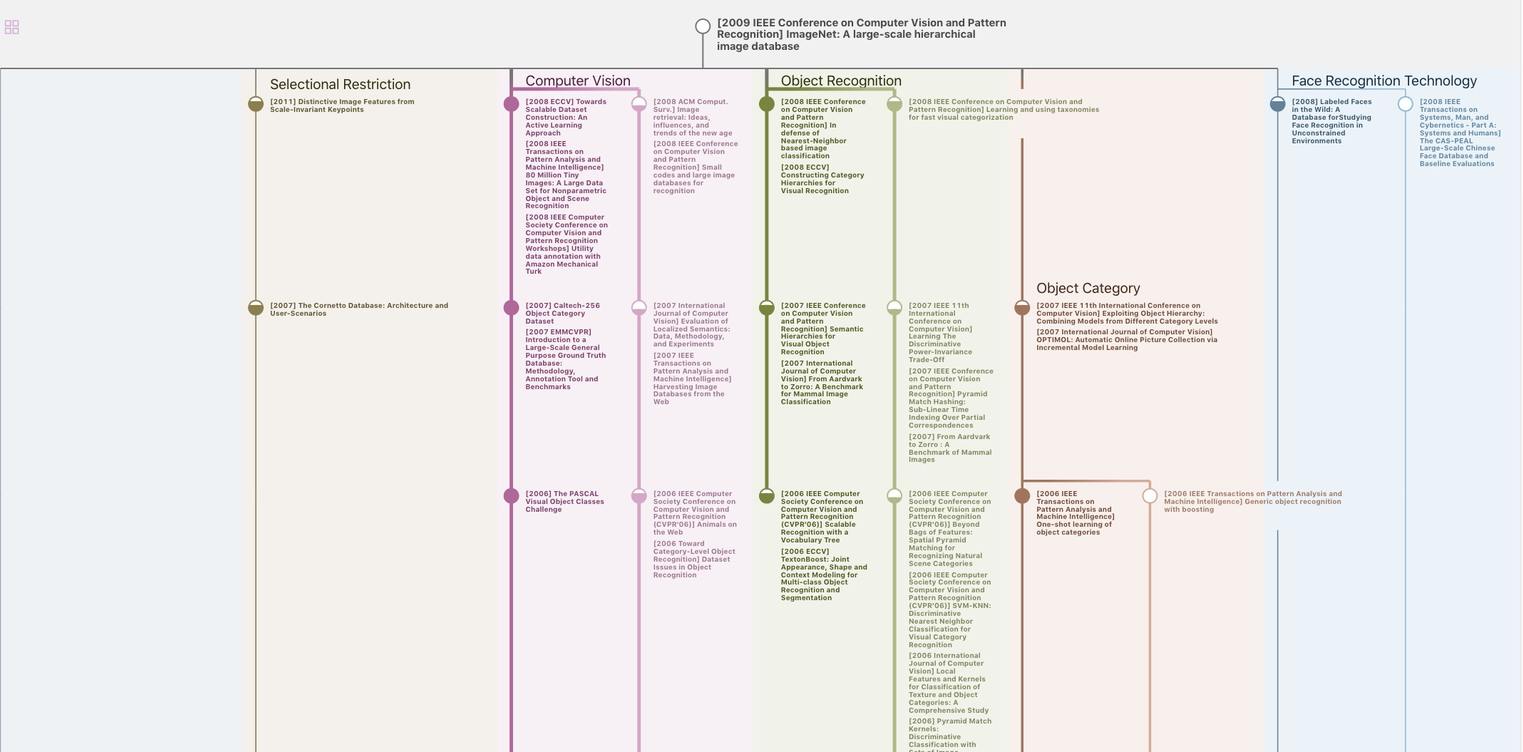
生成溯源树,研究论文发展脉络
Chat Paper
正在生成论文摘要