Supporting Green Neuromorphic Computing: Machine Learning Guided Microfabrication for Resistive Random Access Memory
2022 IEEE/ACM International Conference on Big Data Computing, Applications and Technologies (BDCAT)(2022)
摘要
The growing popularity of big data and machine learning applications call for a more powerful and energy-efficient way to execute deep learning workflows. Neuromorphic chips provide a potential solution, as they attempt to mimic the neuronal architectures in human brain and show great potentials in reducing energy consumption in the order of magnitude and also improve the computational performance. However, the fabrication process for neuromophic chips is costly and currently based on trial-and-error, which adds complexity to the design process. In this paper, we address this challenge by designing and developing machine learning guided microfabrication process for Resistive Random Access Memory (RRAM), which is a key device in neuromorphic chips. Experimental results show that our approach is effective in terms of predicting the performance of RRAM devices fabricated under various process conditions.
更多查看译文
关键词
Resistive Random Access Memory,Neuromorphic Computing,Machine Learning,Big Data,Microfabrication
AI 理解论文
溯源树
样例
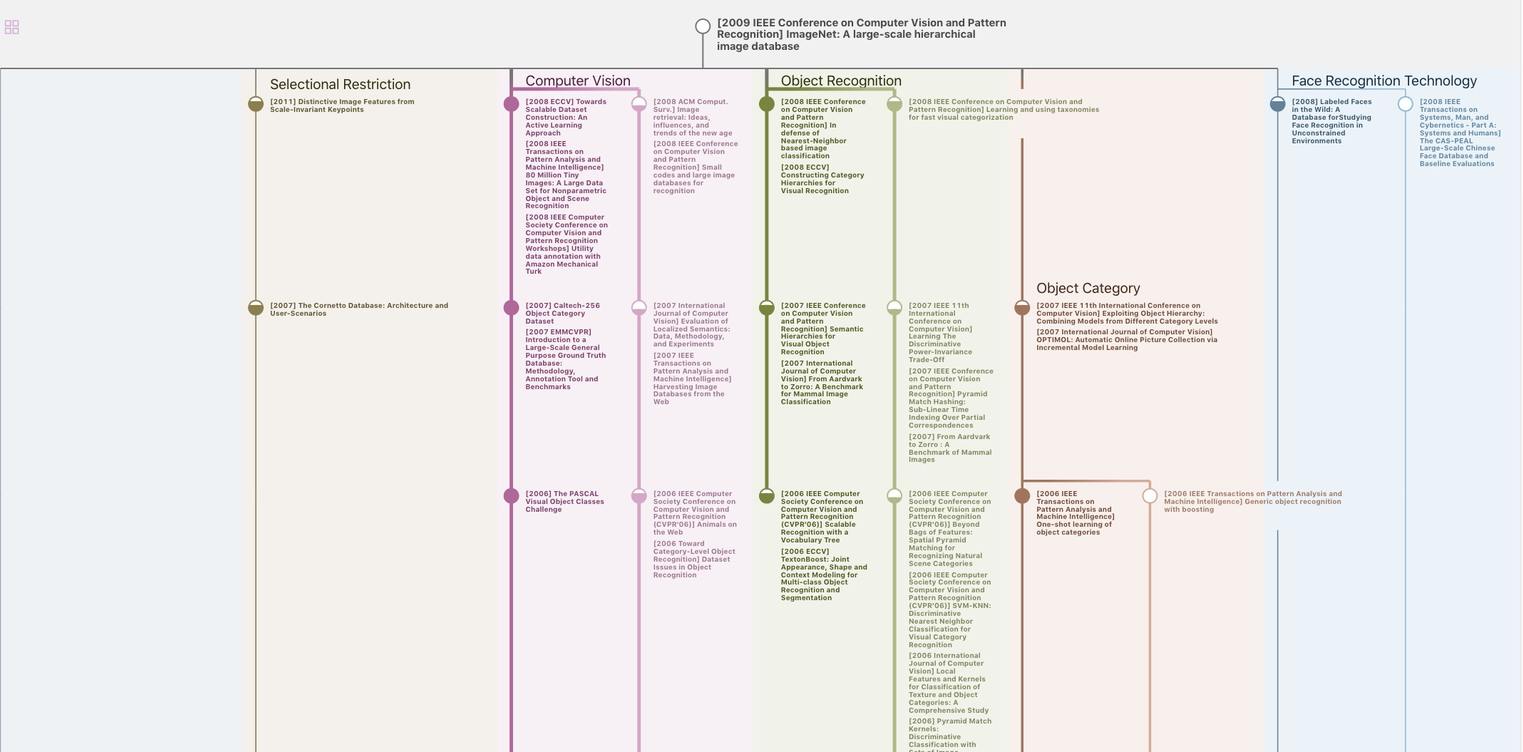
生成溯源树,研究论文发展脉络
Chat Paper
正在生成论文摘要