Revisit Parameter-Efficient Transfer Learning: A Two-Stage Paradigm
arxiv(2023)
摘要
Parameter-Efficient Transfer Learning (PETL) aims at efficiently adapting large models pre-trained on massive data to downstream tasks with limited task-specific data. In view of the practicality of PETL, previous works focus on tuning a small set of parameters for each downstream task in an end-to-end manner while rarely considering the task distribution shift issue between the pre-training task and the downstream task. This paper proposes a novel two-stage paradigm, where the pre-trained model is first aligned to the target distribution. Then the task-relevant information is leveraged for effective adaptation. Specifically, the first stage narrows the task distribution shift by tuning the scale and shift in the LayerNorm layers. In the second stage, to efficiently learn the task-relevant information, we propose a Taylor expansion-based importance score to identify task-relevant channels for the downstream task and then only tune such a small portion of channels, making the adaptation to be parameter-efficient. Overall, we present a promising new direction for PETL, and the proposed paradigm achieves state-of-the-art performance on the average accuracy of 19 downstream tasks.
更多查看译文
关键词
learning,transfer,parameter-efficient,two-stage
AI 理解论文
溯源树
样例
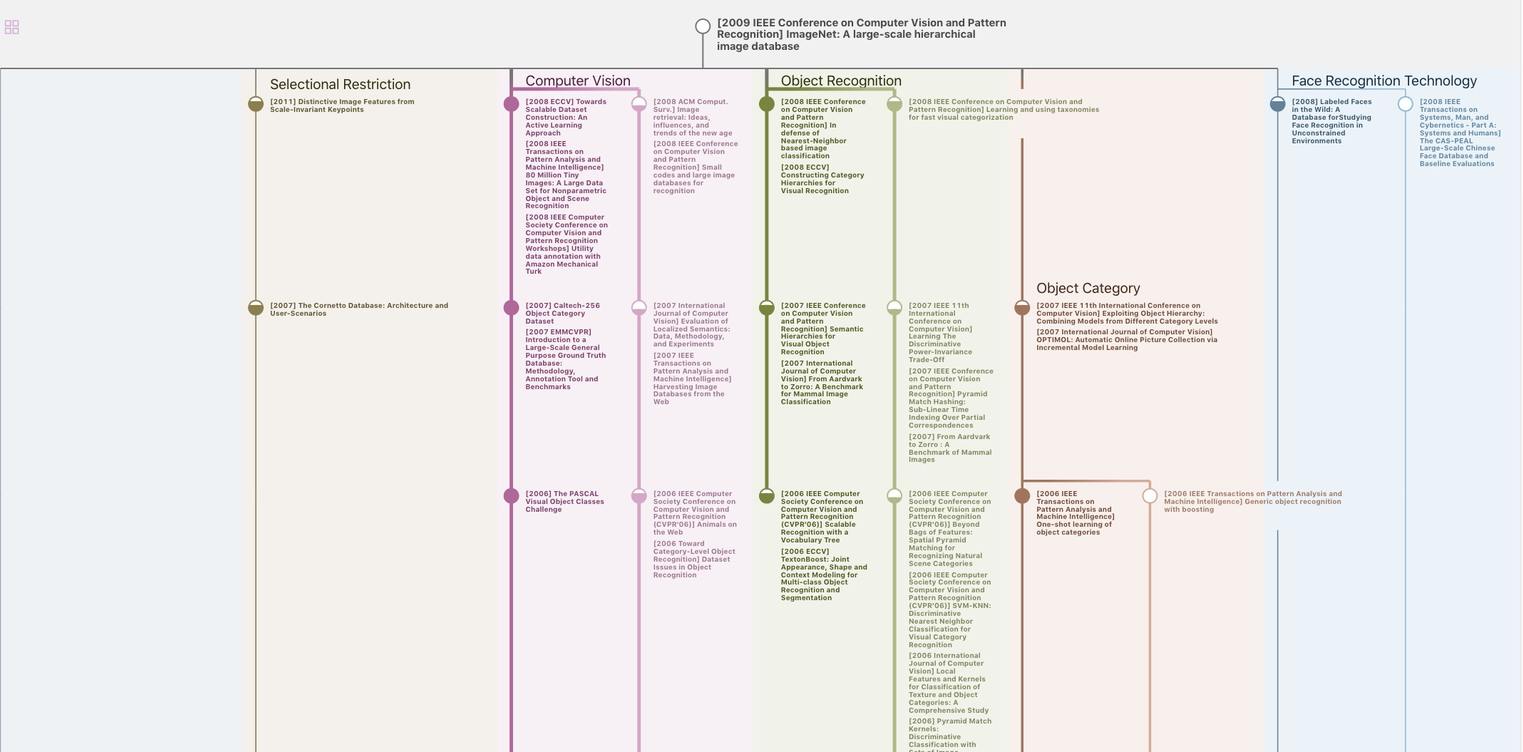
生成溯源树,研究论文发展脉络
Chat Paper
正在生成论文摘要