Automatic Locally Stationary Time Series Forecasting with application to predicting U.K. Gross Value Added Time Series under sudden shocks caused by the COVID pandemic
arxiv(2023)
摘要
Accurate forecasting of the U.K. gross value added (GVA) is fundamental for measuring the growth of the U.K. economy. A common nonstationarity in GVA data, such as the ABML series, is its increase in variance over time due to inflation. Transformed or inflation-adjusted series can still be challenging for classical stationarity-assuming forecasters. We adopt a different approach that works directly with the GVA series by advancing recent forecasting methods for locally stationary time series. Our approach results in more accurate and reliable forecasts, and continues to work well even when the ABML series becomes highly variable during the COVID pandemic.
更多查看译文
关键词
covid,sudden shocks,pandemic,stationary
AI 理解论文
溯源树
样例
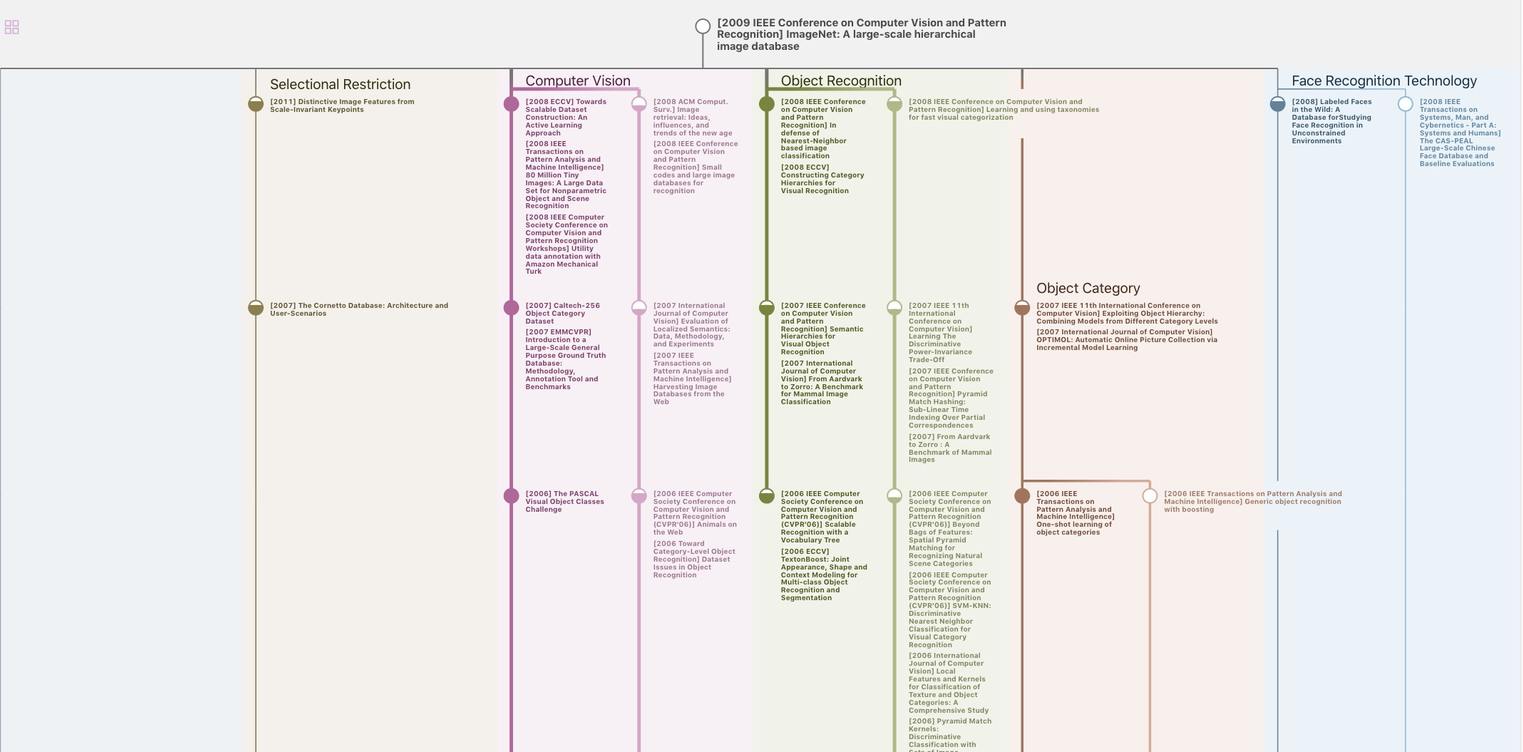
生成溯源树,研究论文发展脉络
Chat Paper
正在生成论文摘要