A Workflow for Statistical Inference in Stochastic Gradient Descent
arxiv(2023)
摘要
Stochastic gradient descent (SGD) is an estimation tool for large data employed in machine learning and statistics. Due to the Markovian nature of the SGD process, inference is a challenging problem. An underlying asymptotic normality of the averaged SGD (ASGD) estimator allows for the construction of a batch-means estimator of the asymptotic covariance matrix. Instead of the usual increasing batch-size strategy employed in ASGD, we propose a memory efficient equal batch-size strategy and show that under mild conditions, the estimator is consistent. A key feature of the proposed batching technique is that it allows for bias-correction of the variance, at no cost to memory. Since joint inference for high dimensional problems may be undesirable, we present marginal-friendly simultaneous confidence intervals, and show through an example how covariance estimators of ASGD can be employed in improved predictions.
更多查看译文
关键词
statistical inference,gradient,workflow
AI 理解论文
溯源树
样例
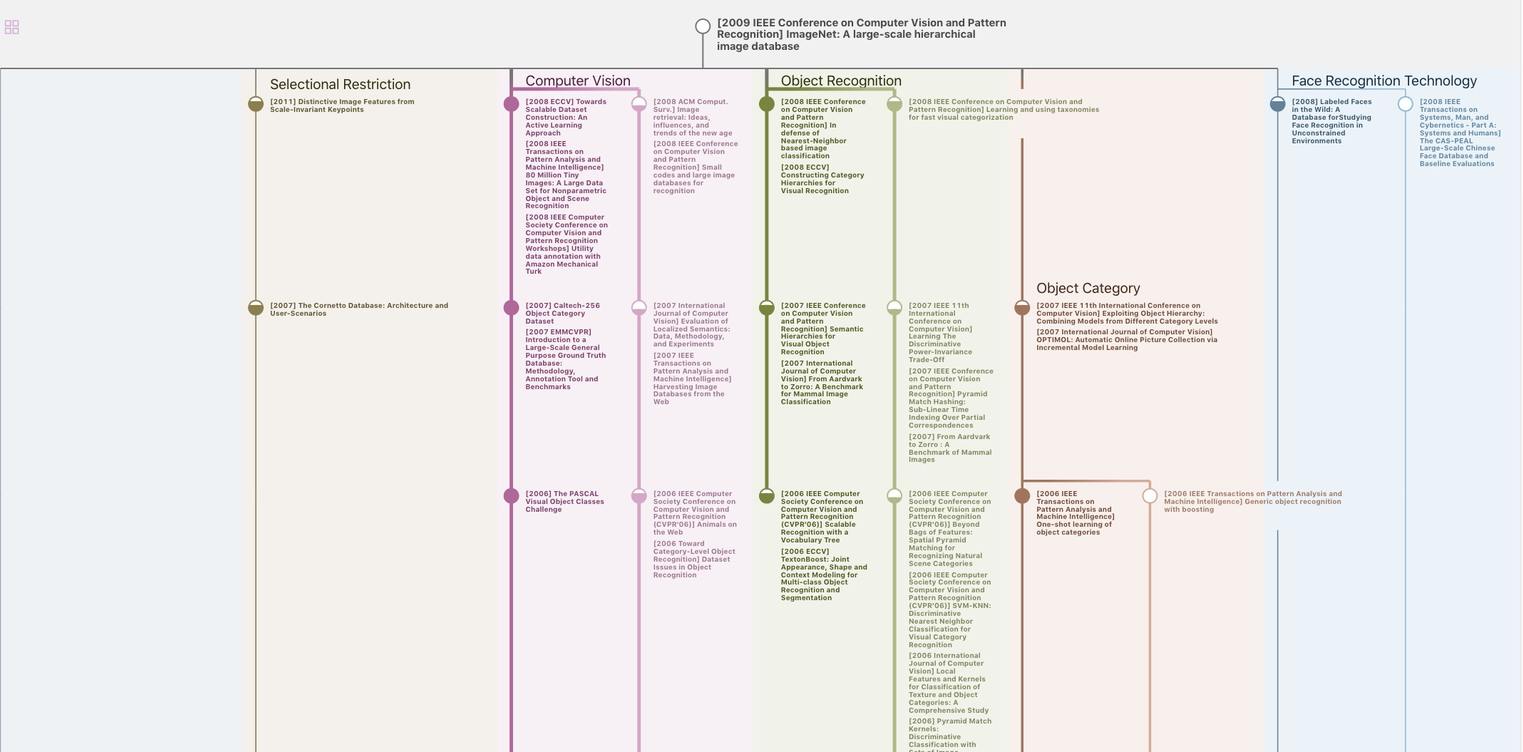
生成溯源树,研究论文发展脉络
Chat Paper
正在生成论文摘要