SuperMask: Generating High-resolution object masks from multi-view, unaligned low-resolution MRIs
arXiv (Cornell University)(2023)
摘要
Three-dimensional segmentation in magnetic resonance images (MRI), which reflects the true shape of the objects, is challenging since high-resolution isotropic MRIs are rare and typical MRIs are anisotropic, with the out-of-plane dimension having a much lower resolution. A potential remedy to this issue lies in the fact that often multiple sequences are acquired on different planes. However, in practice, these sequences are not orthogonal to each other, limiting the applicability of many previous solutions to reconstruct higher-resolution images from multiple lower-resolution ones. We propose a weakly-supervised deep learning-based solution to generating high-resolution masks from multiple low-resolution images. Our method combines segmentation and unsupervised registration networks by introducing two new regularizations to make registration and segmentation reinforce each other. Finally, we introduce a multi-view fusion method to generate high-resolution target object masks. The experimental results on two datasets show the superiority of our methods. Importantly, the advantage of not using high-resolution images in the training process makes our method applicable to a wide variety of MRI segmentation tasks.
更多查看译文
关键词
high-resolution,multi-view,low-resolution
AI 理解论文
溯源树
样例
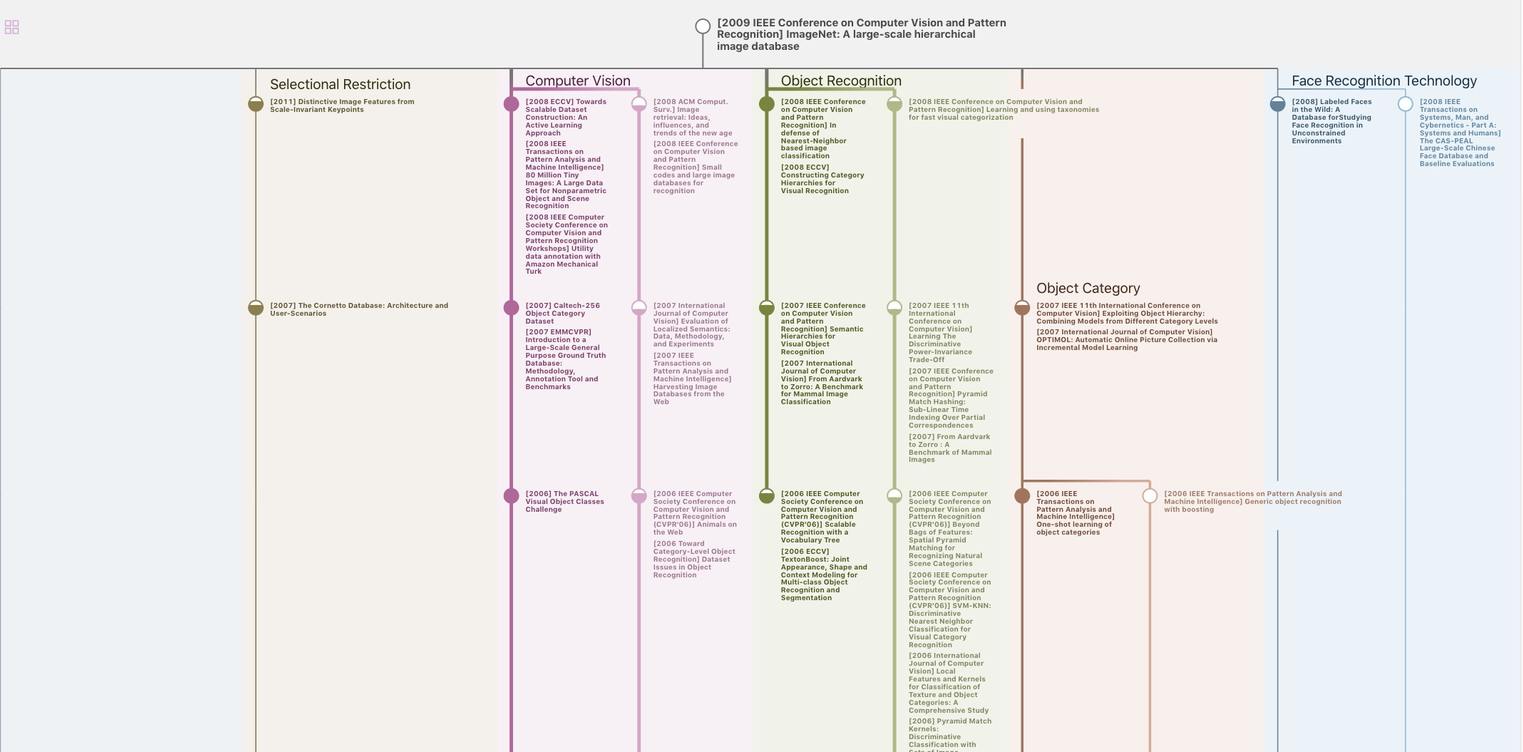
生成溯源树,研究论文发展脉络
Chat Paper
正在生成论文摘要