Label-efficient semantic segmentation of large-scale industrial point clouds using weakly supervised learning
Automation in Construction(2023)
摘要
Semantic segmentation plays an important role in understanding 3D scenes, recognizing objects, and reconstructing 3D models. However, this predominantly requires the training of deep neural networks (DNNs) on large amounts of labeled point clouds, which is time-demanding and expensive. Very recently, label-efficient semantic segmentation also called weakly supervised semantic segmentation (WSSS), is gaining attention, as it opens the way to train DNNs with very limited training labels. However, most of the WSSS focused on learning the labeled data, neglecting to efficiently exploit large amounts of the unlabeled data to learn point cloud features. To fill this gap, this study presents a novel WSSS framework integrated with Self-Training (WSSS-ST) based on a seminal network SQN to fully unleash full potential of the unlabeled data. The proposed WSSS-ST consists of two core components: a WSSS network based on a semantic query net (SQN) and a self-training (ST) module. In the WSSS, a more capable encoder is deployed to obtain more expressive multi-scale hierarchical point embeddings. Furthermore, a new outlier-free interpolation algorithm is devised to acquire queried features for the labeled points. Regarding the ST, a new self-training strategy is harnessed to generate high-quality pseudo-labels to augment the training set, further improving the segmentation performance. To validate the proposed framework, comprehensive experiments are conducted to demonstrate the effectiveness of our WSSS-ST with fully supervised methods and baseline on two large-scale datasets PSNet5 and S3DIS. Surprisingly, the proposed WSSS-ST achieves comparable performance to the fully supervised methods on the PSNet5 with as few as 1% training labels. Besides, it also achieves outstanding performance on the S3DIS. This study contributes to new data-driven approaches for point cloud representation learning, which shall greatly relieve the burden of building labeled datasets for understanding 3D scenes using deep learning.
更多查看译文
关键词
Industrial point clouds,Label-efficient semantic segmentation,Weakly supervised learning,Deep learning,Spatial interpolation
AI 理解论文
溯源树
样例
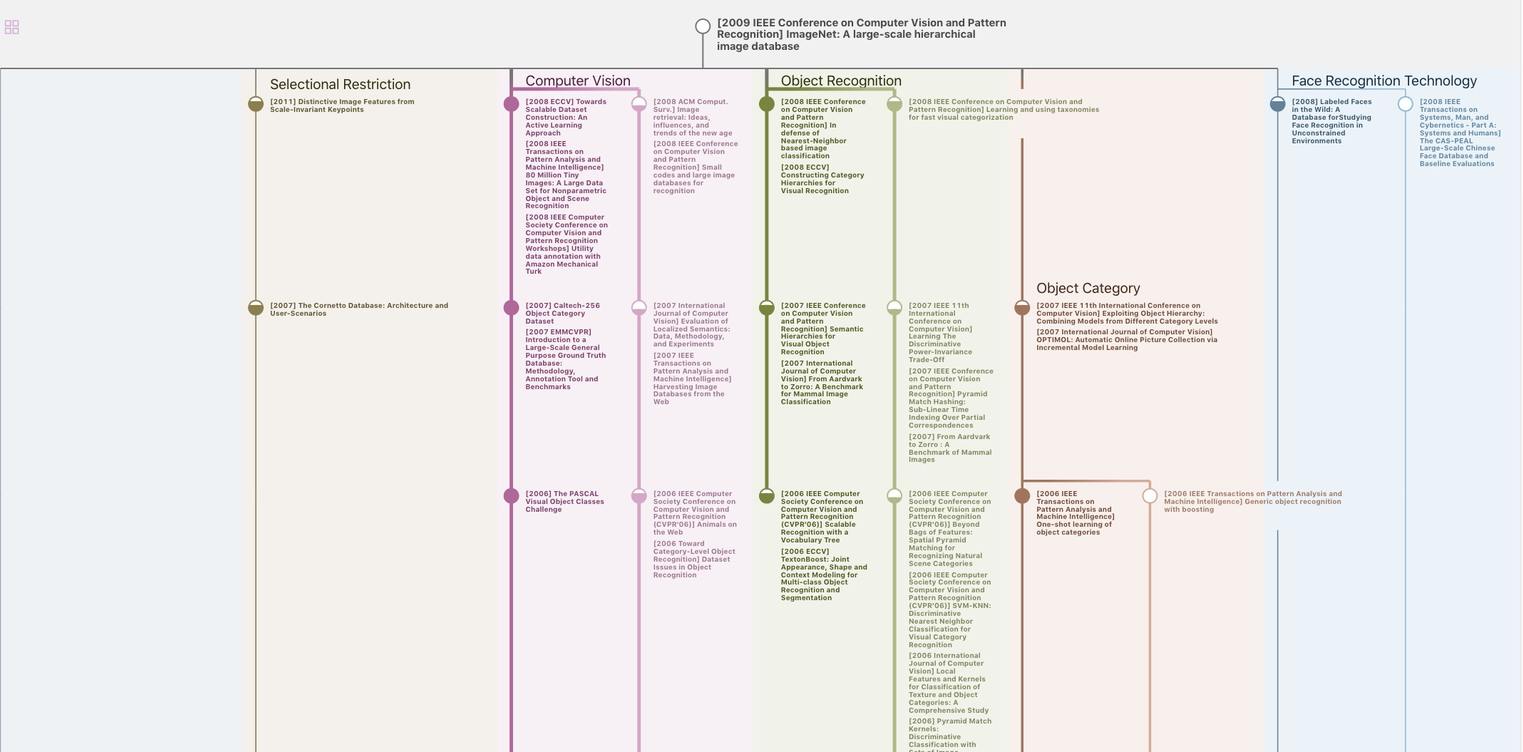
生成溯源树,研究论文发展脉络
Chat Paper
正在生成论文摘要