Cross-Device Federated Learning for Mobile Health Diagnostics: A First Study on COVID-19 Detection
arxiv(2023)
摘要
Federated learning (FL) aided health diagnostic models can incorporate data from a large number of personal edge devices (e.g., mobile phones) while keeping the data local to the originating devices, largely ensuring privacy. However, such a cross-device FL approach for health diagnostics still imposes many challenges due to both local data imbalance (as extreme as local data consists of a single disease class) and global data imbalance (the disease prevalence is generally low in a population). Since the federated server has no access to data distribution information, it is not trivial to solve the imbalance issue towards an unbiased model. In this paper, we propose FedLoss, a novel cross-device FL framework for health diagnostics. Here the federated server averages the models trained on edge devices according to the predictive loss on the local data, rather than using only the number of samples as weights. As the predictive loss better quantifies the data distribution at a device, FedLoss alleviates the impact of data imbalance. Through a real-world dataset on respiratory sound and symptom-based COVID-19 detection task, we validate the superiority of FedLoss. It achieves competitive COVID-19 detection performance compared to a centralised model with an AUC-ROC of 79%. It also outperforms the state-of-the-art FL baselines in sensitivity and convergence speed. Our work not only demonstrates the promise of federated COVID-19 detection but also paves the way to a plethora of mobile health model development in a privacy-preserving fashion.
更多查看译文
关键词
Federated learning,Privacy-preserving,Mobile health,COVID-19 detection,Acoustic modelling
AI 理解论文
溯源树
样例
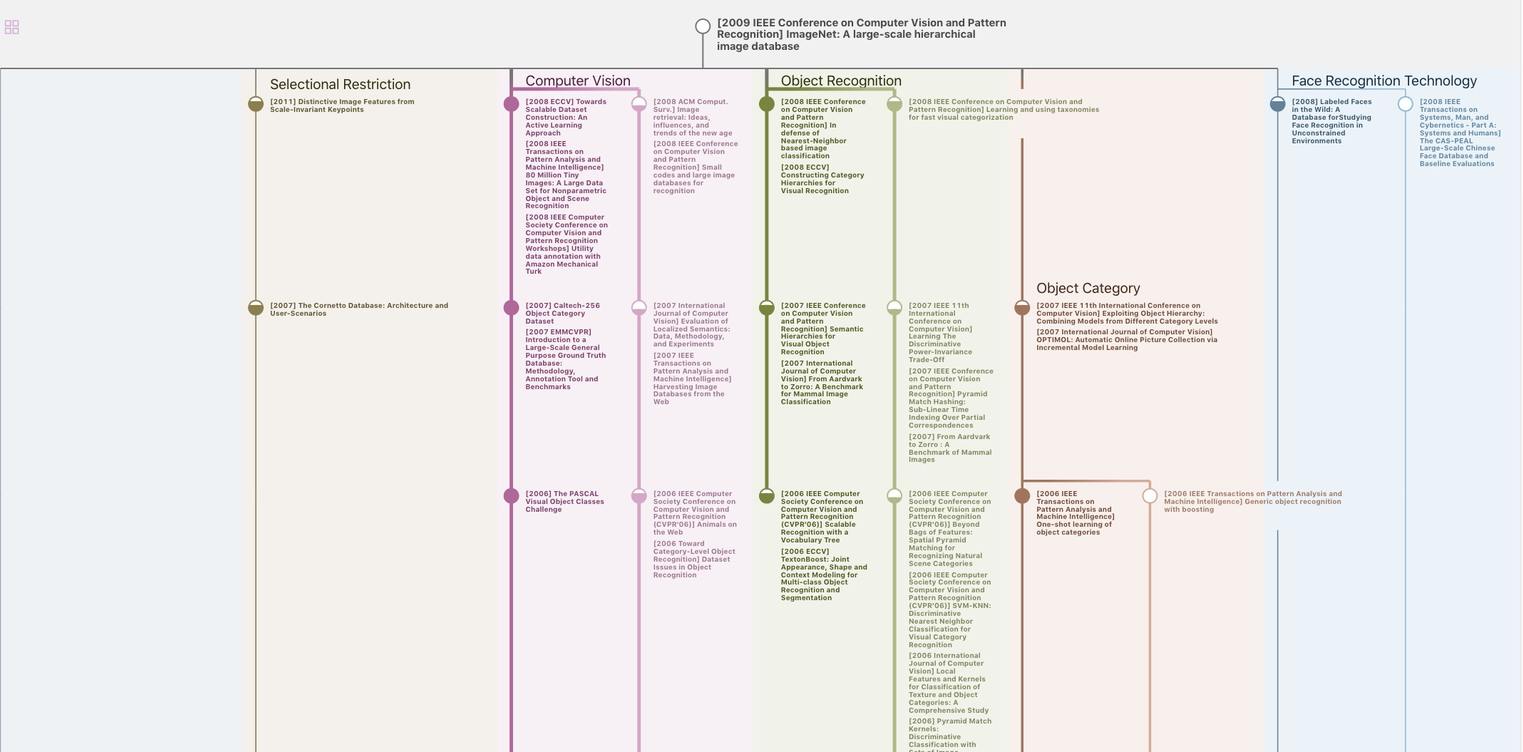
生成溯源树,研究论文发展脉络
Chat Paper
正在生成论文摘要