Bridging the gap between chemical reaction pretraining and conditional molecule generation with a unified model
NATURE MACHINE INTELLIGENCE(2023)
摘要
Chemical reactions are the fundamental building blocks of drug design and organic chemistry research. In recent years, there has been a growing need for a large-scale deep-learning framework that can efficiently capture the basic rules of chemical reactions. In this paper, we have proposed a unified framework that addresses both the reaction-representation learning and molecule generation tasks, which allows for a more holistic approach. Inspired by the organic chemistry mechanism, we develop a new pretraining framework that enables us to incorporate inductive biases into the model. Our framework achieves state-of-the-art results in performance of challenging downstream tasks. By possessing chemical knowledge, our generative framework overcomes the limitations of current molecule generation models that rely on a small number of reaction templates. In extensive experiments, our model generates synthesizable drug-like structures of high quality. Overall, our work presents a noteworthy step toward a large-scale deep-learning framework for a variety of reaction-based applications. Virtual drug design has seen recent progress in methods that can generate new molecules with specific properties. Separately, methods have also improved in the task of computationally predicting the outcome of chemical reactions. Qiang and colleagues use the close relation of the two problems to train a model that aims at solving both tasks.
更多查看译文
关键词
chemical reaction pretraining,conditional molecule generation,unified model
AI 理解论文
溯源树
样例
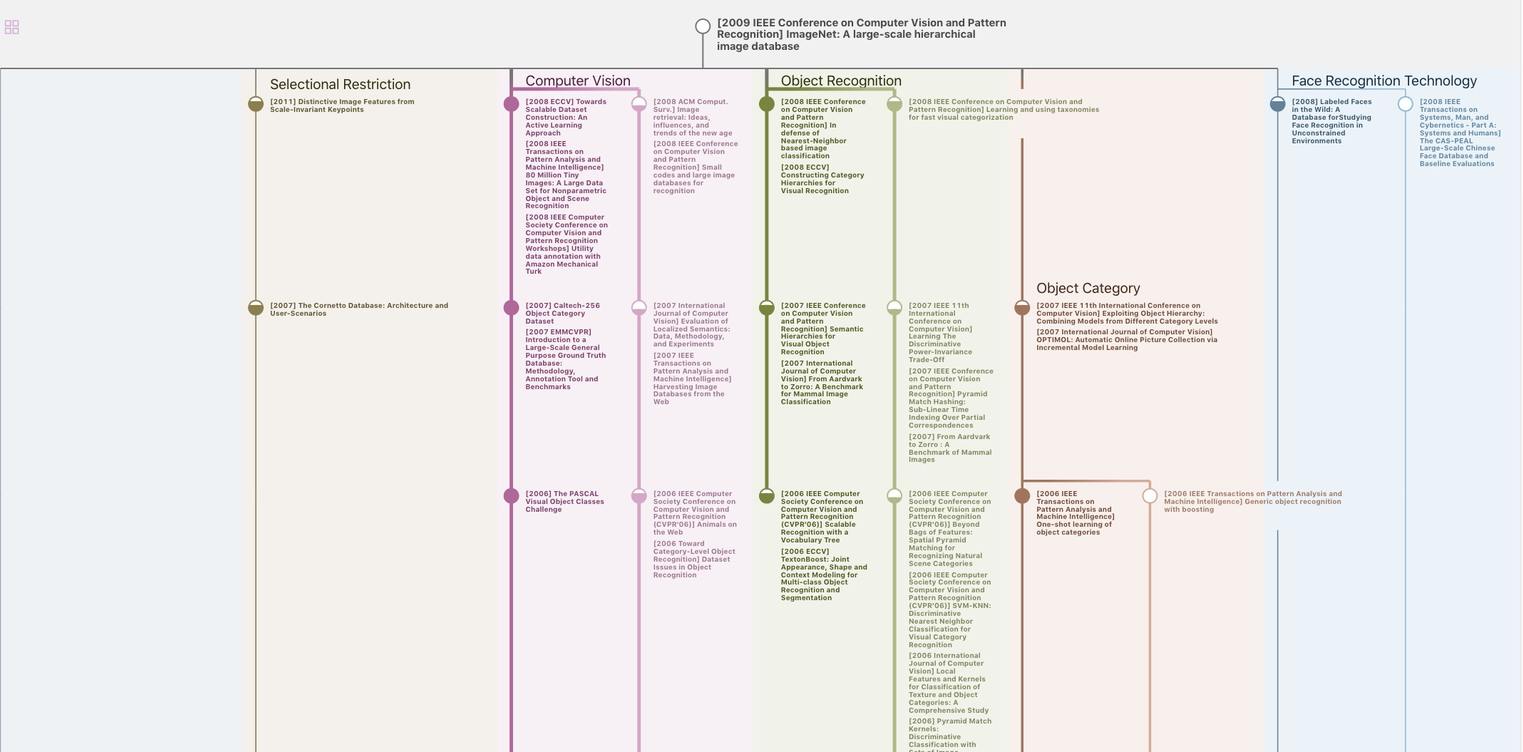
生成溯源树,研究论文发展脉络
Chat Paper
正在生成论文摘要