Leveraging Neural Koopman Operators to Learn Continuous Representations of Dynamical Systems from Scarce Data
arxiv(2023)
摘要
Over the last few years, several works have proposed deep learning architectures to learn dynamical systems from observation data with no or little knowledge of the underlying physics. A line of work relies on learning representations where the dynamics of the underlying phenomenon can be described by a linear operator, based on the Koopman operator theory. However, despite being able to provide reliable long-term predictions for some dynamical systems in ideal situations, the methods proposed so far have limitations, such as requiring to discretize intrinsically continuous dynamical systems, leading to data loss, especially when handling incomplete or sparsely sampled data. Here, we propose a new deep Koopman framework that represents dynamics in an intrinsically continuous way, leading to better performance on limited training data, as exemplified on several datasets arising from dynamical systems.
更多查看译文
关键词
Learning dynamical systems,Koopman operator,Forecasting,Data assimilation,Continuous dynamical systems
AI 理解论文
溯源树
样例
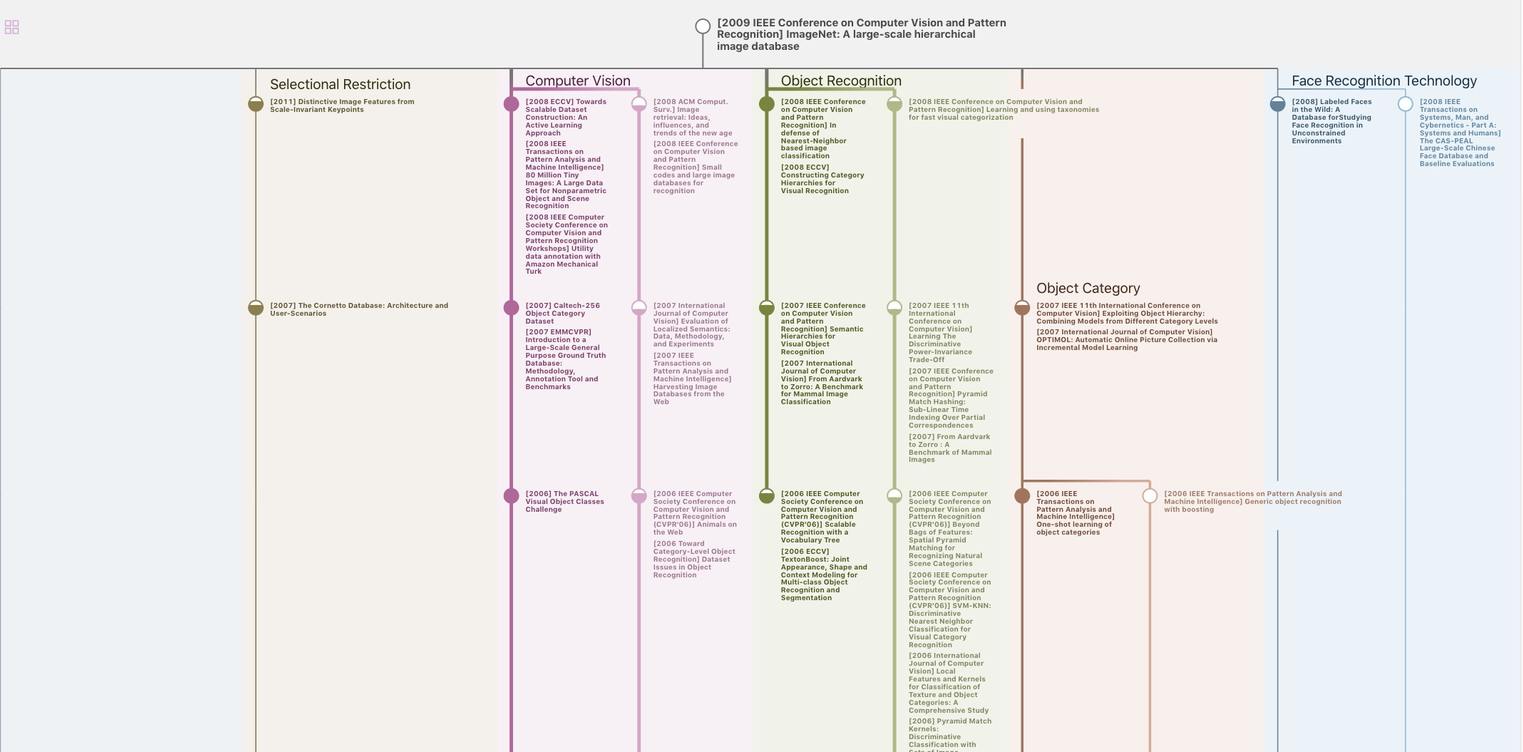
生成溯源树,研究论文发展脉络
Chat Paper
正在生成论文摘要