Improving Table Structure Recognition With Visual-Alignment Sequential Coordinate Modeling
CVPR 2023(2023)
摘要
Table structure recognition aims to extract the logical and physical structure of unstructured table images into a machine-readable format. The latest end-to-end image-to-text approaches simultaneously predict the two structures by two decoders, where the prediction of the physical structure (the bounding boxes of the cells) is based on the representation of the logical structure. However, as the logical representation lacks the local visual information, the previous methods often produce imprecise bounding boxes. To address this issue, we propose an end-to-end sequential modeling framework for table structure recognition called VAST. It contains a novel coordinate sequence decoder triggered by the representation of the non-empty cell from the logical structure decoder. In the coordinate sequence decoder, we model the bounding box coordinates as a language sequence, where the left, top, right and bottom coordinates are decoded sequentially to leverage the inter-coordinate dependency. Furthermore, we propose an auxiliary visual-alignment loss to enforce the logical representation of the non-empty cells to contain more local visual details, which helps produce better cell bounding boxes. Extensive experiments demonstrate that our proposed method can achieve state-of-the-art results in both logical and physical structure recognition. The ablation study also validates that the proposed coordinate sequence decoder and the visual-alignment loss are the keys to the success of our method.
更多查看译文
关键词
table structure recognition,coordinate modeling,visual-alignment
AI 理解论文
溯源树
样例
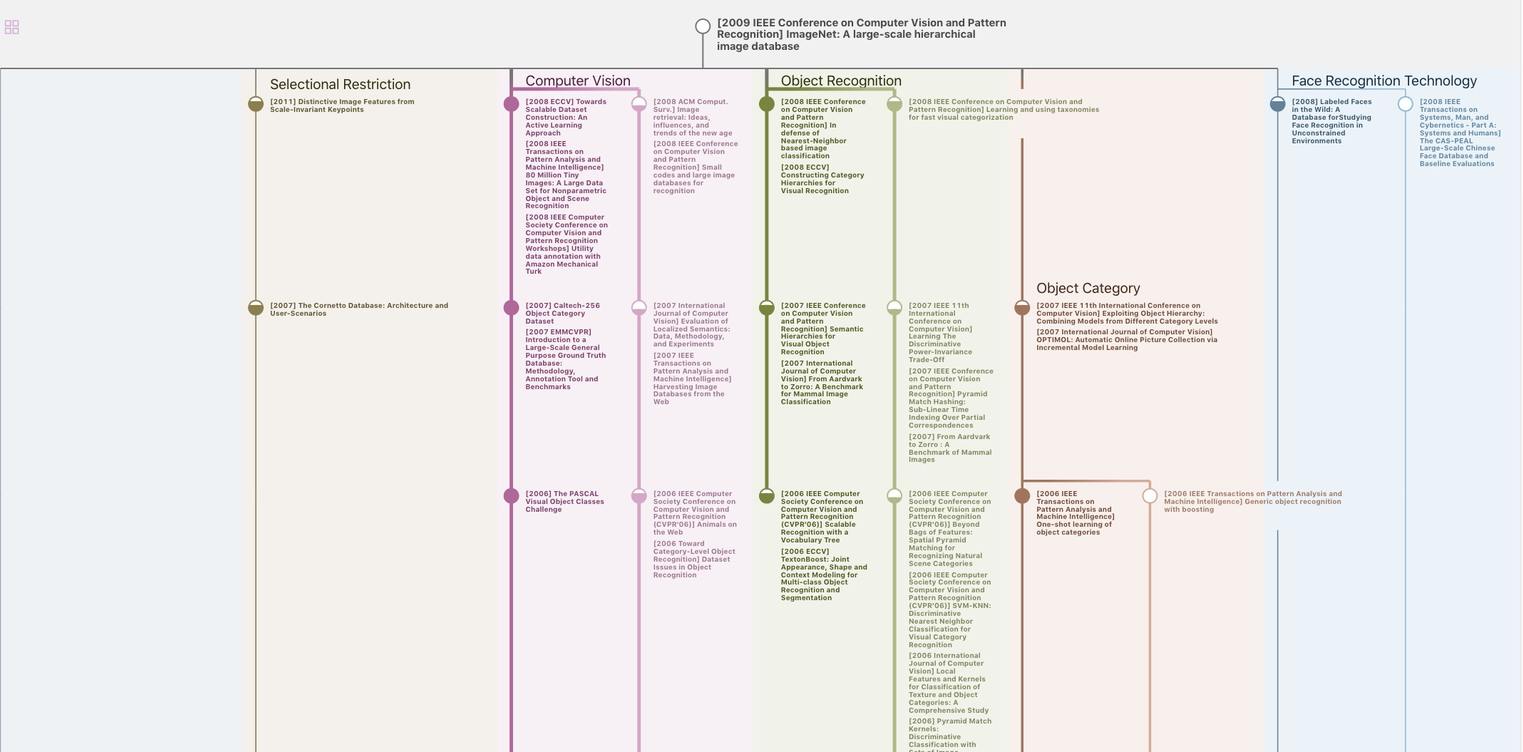
生成溯源树,研究论文发展脉络
Chat Paper
正在生成论文摘要