Nonlinear Consensus for Distributed Optimization
arxiv(2023)
摘要
Distributed optimization algorithms have been studied extensively in the literature; however, underlying most algorithms is a linear consensus scheme, i.e. averaging variables from neighbors via doubly stochastic matrices. We consider nonlinear consensus schemes with a set of time-varying and agent-dependent monotonic Lipschitz nonlinear transformations, where we note the operation is a subprojection onto the consensus plane, which has been identified via stochastic approximation theory. For the proposed nonlinear consensus schemes, we establish convergence when combined with the NEXT algorithm [Lorenzo and Scutari, 2016], and analyze the convergence rate when combined with the DGD algorithm [Nedi\'c and Ozdaglar, 2009]. In addition, we show that convergence can still be guaranteed even though column stochasticity is relaxed for the gossip of the primal variable, and then couple this with nonlinear transformations to show that as a result one can choose any point within the "shrunk cube hull" spanned from neighbors' variables during the consensus step. We perform numerical simulations to demonstrate the different consensus schemes proposed in the paper can outperform the traditional linear scheme.
更多查看译文
关键词
distributed optimization,nonlinear consensus
AI 理解论文
溯源树
样例
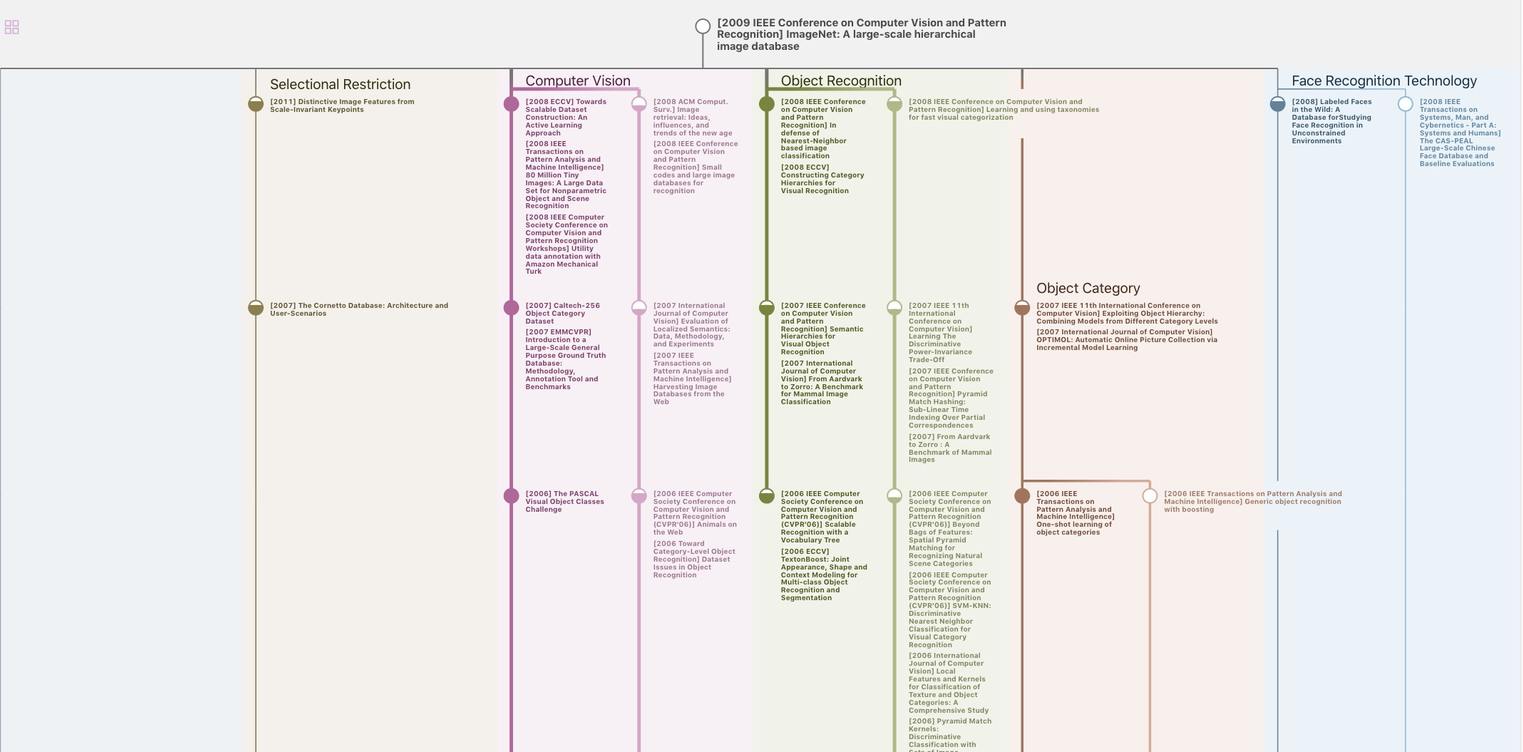
生成溯源树,研究论文发展脉络
Chat Paper
正在生成论文摘要