Non-Trivial Query Sampling For Efficient Learning To Plan
arxiv(2023)
摘要
In recent years, learning-based approaches have revolutionized motion planning. The data generation process for these methods involves caching a large number of high quality paths for different queries (start, goal pairs) in various environments. Conventionally, a uniform random strategy is used for sampling these queries. However, this leads to inclusion of "trivial paths" in the dataset (e.g.,, straight line paths in case of length-optimal planning), which can be solved efficiently if the planner has access to a steering function. This work proposes a "non-trivial" query sampling procedure to add more complex paths in the dataset. Numerical experiments show that a higher success rate can be attained for neural planners trained on such a non-trivial dataset.
更多查看译文
关键词
efficient learning,plan,non-trivial
AI 理解论文
溯源树
样例
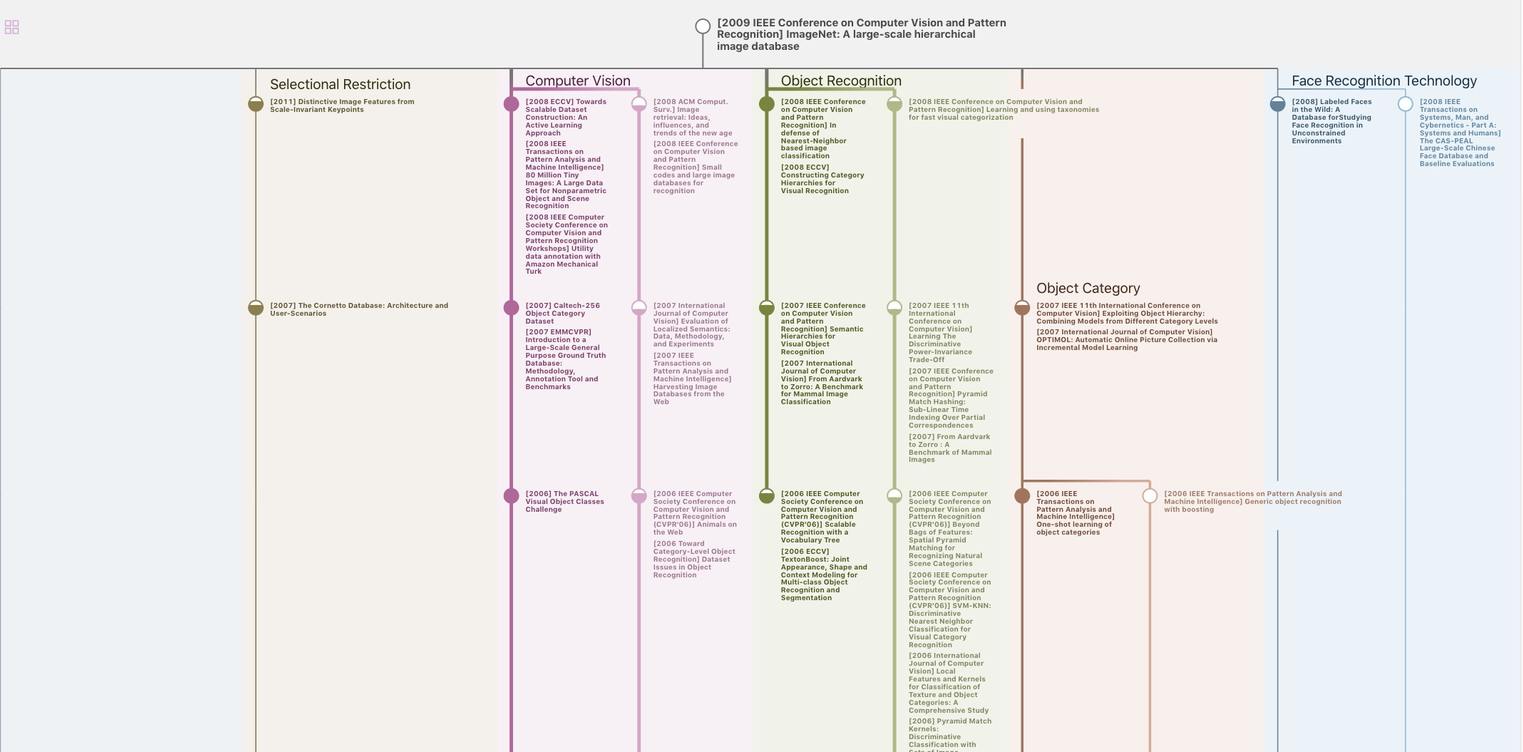
生成溯源树,研究论文发展脉络
Chat Paper
正在生成论文摘要