LUKE-Graph: A Transformer-based Approach with Gated Relational Graph Attention for Cloze-style Reading Comprehension
arxiv(2023)
摘要
Incorporating prior knowledge has been identified as a promising approach to enhance existing pre-training models in cloze-style machine reading, as observed in recent studies. Despite the use of external knowledge graphs (KG) and transformer-based models like BERT in most existing models, the identification of the most pertinent ambiguous entities in KG, as well as the extraction of the optimal subgraphs, remains problematic. To address these challenges, we introduce the LUKE-Graph model, which constructs a heterogeneous graph based on the intuitive relationships between entities in the documents without relying on external KGs. We then employ a Relational Graph Attention (RGAT) network to combine the reasoning information of the graph with the contextual representation generated by the pre-trained LUKE model. In this way, we can take advantage of LUKE, to derive an entity-aware representation; and a graph model - to exploit relation-aware representation. Furthermore, we present Gated-RGAT, an enhancement to RGAT that incorporates a gating mechanism to control the question information during the graph convolution operation. This mechanism emulates the human reasoning process in selecting the most suitable entity candidate based on question information. Our experimental results demonstrate that the proposed LUKE-Graph model surpasses the LUKE state-of-the-art model on the ReCoRD dataset, which focuses on commonsense reasoning, and the WikiHop dataset, which centers on multi-hop reasoning problems.
更多查看译文
关键词
Transformer-based model,Gated relational graph attention model,Cloze-style machine reading comprehension,Question answering,LUKE,Commonsense reasoning
AI 理解论文
溯源树
样例
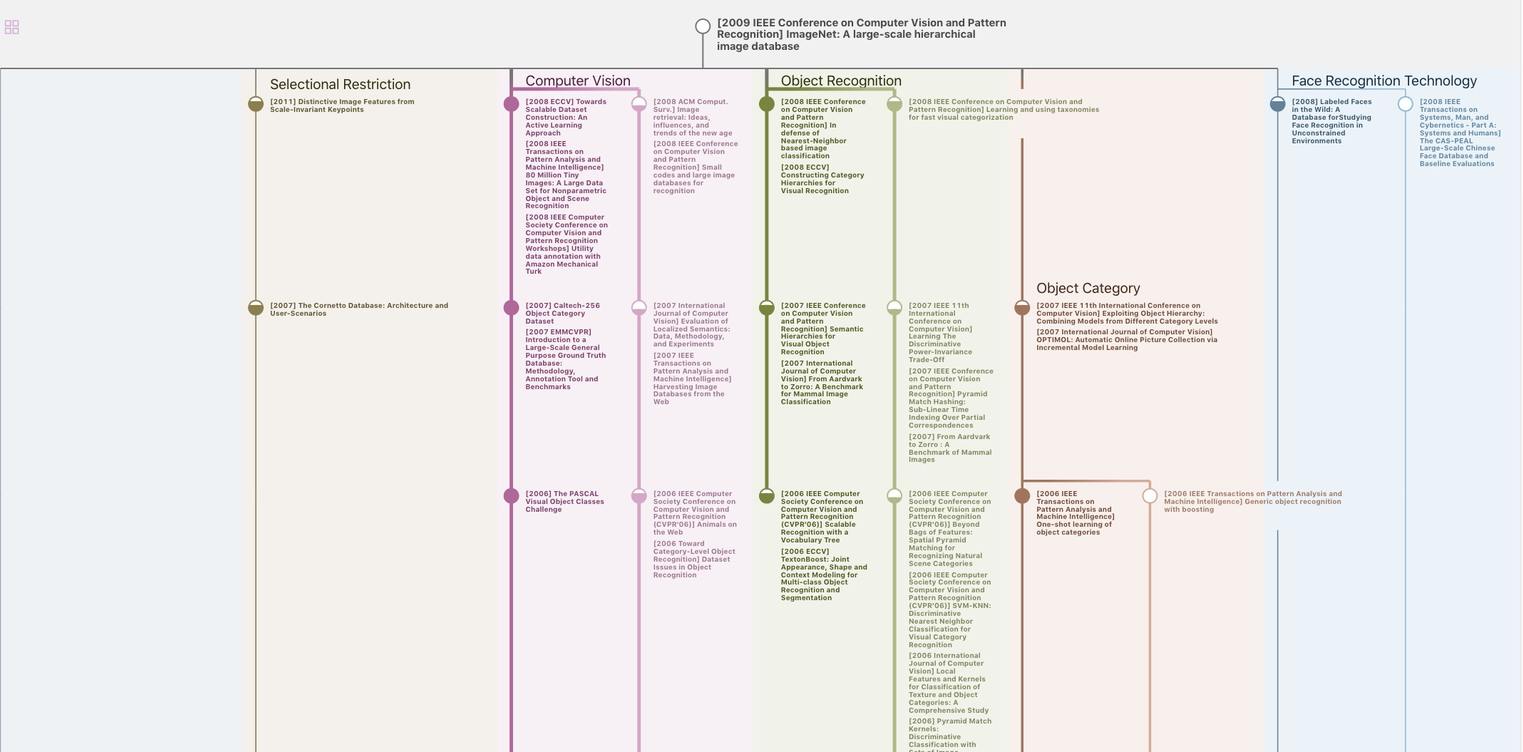
生成溯源树,研究论文发展脉络
Chat Paper
正在生成论文摘要