Learning Deep Intensity Field for Extremely Sparse-View CBCT Reconstruction
MEDICAL IMAGE COMPUTING AND COMPUTER ASSISTED INTERVENTION, MICCAI 2023, PT X(2023)
摘要
Sparse-view cone-beam CT (CBCT) reconstruction is an important direction to reduce radiation dose and benefit clinical applications. Previous voxel-based generation methods represent the CT as discrete voxels, resulting in high memory requirements and limited spatial resolution due to the use of 3D decoders. In this paper, we formulate the CT volume as a continuous intensity field and develop a novel DIF-Net to perform high-quality CBCT reconstruction from extremely sparse (<= 10) projection views at an ultrafast speed. The intensity field of a CT can be regarded as a continuous function of 3D spatial points. Therefore, the reconstruction can be reformulated as regressing the intensity value of an arbitrary 3D point from given sparse projections. Specifically, for a point, DIF-Net extracts its view-specific features from different 2D projection views. These features are subsequently aggregated by a fusion module for intensity estimation. Notably, thousands of points can be processed in parallel to improve efficiency during training and testing. In practice, we collect a knee CBCT dataset to train and evaluate DIF-Net. Extensive experiments show that our approach can reconstruct CBCT with high image quality and high spatial resolution from extremely sparse views within 1.6 s, significantly outperforming state-of-the-art methods. Our code will be available at https://github.com/xmed-lab/DIF-Net.
更多查看译文
关键词
CBCT Reconstruction,Implicit Neural Representation,Sparse View,Low Dose,Efficient Reconstruction
AI 理解论文
溯源树
样例
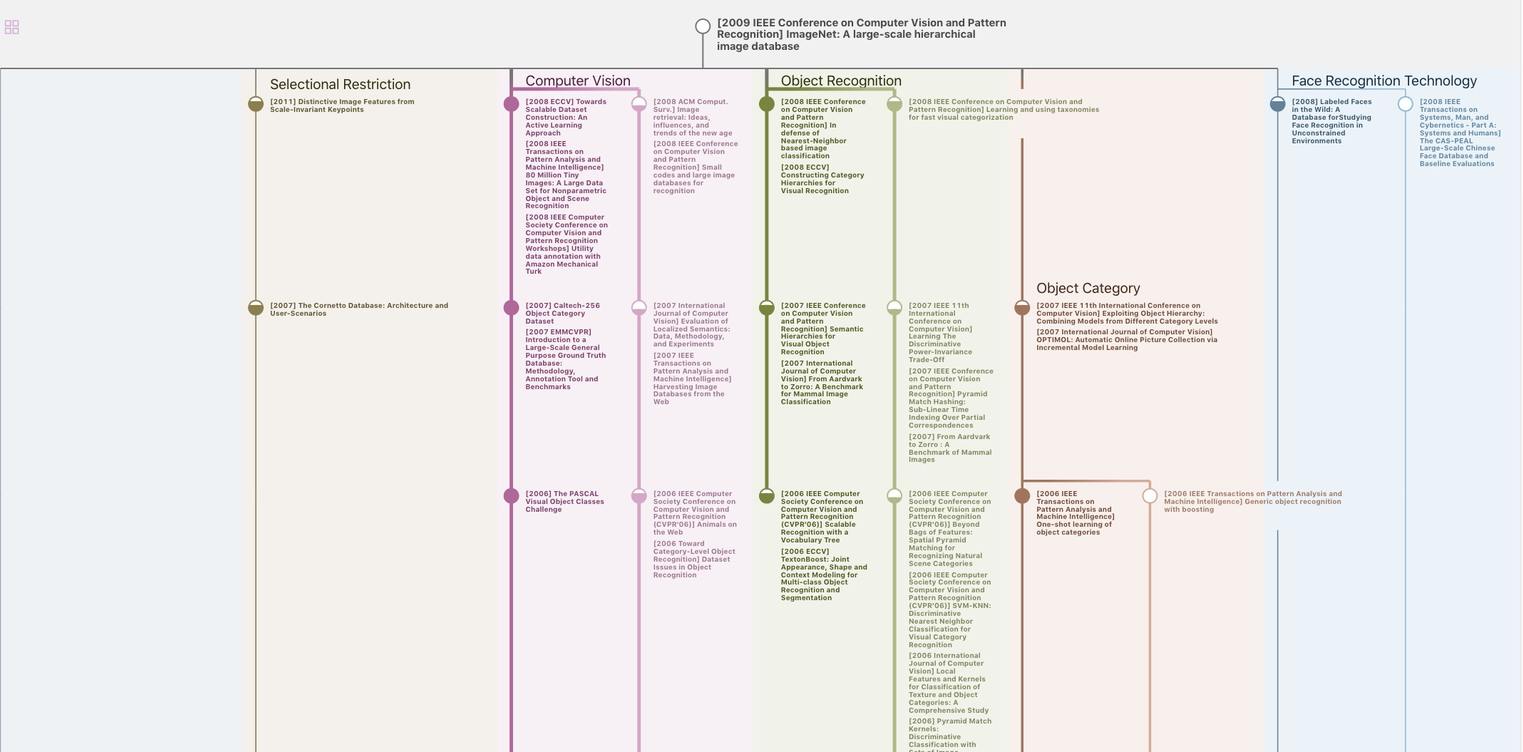
生成溯源树,研究论文发展脉络
Chat Paper
正在生成论文摘要