Improving the Intent Classification accuracy in Noisy Environment
arxiv(2023)
摘要
Intent classification is a fundamental task in the spoken language understanding field that has recently gained the attention of the scientific community, mainly because of the feasibility of approaching it with end-to-end neural models. In this way, avoiding using intermediate steps, i.e. automatic speech recognition, is possible, thus the propagation of errors due to background noise, spontaneous speech, speaking styles of users, etc. Towards the development of solutions applicable in real scenarios, it is interesting to investigate how environmental noise and related noise reduction techniques to address the intent classification task with end-to-end neural models. In this paper, we experiment with a noisy version of the fluent speech command data set, combining the intent classifier with a time-domain speech enhancement solution based on Wave-U-Net and considering different training strategies. Experimental results reveal that, for this task, the use of speech enhancement greatly improves the classification accuracy in noisy conditions, in particular when the classification model is trained on enhanced signals.
更多查看译文
关键词
intent classification accuracy,noisy environment
AI 理解论文
溯源树
样例
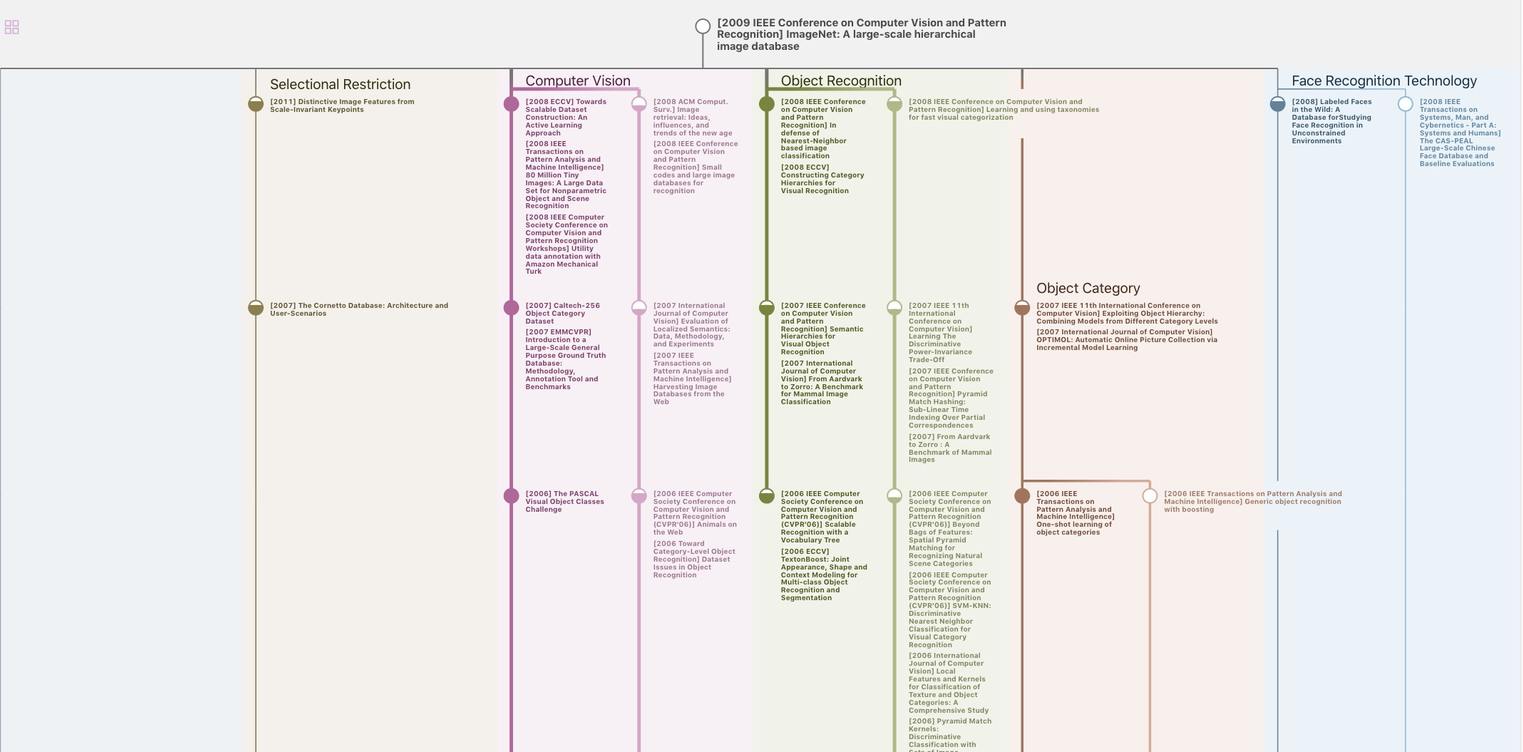
生成溯源树,研究论文发展脉络
Chat Paper
正在生成论文摘要