Scope and Arbitration in Machine Learning Clinical EEG Classification
arxiv(2023)
摘要
A key task in clinical EEG interpretation is to classify a recording or session as normal or abnormal. In machine learning approaches to this task, recordings are typically divided into shorter windows for practical reasons, and these windows inherit the label of their parent recording. We hypothesised that window labels derived in this manner can be misleading for example, windows without evident abnormalities can be labelled `abnormal' disrupting the learning process and degrading performance. We explored two separable approaches to mitigate this problem: increasing the window length and introducing a second-stage model to arbitrate between the window-specific predictions within a recording. Evaluating these methods on the Temple University Hospital Abnormal EEG Corpus, we significantly improved state-of-the-art average accuracy from 89.8 percent to 93.3 percent. This result defies previous estimates of the upper limit for performance on this dataset and represents a major step towards clinical translation of machine learning approaches to this problem.
更多查看译文
关键词
classification,machine learning,arbitration,scope
AI 理解论文
溯源树
样例
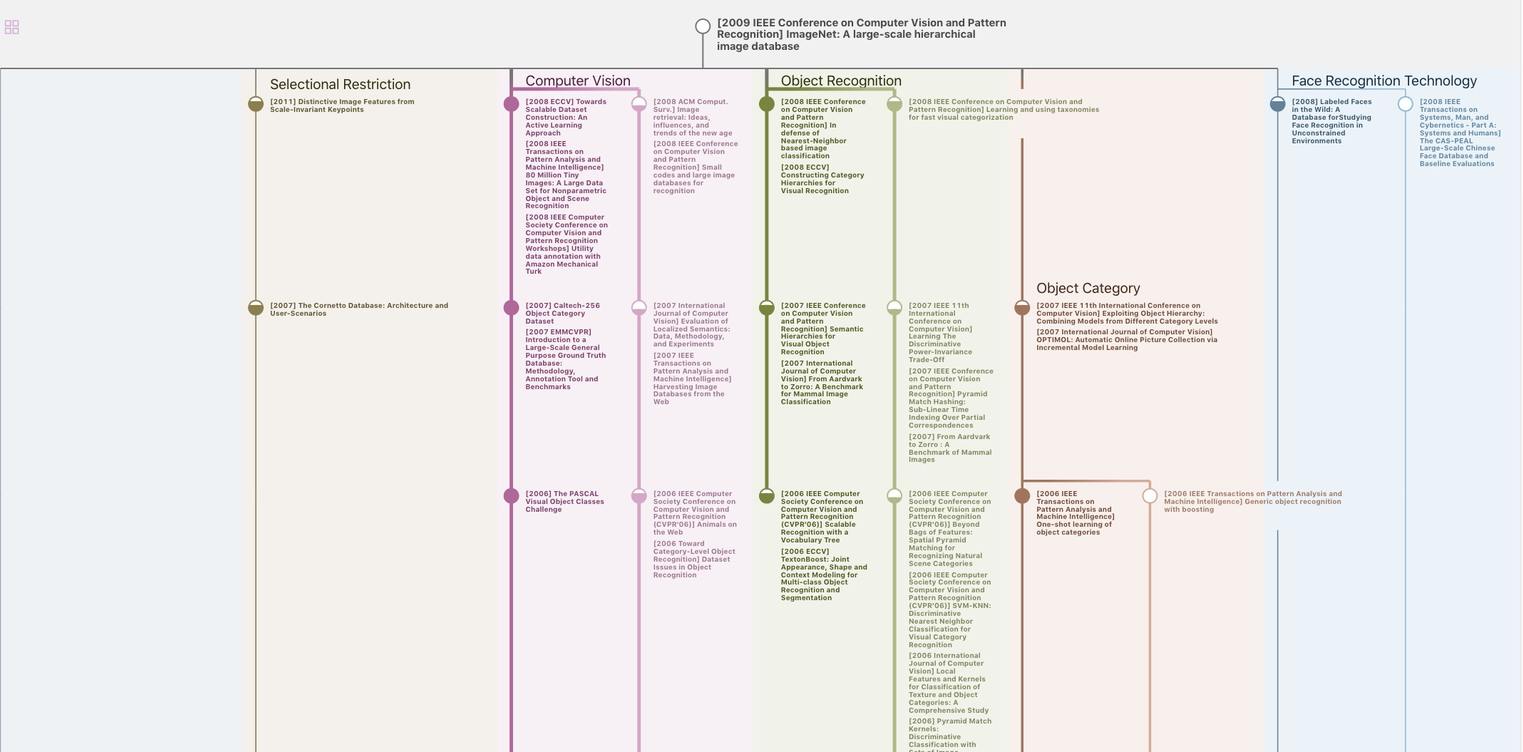
生成溯源树,研究论文发展脉络
Chat Paper
正在生成论文摘要