Sem-cs: semantic clipstyler for text-based image style transfer
2023 IEEE INTERNATIONAL CONFERENCE ON IMAGE PROCESSING, ICIP(2023)
摘要
CLIPStyler demonstrated image style transfer with realistic textures using only a style text description (instead of requiring a reference style image). However, the ground semantics of objects in the style transfer output is lost due to style spillover on salient and background objects (content mismatch) or over-stylization. To solve this, we propose Semantic CLIPStyler (Sem-CS), that performs semantic style transfer. Sem-CS first segments the content image into salient and non-salient objects and then transfers artistic style based on a given style text description. The semantic style transfer is achieved using global foreground loss (for salient objects) and global background loss (for non-salient objects). Our empirical results, including DISTS, NIMA and user study scores, show that our proposed framework yields superior qualitative and quantitative performance. Our code is available at github.com/chandagrover/sem-cs.
更多查看译文
关键词
Object detection,Salient,CLIP,Style Transfer,Semantics
AI 理解论文
溯源树
样例
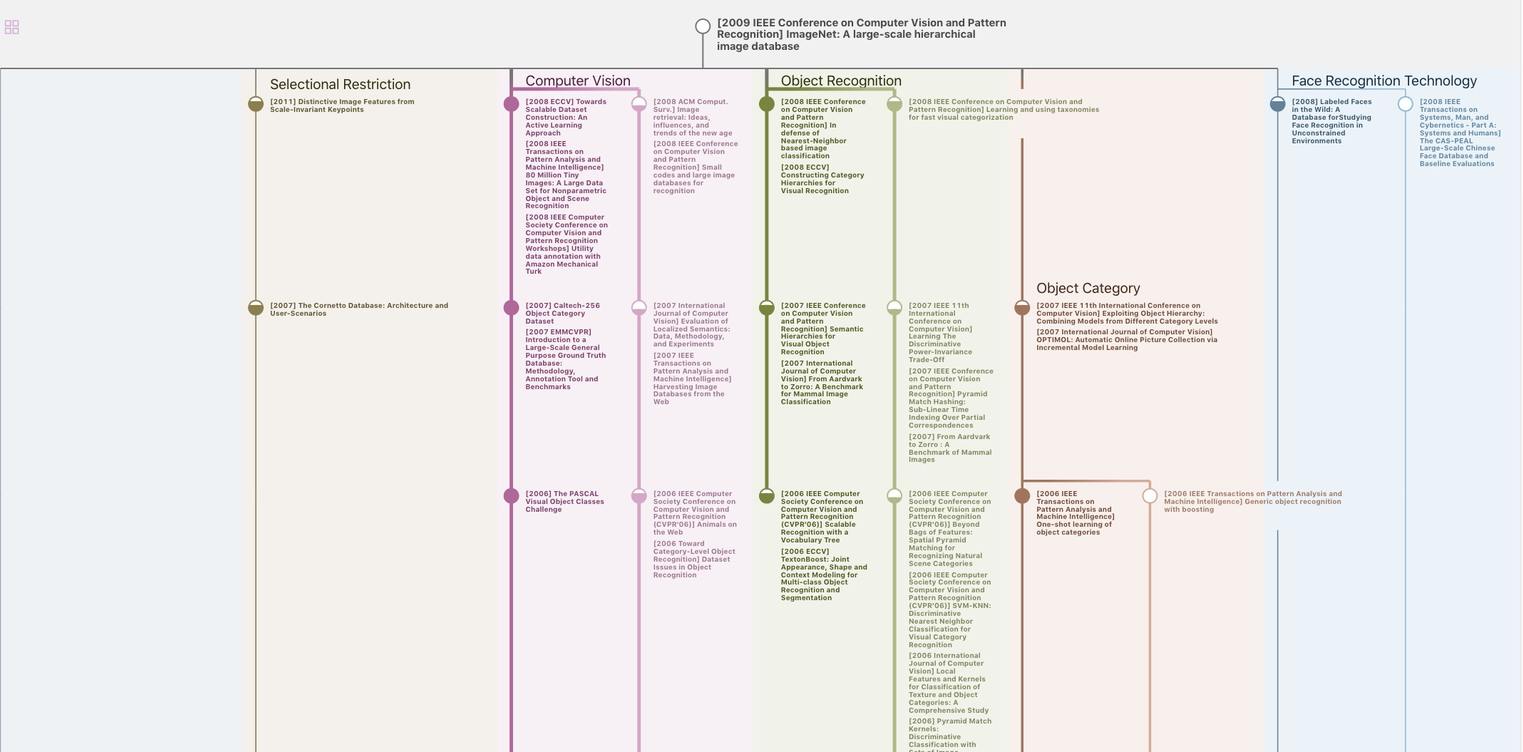
生成溯源树,研究论文发展脉络
Chat Paper
正在生成论文摘要