Machine Learning Enhanced Hankel Dynamic-Mode Decomposition
arxiv(2023)
摘要
While the acquisition of time series has become more straightforward, developing dynamical models from time series is still a challenging and evolving problem domain. Within the last several years, to address this problem, there has been a merging of machine learning tools with what is called the dynamic mode decomposition (DMD). This general approach has been shown to be an especially promising avenue for accurate model development. Building on this prior body of work, we develop a deep learning DMD based method which makes use of the fundamental insight of Takens' Embedding Theorem to build an adaptive learning scheme that better approximates higher dimensional and chaotic dynamics. We call this method the Deep Learning Hankel DMD (DLHDMD). We likewise explore how our method learns mappings which tend, after successful training, to significantly change the mutual information between dimensions in the dynamics. This appears to be a key feature in enhancing the DMD overall, and it should help provide further insight for developing other deep learning methods for time series analysis and model generation.
更多查看译文
关键词
machine learning,dynamic-mode
AI 理解论文
溯源树
样例
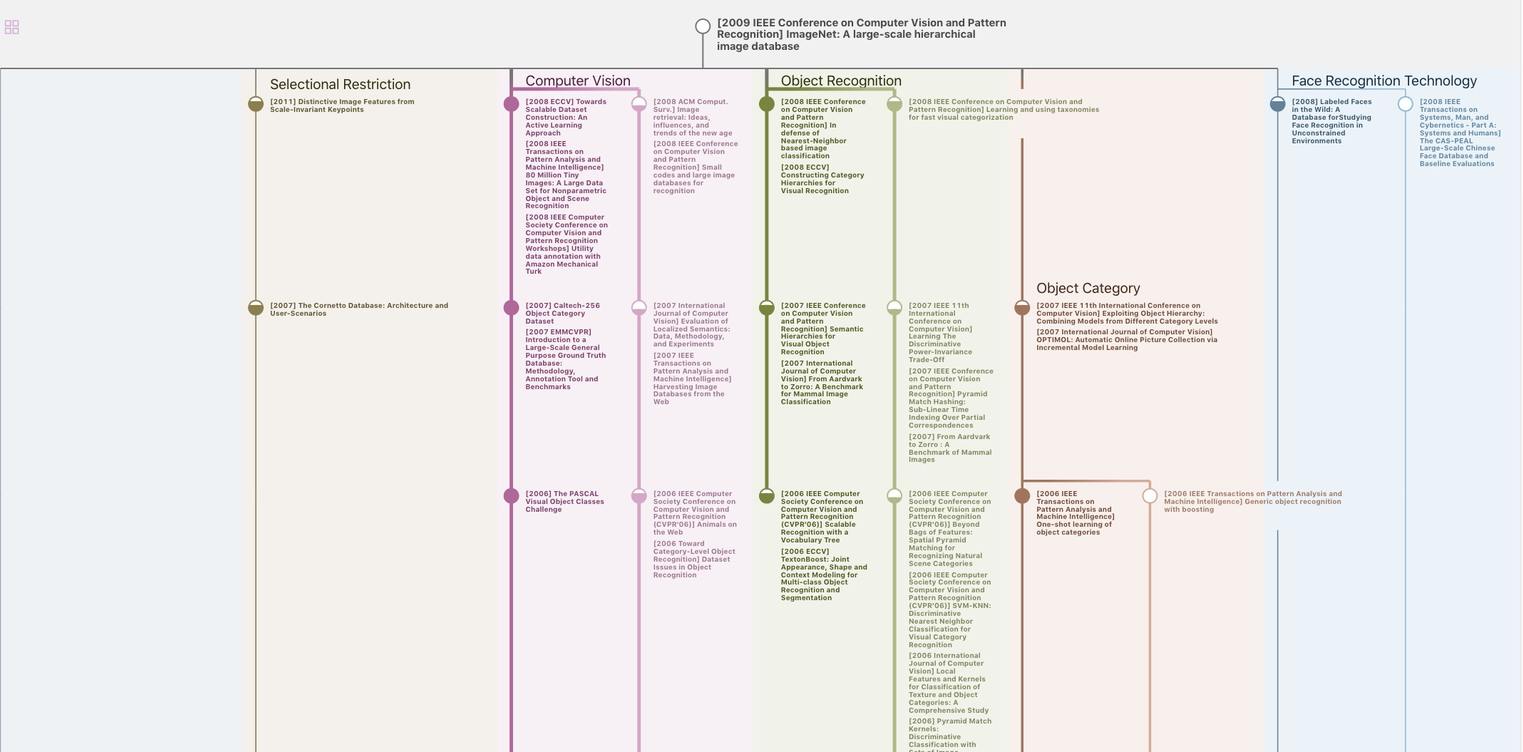
生成溯源树,研究论文发展脉络
Chat Paper
正在生成论文摘要