Optimizing Federated Learning for Medical Image Classification on Distributed Non-iid Datasets with Partial Labels
arxiv(2023)
摘要
Numerous large-scale chest x-ray datasets have spearheaded expert-level detection of abnormalities using deep learning. However, these datasets focus on detecting a subset of disease labels that could be present, thus making them distributed and non-iid with partial labels. Recent literature has indicated the impact of batch normalization layers on the convergence of federated learning due to domain shift associated with non-iid data with partial labels. To that end, we propose FedFBN, a federated learning framework that draws inspiration from transfer learning by using pretrained networks as the model backend and freezing the batch normalization layers throughout the training process. We evaluate FedFBN with current FL strategies using synthetic iid toy datasets and large-scale non-iid datasets across scenarios with partial and complete labels. Our results demonstrate that FedFBN outperforms current aggregation strategies for training global models using distributed and non-iid data with partial labels.
更多查看译文
关键词
federated learning,medical image classification,datasets,non-iid
AI 理解论文
溯源树
样例
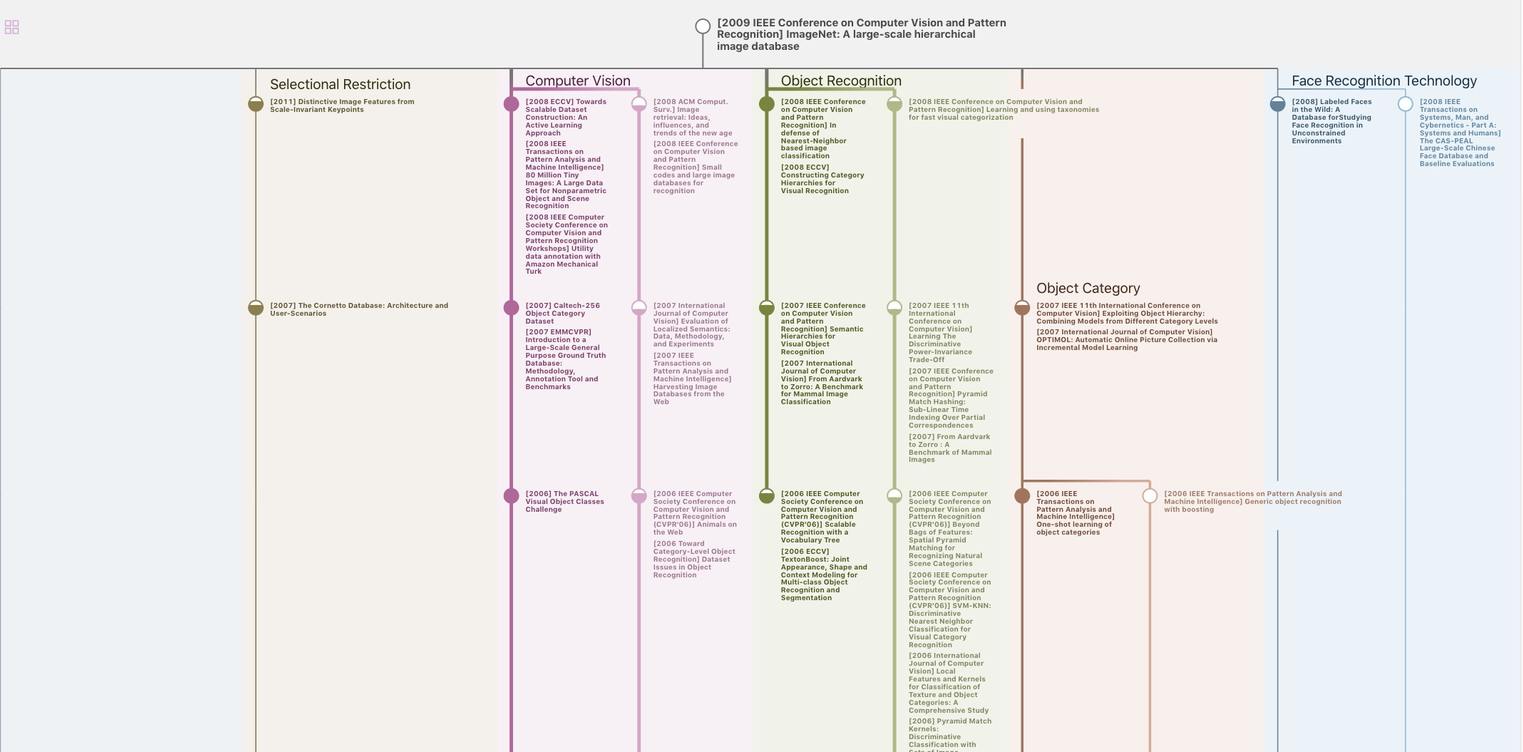
生成溯源树,研究论文发展脉络
Chat Paper
正在生成论文摘要