VALERIAN: Invariant Feature Learning for IMU Sensor-based Human Activity Recognition in the Wild
PROCEEDINGS 8TH ACM/IEEE CONFERENCE ON INTERNET OF THINGS DESIGN AND IMPLEMENTATION, IOTDI 2023(2023)
摘要
Deep neural network models for IMU sensor-based human activity recognition (HAR) that are trained from controlled, well-curated datasets suffer from poor generalizability in practical deployments. However, data collected from naturalistic settings often contains significant label noise. In this work, we examine two in-the-wild HAR datasets and DivideMix, a state-of-the-art learning with noise labels (LNL) method to understand the extent and impacts of noisy labels in training data. Our empirical analysis reveals that the substantial domain gaps among diverse subjects cause LNL methods to violate a key underlying assumption, namely, neural networks tend to fit simpler (and thus clean) data in early training epochs. Motivated by the insights, we design VALERIAN, an invariant feature learning method for in-the-wild wearable sensor-based HAR. By training a multi-task model with separate task-specific layers for each subject, VALERIAN allows noisy labels to be dealt with individually while benefiting from shared feature representation across subjects. We evaluated VALERIAN on four datasets, two collected in a controlled environment and two in the wild. Experimental results show that VALERIAN significantly outperforms baseline approaches. VALERIAN can correct 75% - 93% of label errors in the source domains. When only 10-second clean labeled data per class is available from a new target subject, even with 40% label noise in training data, it achieves similar to 83% test accuracy. Code is available at: https://github.com/YujiaoHao/VALERIAN.git
更多查看译文
关键词
IMU,multi-task learning,human activity recognition,domain adaptation,learning with noisy labels
AI 理解论文
溯源树
样例
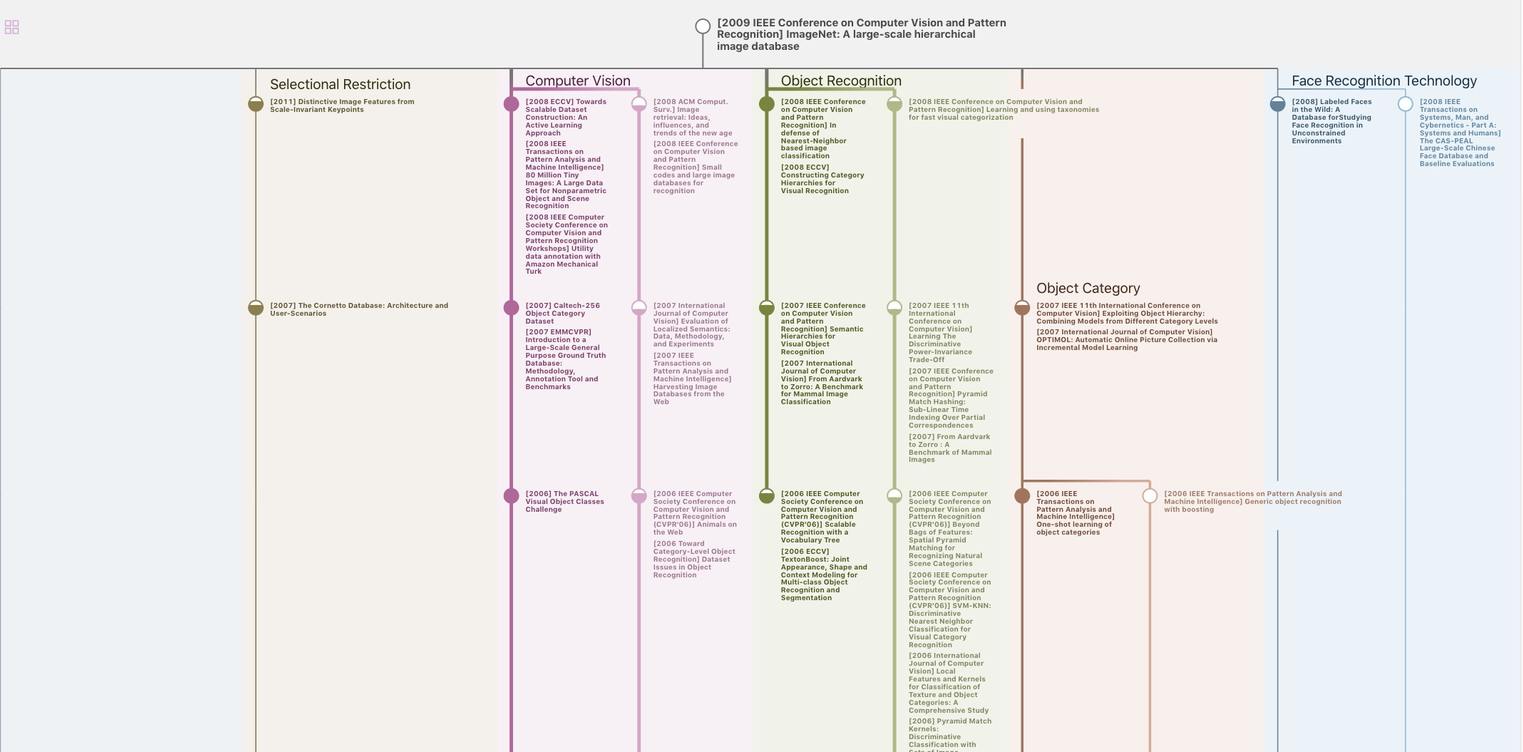
生成溯源树,研究论文发展脉络
Chat Paper
正在生成论文摘要