Data-Driven Machine Learning Calibration Propagation in A Hybrid Sensor Network for Air Quality Monitoring.
Sensors (Basel, Switzerland)(2023)
摘要
Public air quality monitoring relies on expensive monitoring stations which are highly reliable and accurate but require significant maintenance and cannot be used to form a high spatial resolution measurement grid. Recent technological advances have enabled air quality monitoring that uses low-cost sensors. Being inexpensive and mobile, with wireless transfer support, such devices represent a very promising solution for hybrid sensor networks comprising public monitoring stations supported by many low-cost devices for complementary measurements. However, low-cost sensors can be influenced by weather and degradation, and considering that a spatially dense network would include them in large numbers, logistically adept solutions for low-cost device calibration are essential. In this paper, we investigate the possibility of a data-driven machine learning calibration propagation in a hybrid sensor network consisting of One public monitoring station and ten low-cost devices equipped with NO, PM, relative humidity, and temperature sensors. Our proposed solution relies on calibration propagation through a network of low-cost devices where a calibrated low-cost device is used to calibrate an uncalibrated device. This method has shown an improvement of up to 0.35/0.14 for the Pearson correlation coefficient and a reduction of 6.82 µg/m/20.56 µg/m for the RMSE, for NO and PM, respectively, showing promise for efficient and inexpensive hybrid sensor air quality monitoring deployments.
更多查看译文
关键词
air pollution monitoring,air quality,calibration propagation,hybrid network,low-cost sensors,machine learning,sensor calibration
AI 理解论文
溯源树
样例
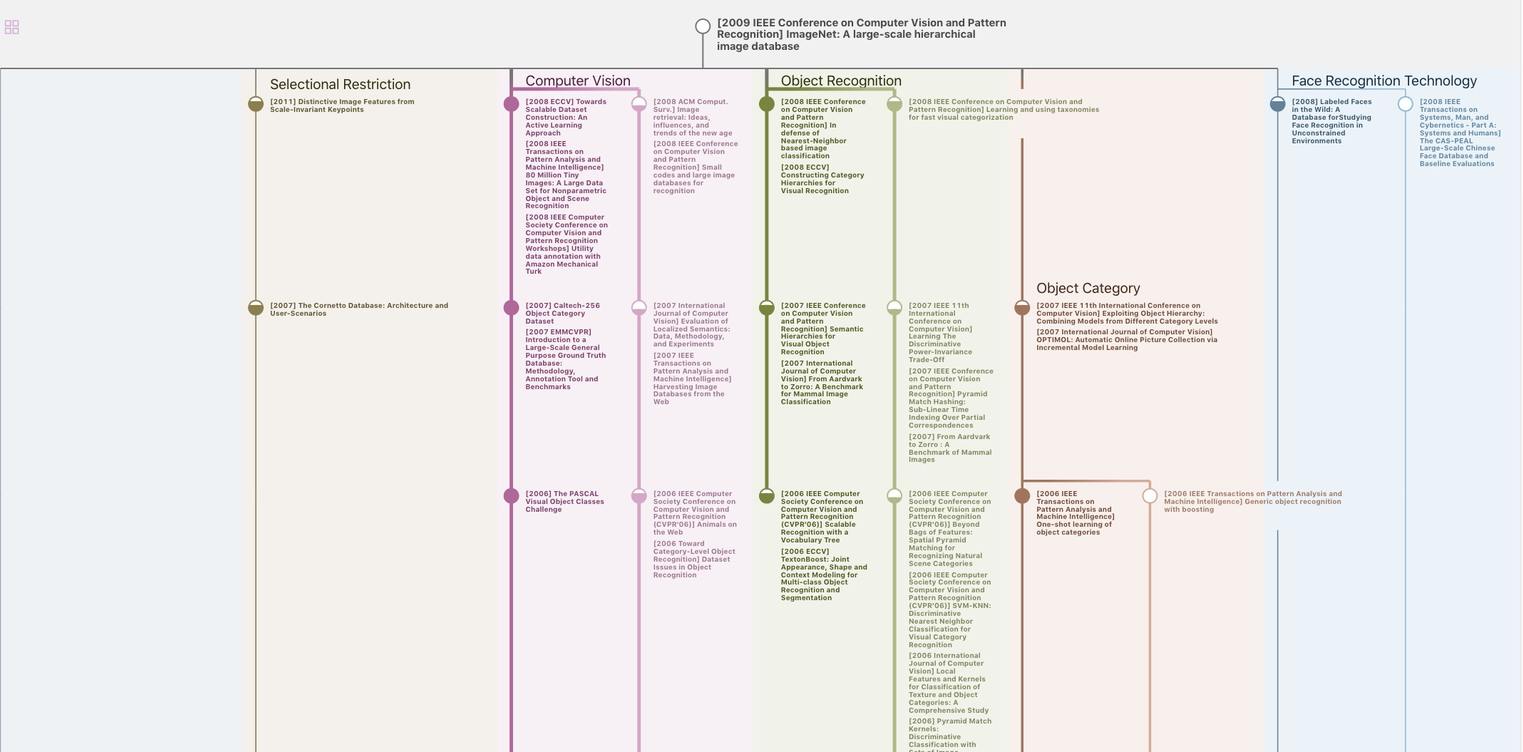
生成溯源树,研究论文发展脉络
Chat Paper
正在生成论文摘要