Automatic Code Review by Learning the Structure Information of Code Graph.
Sensors (Basel, Switzerland)(2023)
摘要
At present, the explosive growth of software code volume and quantity makes the code review process very labor-intensive and time-consuming. An automated code review model can assist in improving the efficiency of the process. Tufano et al., designed two automated tasks to help improve the efficiency of code review based on the deep learning approach, from two different perspectives, namely, the developer submitting the code and the code reviewer. However, they only used code sequence information and did not explore the logical structure information with a richer meaning of the code. To improve the learning of code structure information, a program dependency graph serialization algorithm PDG2Seq algorithm is proposed, which converts the program dependency graph into a unique graph code sequence in a lossless manner, while retaining the program structure information and semantic information. We then designed an automated code review model based on the pre-trained model CodeBERT architecture, which strengthens the learning of code information by fusing program structure information and code sequence information, and then fine-tuned the model according to the code review activity scene to complete the automatic modification of the code. To verify the efficiency of the algorithm, the two tasks in the experiment were compared with the best Algorithm 1-encoder/2-encoder. The experimental results show that the model we proposed has a significant improvement under the BLEU, Lewinshtein distance and ROUGE-L metrics.
更多查看译文
关键词
code review,program dependency graph,deep learning,CodeBERT
AI 理解论文
溯源树
样例
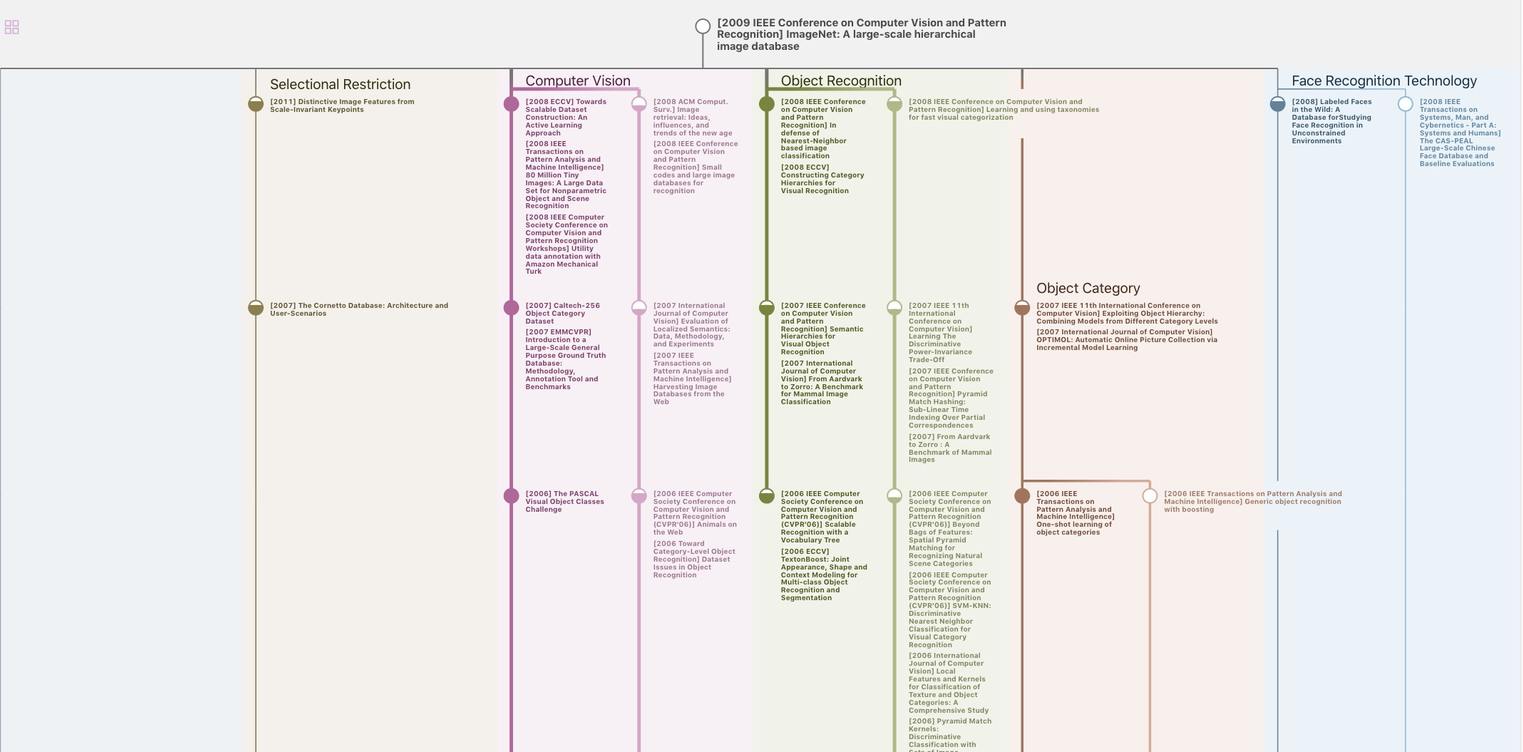
生成溯源树,研究论文发展脉络
Chat Paper
正在生成论文摘要