A Bimodal Emotion Recognition Approach through the Fusion of Electroencephalography and Facial Sequences.
Diagnostics (Basel, Switzerland)(2023)
摘要
In recent years, human-computer interaction (HCI) systems have become increasingly popular. Some of these systems demand particular approaches for discriminating actual emotions through the use of better multimodal methods. In this work, a deep canonical correlation analysis (DCCA) based multimodal emotion recognition method is presented through the fusion of electroencephalography (EEG) and facial video clips. A two-stage framework is implemented, where the first stage extracts relevant features for emotion recognition using a single modality, while the second stage merges the highly correlated features from the two modalities and performs classification. Convolutional neural network (CNN) based Resnet50 and 1D-CNN (1-Dimensional CNN) have been utilized to extract features from facial video clips and EEG modalities, respectively. A DCCA-based approach was used to fuse highly correlated features, and three basic human emotion categories (happy, neutral, and sad) were classified using the SoftMax classifier. The proposed approach was investigated based on the publicly available datasets called MAHNOB-HCI and DEAP. Experimental results revealed an average accuracy of 93.86% and 91.54% on the MAHNOB-HCI and DEAP datasets, respectively. The competitiveness of the proposed framework and the justification for exclusivity in achieving this accuracy were evaluated by comparison with existing work.
更多查看译文
关键词
CNN,Deep CCA,HCI,bimodal,electroencephalography,emotion recognition,facial video clips,feature level fusion
AI 理解论文
溯源树
样例
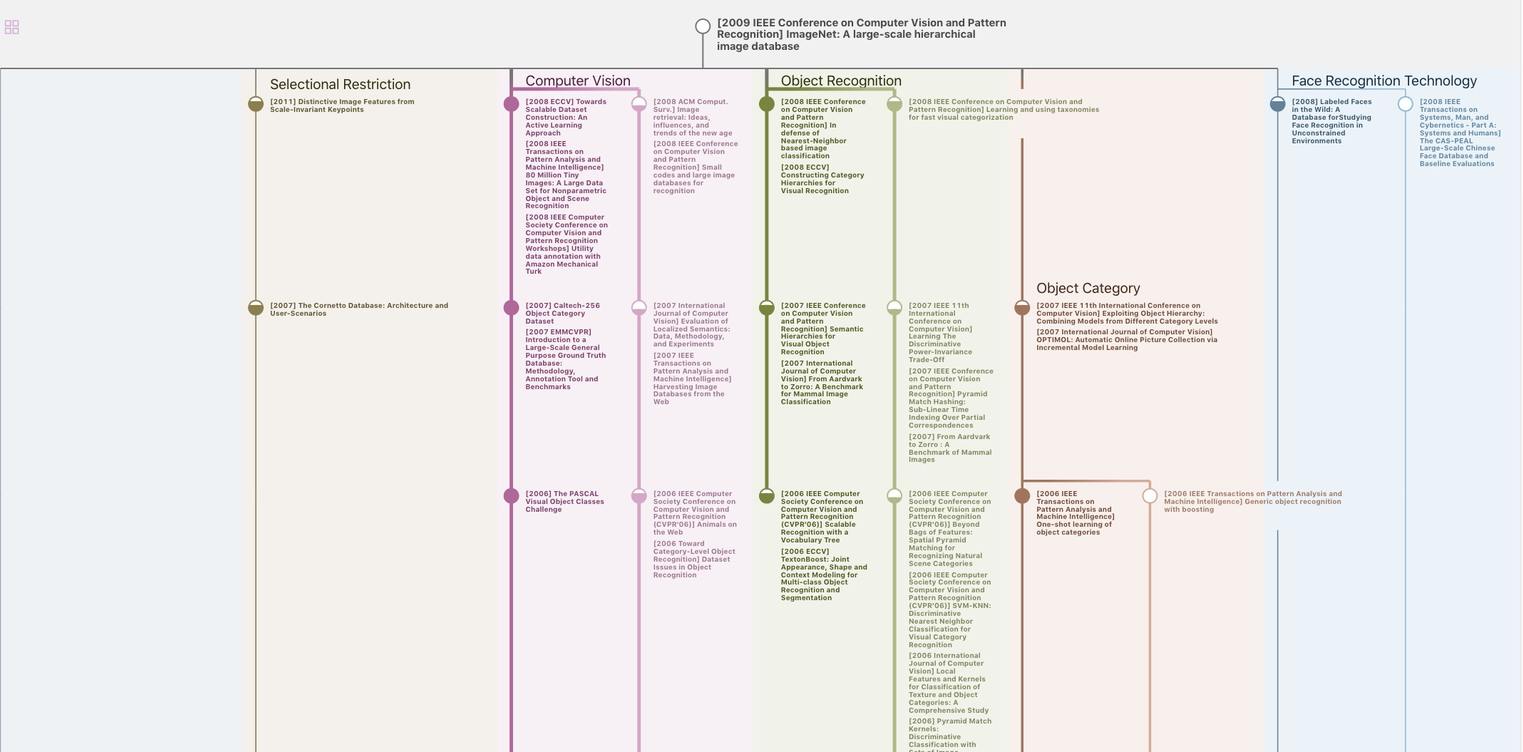
生成溯源树,研究论文发展脉络
Chat Paper
正在生成论文摘要