Fairness-aware genetic-algorithm-based few-shot classification.
Mathematical biosciences and engineering : MBE(2023)
摘要
Artificial-intelligence-assisted decision-making is appearing increasingly more frequently in our daily lives; however, it has been shown that biased data can cause unfairness in decision-making. In light of this, computational techniques are needed to limit the inequities in algorithmic decision-making. In this letter, we present a framework to join fair feature selection and fair meta-learning to do few-shot classification, which contains three parts: (1) a pre-processing component acts as an intermediate bridge between fair genetic algorithm (FairGA) and fair few-shot (FairFS) to generate the feature pool; (2) the FairGA module considers the presence or absence of words as gene expression, and filters out key features by a fairness clustering genetic algorithm; (3) the FairFS part carries out the task of representation and fairness constraint classification. Meanwhile, we propose a combinatorial loss function to cope with fairness constraints and hard samples. Experiments show that the proposed method achieves strong competitive performance on three public benchmarks.
更多查看译文
关键词
fairness , feature selection , few-shot , genetic algorithm , meta-learning
AI 理解论文
溯源树
样例
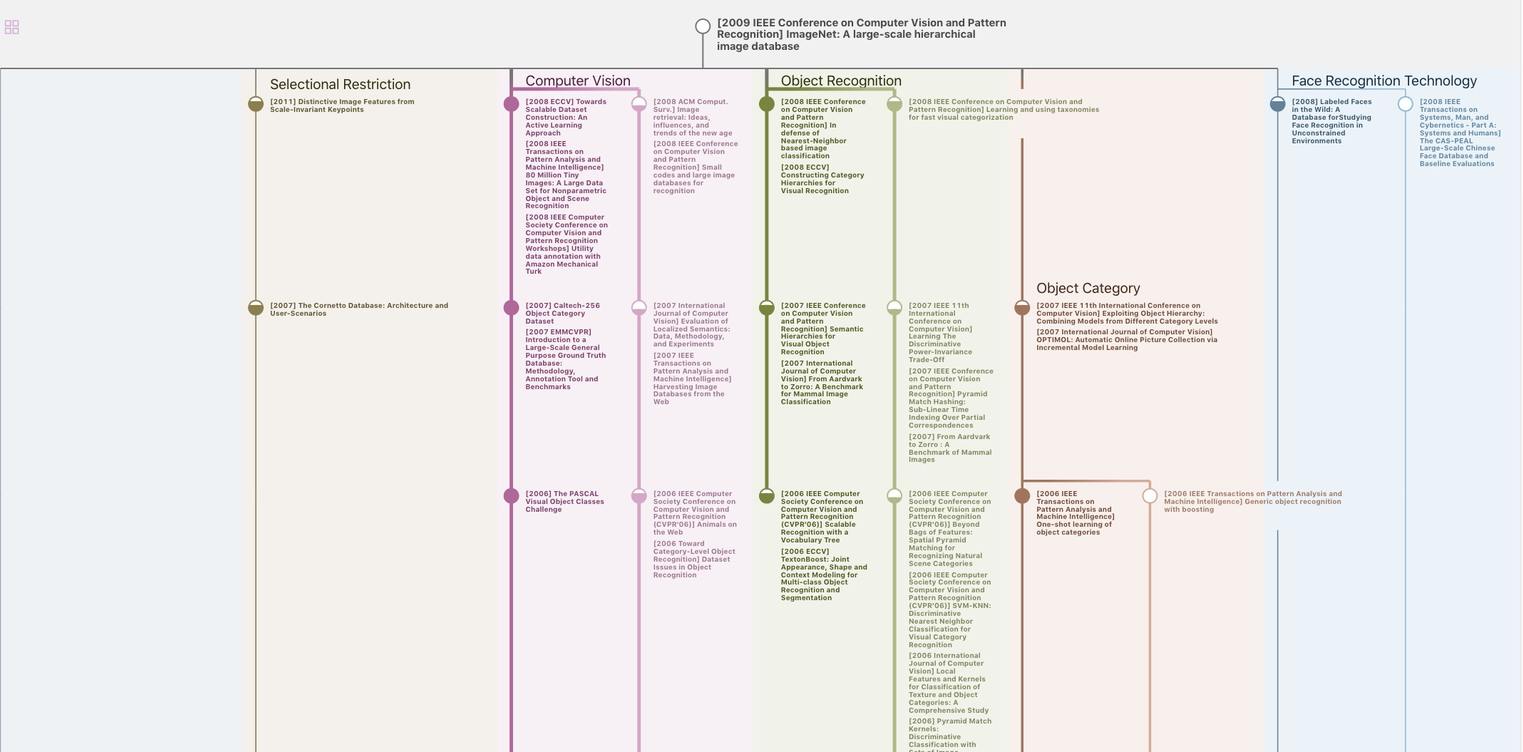
生成溯源树,研究论文发展脉络
Chat Paper
正在生成论文摘要