Memory Conscious Machine Learning Method to Extract Time-of-Flight Data From Flash LiDARs
IEEE Sensors Letters(2023)
摘要
This letter presents a memory-conscious architecture for single-photon-avalanche-diode (SPAD) light detection and ranging (LiDAR) systems to extract time-of-flight (ToF) information by learning the distribution of incoming photons' ToF to reduce the I/O bandwidth required by flash LiDAR digitizers. Such LiDAR systems can be sensitive to single photons, which makes them an excellent choice for both short- and long-range applications. However, counting and processing every photon means that the sensor front-end generates a massive amount of data to be processed in each frame. The proposed architecture will tackle this challenge by learning the incoming photons' distribution on-chip using mini-batches of time-stamped data from time-to-digital converters. Hence, only learned parameters need to be transferred off-chip instead of the raw data to re-create the histogram. We demonstrate the feasibility of this model in a Monte Carlo simulation.
更多查看译文
关键词
Sensor systems,sensor applications,flash light detection and ranging (LiDAR),machine learning,single-photon avalanche photodiode (SPAD),time-of-flight (ToF)
AI 理解论文
溯源树
样例
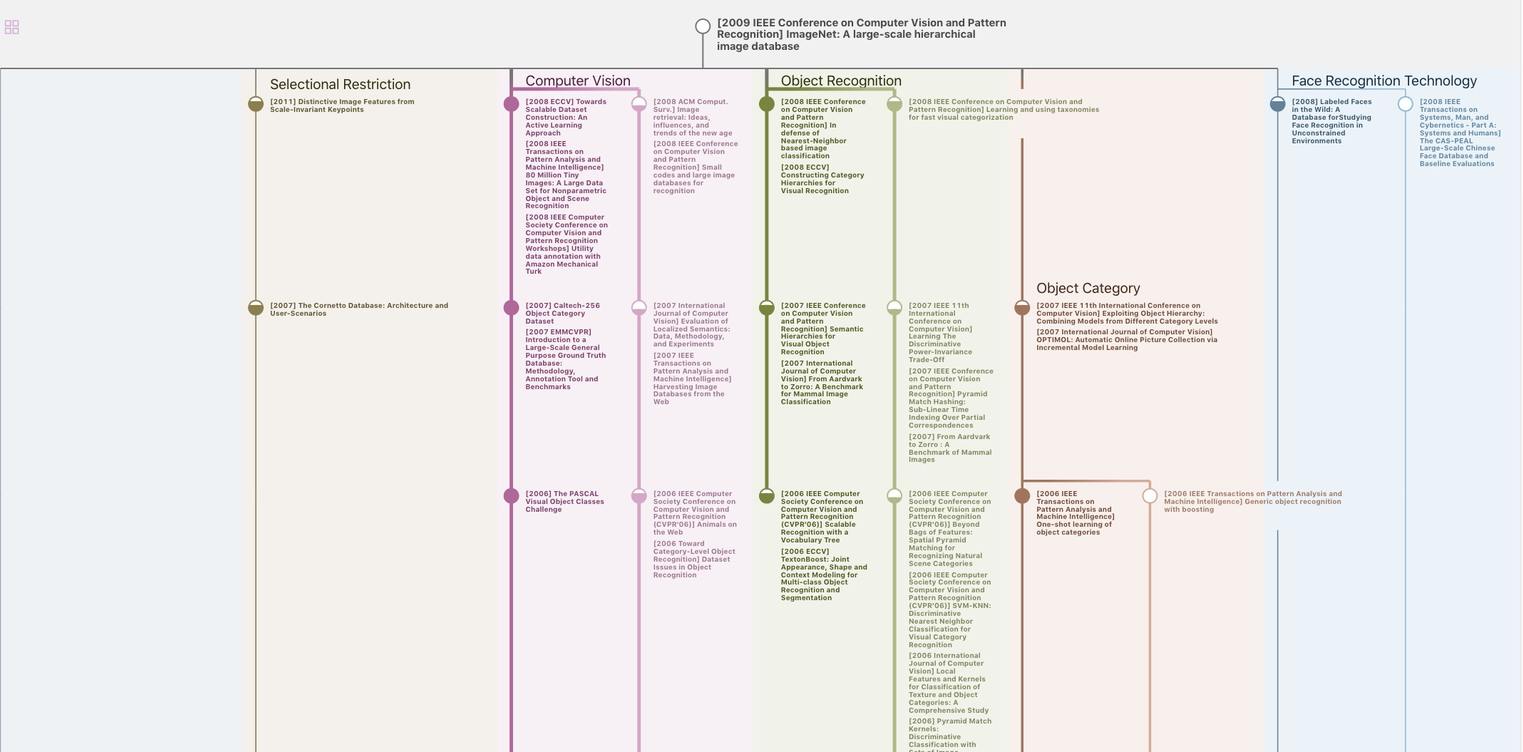
生成溯源树,研究论文发展脉络
Chat Paper
正在生成论文摘要