SCPNet: Self-constrained parallelism network for keypoint-based lightweight object detection
Journal of Visual Communication and Image Representation(2023)
摘要
Keypoint-based object detection achieves better performance without positioning calculations and extensive prediction. However, they have heavy backbone, and high-resolution is restored using upsampling that obtain unreliable features. We propose a self-constrained parallelism keypoint-based lightweight object detection network (SCPNet), which speeds inference, drops parameters, widens receptive fields, and makes prediction accurate. Specifically, the parallel multi-scale fusion module (PMFM) with parallel shuffle blocks (PSB) adopts parallel structure to obtain reliable features and reduce depth, adopts repeated multi-scale fusion to avoid too many parallel branches. The self-constrained detection module (SCDM) has a two-branch structure, with one branch predicting corners, and employing entad offset to match high-quality corner pairs, and the other branch predicting center keypoints. The distances between the paired corners’ geometric centers and the center keypoints are used for self-constrained detection. On MS-COCO 2017 and PASCAL VOC, SCPNet’s results are competitive with the state-of-the-art lightweight object detection. https://github.com/mengdie-wang/SCPNet.git.
更多查看译文
关键词
Keypoint-based lightweight object detection,Parallel multi-scale fusion,Parallel shuffle block,Self-constrained detection
AI 理解论文
溯源树
样例
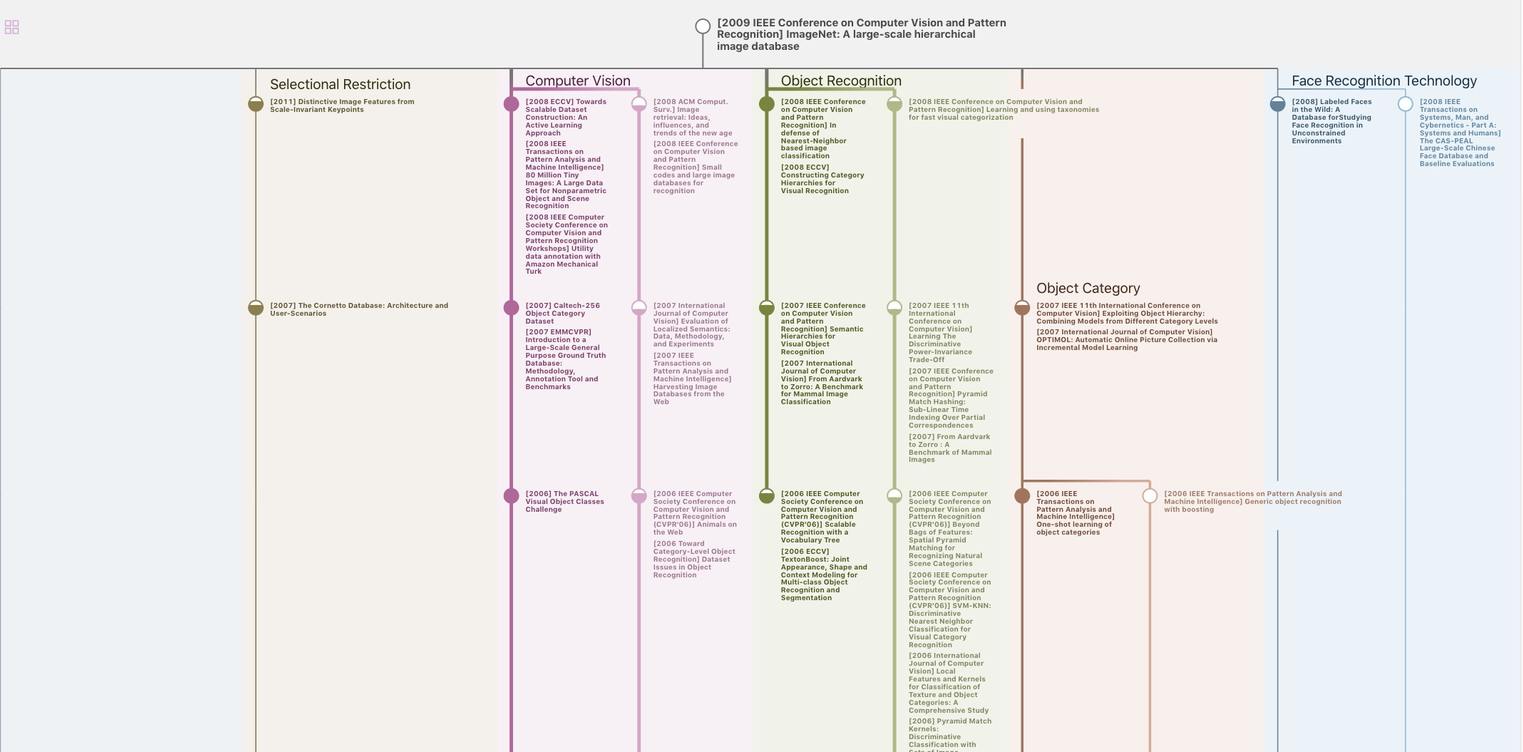
生成溯源树,研究论文发展脉络
Chat Paper
正在生成论文摘要