Anomaly detection framework for unmanned vending machines
Knowledge-Based Systems(2023)
摘要
Unmanned vending machines (UVMs) based on computer vision have shown huge commercial potential in unmanned retail. Anomaly detection is the problem of recognizing the falling-over and occlusion anomalies in UVMs. However, considering various anomaly features with different feature complexities, the existing methods are not sufficiently effective. In this paper, we propose an unmanned retail anomaly detection method based on deep convolutional neural networks (CNNs) called the complexity-classification anomaly detection (ClassAD) framework. The ClassAD method consists of two modules: a complexity rating module and a classification module. These two modules jointly input images of different feature complexity into different-capacity networks, which makes full use of the feature extraction ability of CNNs. ClassAD includes a decomposition feature enhancement approach to enhance inference speed. Furthermore, we introduce complexity-class loss and multi-instance loss in ClassAD for accuracy and learning stability. Experiments show that ClassAD yields promising results compared with state-of-the-art methods in both effectiveness and efficiency. In addition, we propose a UVM-Anomaly dataset for anomaly detection in UVMs.
更多查看译文
关键词
Unmanned retail,Anomaly detection,Classification,Loss function,Dataset
AI 理解论文
溯源树
样例
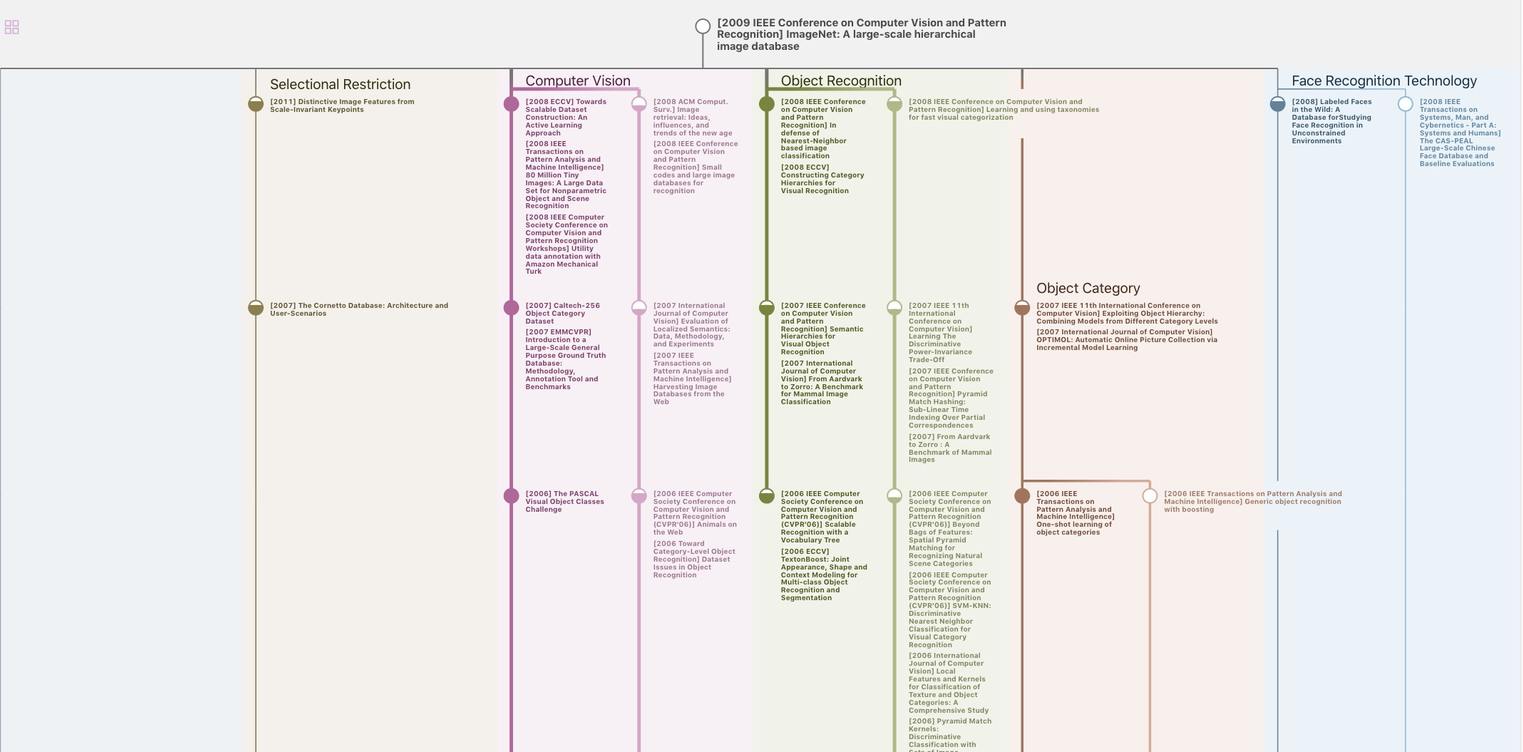
生成溯源树,研究论文发展脉络
Chat Paper
正在生成论文摘要