ConvBNet: A Convolutional Network for Building Footprint Extraction
IEEE Geoscience and Remote Sensing Letters(2023)
摘要
Building footprint is a key indicator of urban structures and economic development. Automatic extraction of building footprint from very-high-resolution (VHR) remote sensing imagery, which is of great practical interest for various geospatial-related applications, is still a challenging task for complex textures, varying scales and shapes, and other confusing artificial objects. To alleviate these problems and improve the extraction accuracy, this study proposed a novel pure convolutional neural network called ConvBNet, which integrates ConvNeXt-XL with a fusing decoder and adopts deep supervision in the training stage for the middle stage. Two-stage training strategy, using weighted cross-entropy (CE) loss and mask CE loss, respectively, ensures the stable convergence and makes the network focus on the boundary region. The proposed model was tested on the Wuhan University (WHU) building dataset (publicly available) and one private Zhejiang building dataset. Compared with other state-of-the-art (SOTA) methods, ConvBNet achieved the best intersection over Union (IoU), 91.22% and 77.90% for the two datasets, which proves its good performance in the task of extracting buildings from VHR images.
更多查看译文
关键词
Building extraction,convolutional neural network,deep learning,remote sensing
AI 理解论文
溯源树
样例
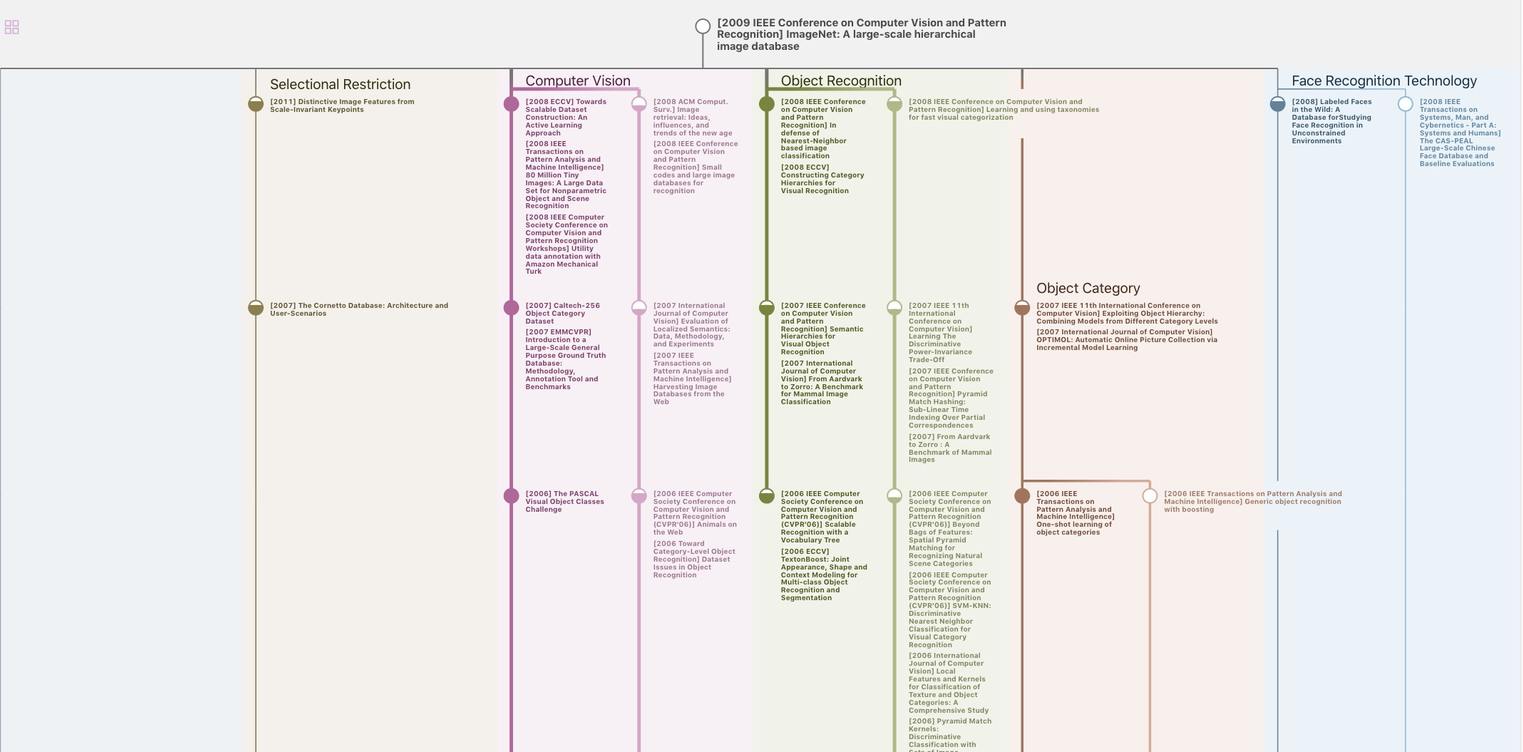
生成溯源树,研究论文发展脉络
Chat Paper
正在生成论文摘要