High-entropy alloy catalysts: Fundamental aspects, promises towards electrochemical NH3 production, and lessons to learn from deep neural networks
Nano Energy(2023)
摘要
A computational approach to judiciously predict high-entropy alloys (HEAs) as an efficient and sustainable material class for the electrochemical reduction of nitrogen is here presented. The approach employs density functional theory (DFT), adsorption energies of N atoms and N2 molecules as descriptors of the catalytic activity and deep neural networks. A probabilistic approach to quantifying the activity of HEA catalysts for nitrogen reduction reaction (NRR) is described, where catalyst elements and concentration are optimized to increase the probability of specific atomic arrangements on the surfaces. The approach provides key features for the effective filtering of HEA candidates without the need for time-consuming calculations. The relationships between activity and selectivity, which correlate with the averaged valence electron concentration and averaged electronegativity of the reference HEA catalyst, are analyzed in terms of sufficient interaction for sustained reactions and, at the same time, for the release of the active site. As a result, a complete list of 3000 HEAs consisting of quinary components of the elements Mo, Cr, Mn, Fe, Co, Ni, Cu, and Zn are reported together with their metrics to rank them from the most likely to the least likely active catalysts for NRR in gas diffusion electrodes, or for the case where non-aqueous electrolytes are utilized to suppress the competing hydrogen evolution reaction. Moreover, the energetic landscape of the electrochemical NRR transformations are computed and compared to the case of Fe. The study also analyses and discusses how the results would translate to liquid-solid reactions in aqueous electrochemical cells, further affected by changes in properties upon hydroxylation, oxygen, hydrogen, and water coverages.
更多查看译文
关键词
High-entropy alloys,Electrocatalytic nitrogen reduction,Scaling-relations,Machine learning,Deep neural networks
AI 理解论文
溯源树
样例
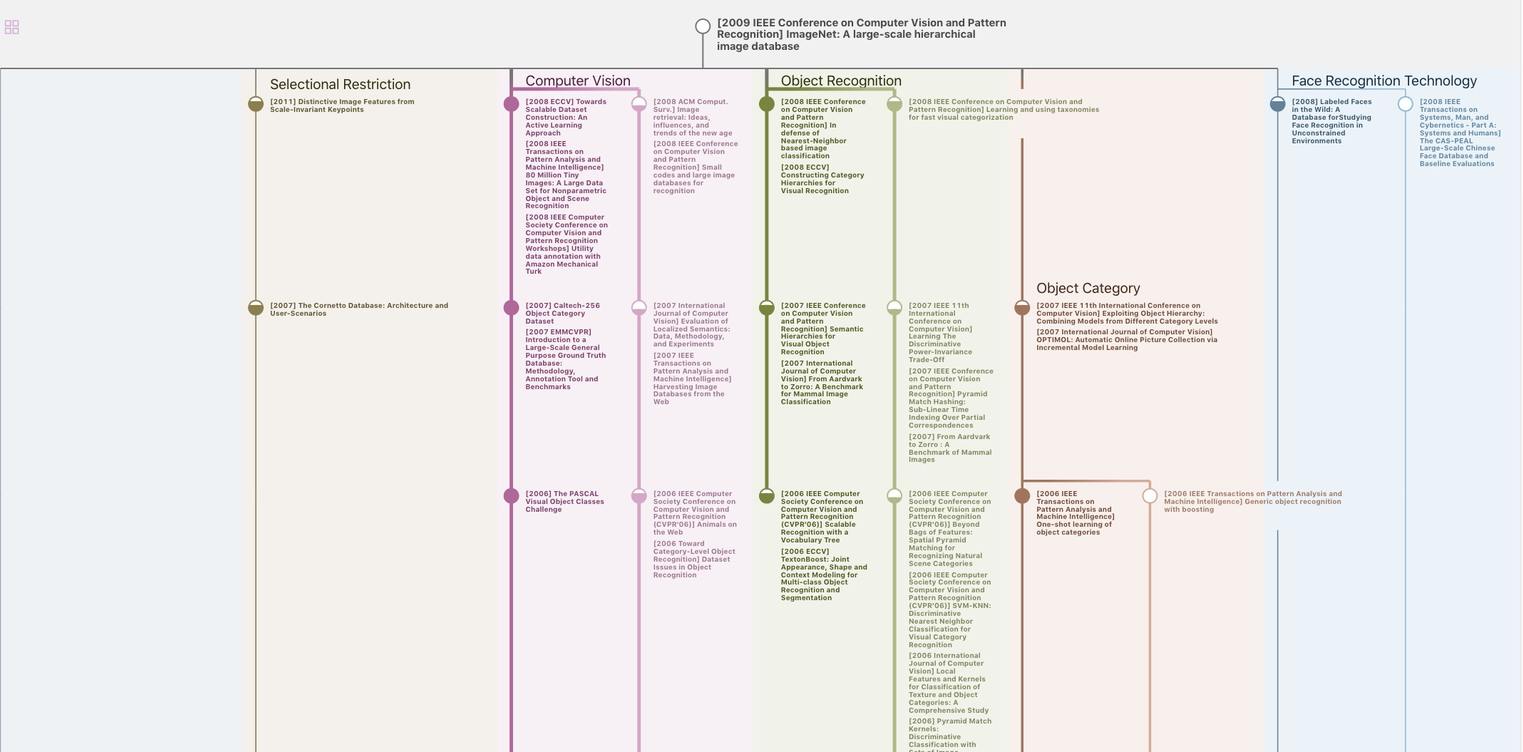
生成溯源树,研究论文发展脉络
Chat Paper
正在生成论文摘要