On better detecting and leveraging noisy samples for learning with severe label noise
Pattern Recognition(2023)
摘要
Despite the success of learning with noisy labels, existing approaches show limited performance when the noise level is extremely high, since deep neural networks (DNNs) are easily overfit to the training set with corrupted labels. In this paper, we introduce Lipschitz regularization to prevent the DNNs from over-fitting to noisy labels quickly. Meanwhile, to better detect and leverage the noisy samples, we pro -pose a Lipschitz regularization based framework with a combination of adaptive modeling and detection module and improved semi-supervised learning. We propose to adaptively model the real distribution of the training set, and the implicit individual clean/noisy distribution, instead of parametric models. With Bayes' rule, we then compute the posterior probability of a sample being clean, which provides a dynamic threshold for the detection of noisy labels. To reduce training instability caused by less labeled data with severe label noise, we improve the semi-supervised learning by combining the advantages of Mixup and FixMatch. It can not only increase the diversity of unlabeled samples, but also improve the generaliza-tion capability of the DNNs to avoid over-fitting. Experiments on several benchmarks demonstrate that our approach achieves comparable results with the state-of-the-art methods in the less-noisy environ-ment, and obtains a substantial improvement ( - 8% and -6% in accuracy on CIFAR-10 and CIFAR-10 0 respectively) with severe noise.(c) 2022 Elsevier Ltd. All rights reserved.
更多查看译文
关键词
Severe label noise,Lipschitz regularization,Adaptive modeling and detection of label,noise,Semi-supervised learning
AI 理解论文
溯源树
样例
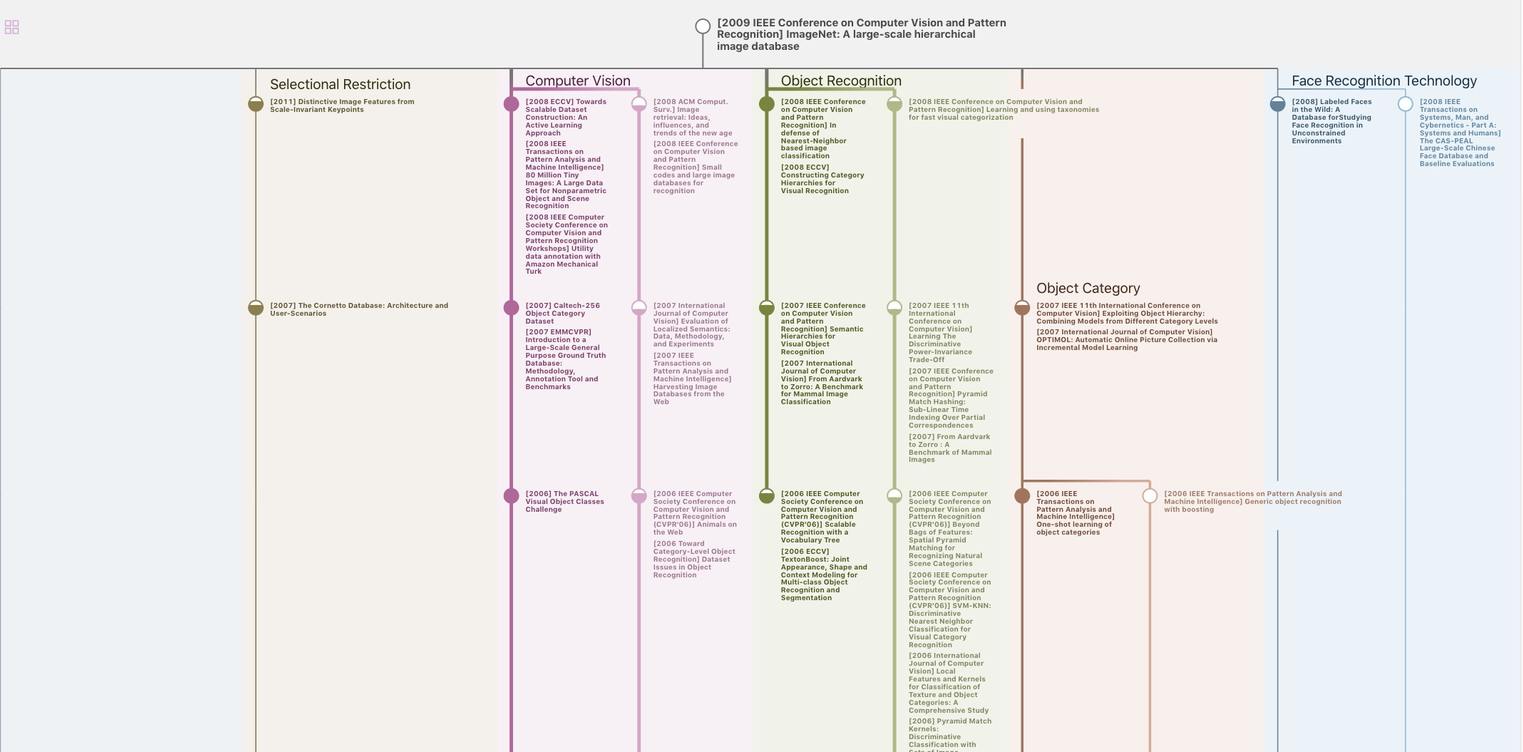
生成溯源树,研究论文发展脉络
Chat Paper
正在生成论文摘要