Beware of Instantaneous Dependence in Reinforcement Learning
arxiv(2023)
摘要
Playing an important role in Model-Based Reinforcement Learning (MBRL), environment models aim to predict future states based on the past. Existing works usually ignore instantaneous dependence in the state, that is, assuming that the future state variables are conditionally independent given the past states. However, instantaneous dependence is prevalent in many RL environments. For instance, in the stock market, instantaneous dependence can exist between two stocks because the fluctuation of one stock can quickly affect the other and the resolution of price change is lower than that of the effect. In this paper, we prove that with few exceptions, ignoring instantaneous dependence can result in suboptimal policy learning in MBRL. To address the suboptimality problem, we propose a simple plug-and-play method to enable existing MBRL algorithms to take instantaneous dependence into account. Through experiments on two benchmarks, we (1) confirm the existence of instantaneous dependence with visualization; (2) validate our theoretical findings that ignoring instantaneous dependence leads to suboptimal policy; (3) verify that our method effectively enables reinforcement learning with instantaneous dependence and improves policy performance.
更多查看译文
关键词
instantaneous dependence,learning
AI 理解论文
溯源树
样例
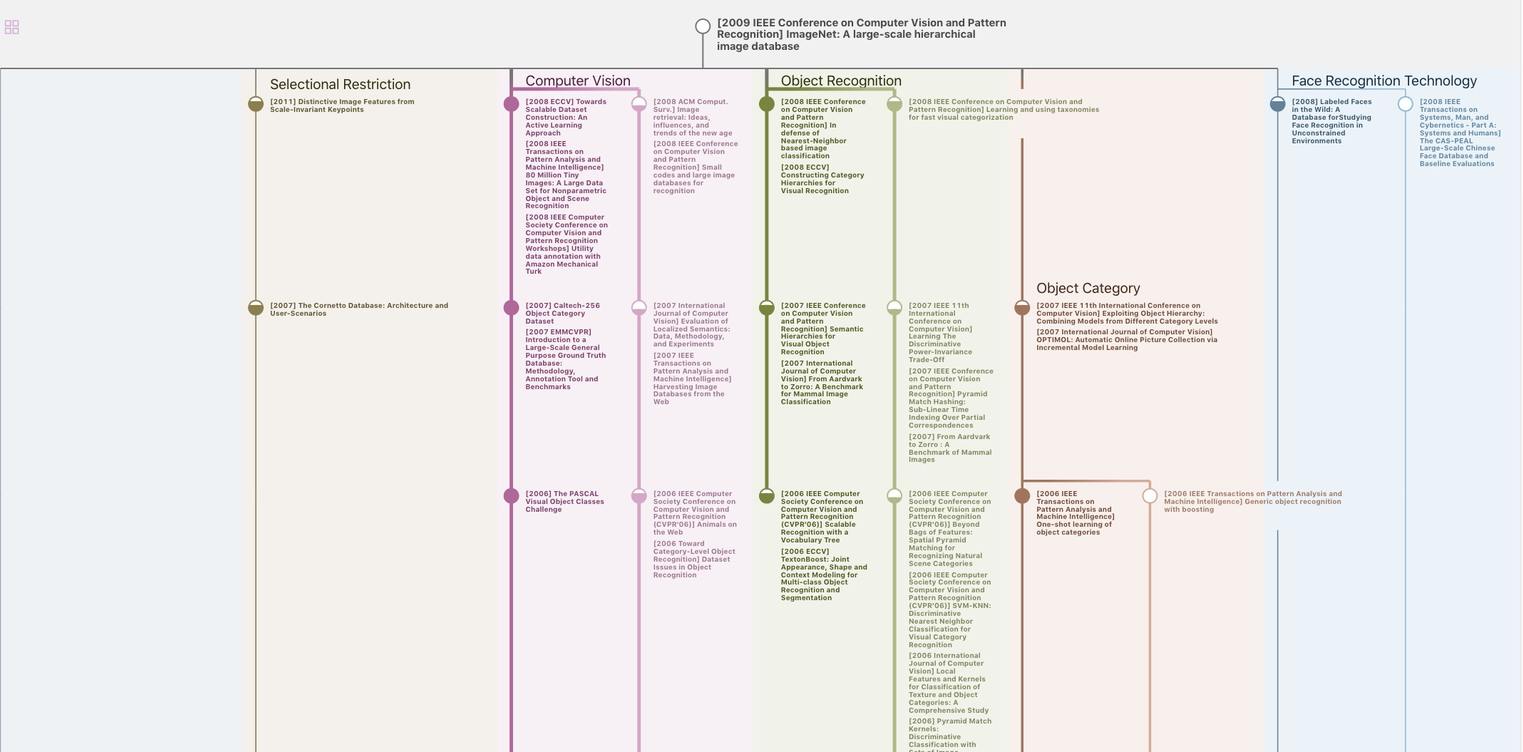
生成溯源树,研究论文发展脉络
Chat Paper
正在生成论文摘要