Dynamic Stashing Quantization for Efficient Transformer Training.
arxiv(2023)
摘要
Large Language Models (LLMs) have demonstrated impressive performance on a range of Natural Language Processing (NLP) tasks. Unfortunately, the immense amount of computations and memory accesses required for LLM training makes them prohibitively expensive in terms of hardware cost, and thus challenging to deploy in use cases such as on-device learning. In this paper, motivated by the observation that LLM training is memory-bound, we propose a novel dynamic quantization strategy, termed Dynamic Stashing Quantization (DSQ), that puts a special focus on reducing the memory operations, but also enjoys the other benefits of low precision training, such as the reduced arithmetic cost. We conduct a thorough study on two translation tasks (trained-from-scratch) and three classification tasks (fine-tuning). DSQ reduces the amount of arithmetic operations by $20.95\times$ and the number of DRAM operations by $2.55\times$ on IWSLT17 compared to the standard 16-bit fixed-point, which is widely used in on-device learning.
更多查看译文
关键词
dynamic stashing quantization,training
AI 理解论文
溯源树
样例
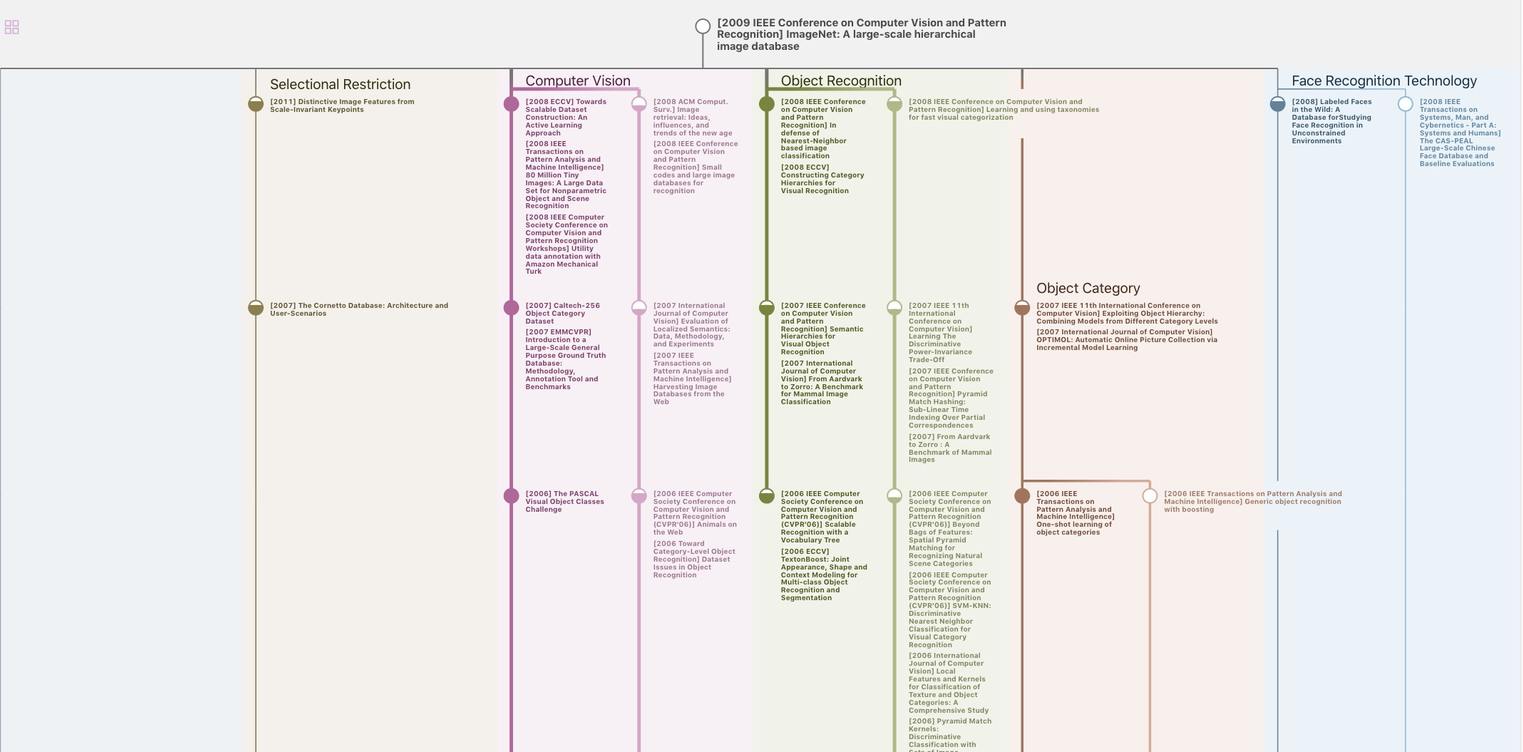
生成溯源树,研究论文发展脉络
Chat Paper
正在生成论文摘要