Structural Similarity: When to Use Deep Generative Models on Imbalanced Image Dataset Augmentation
arxiv(2023)
摘要
Improving the performance on an imbalanced training set is one of the main challenges in nowadays Machine Learning. One way to augment and thus re-balance the image dataset is through existing deep generative models, like class-conditional Generative Adversarial Networks (cGAN) or Diffusion Models by synthesizing images on each of the tail-class. Our experiments on imbalanced image dataset classification show that, the validation accuracy improvement with such re-balancing method is related to the image similarity between different classes. Thus, to quantify this image dataset class similarity, we propose a measurement called Super-Sub Class Structural Similarity (SSIM-supSubCls) based on Structural Similarity (SSIM). A deep generative model data augmentation classification (GM-augCls) pipeline is also provided to verify this metric correlates with the accuracy enhancement. We further quantify the relationship between them, discovering that the accuracy improvement decays exponentially with respect to SSIM-supSubCls values.
更多查看译文
关键词
deep generative models,imbalanced
AI 理解论文
溯源树
样例
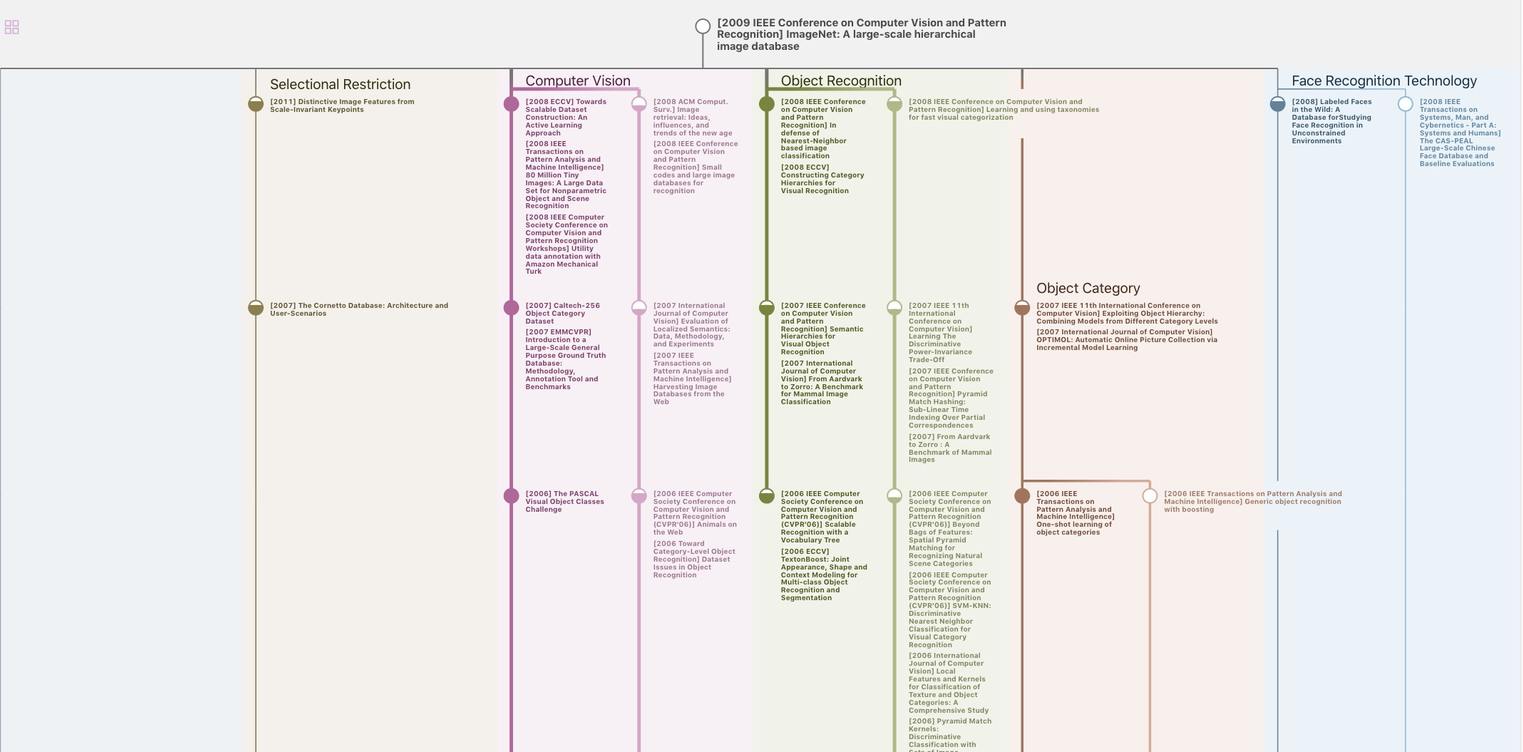
生成溯源树,研究论文发展脉络
Chat Paper
正在生成论文摘要